Gartner predicts that 85 percent of AI projects will fail to perform as expected through 2022. Which means that out of every 20 projects released in the next two years, only 3 will be successful– and the remaining 17 will fail. Why? Because creating a machine learning model and operating it in an enterprise environment are two very different things. The biggest challenge for companies implementing AI is operationalizing machine learning in the real world, which is why MLOps is growing so rapidly.
Feature Stores are a new MLOps technology being adopted by cutting-edge companies like Uber, Airbnb, and Netflix, and for good reason. A Feature Store is a system made specifically to automate the input, tracking, and governance of data into machine learning models. Feature Stores compute and store features, enabling them to be registered, discovered, used, and shared across a company. Providing a centralized and reproducible framework to manage the data feeding machine learning models has a variety of benefits for Enterprise AI.
Improve Data Science Productivity
Data scientists are few and far between, and they don’t come cheap. Improving data science productivity by eliminating repetitive and unnecessary work means that you can produce more models in less time with your current staff.
In a typical data science silo, data scientists spend 80% of their time on data preparation,and only the remaining 20% is actually spent on deploying the machine learning model. Data prep work is manual, monotonous, and tedious: 76% of data scientists rated data prep as the least enjoyable part of their work. On top of that, many data scientists throughout a company end up slogging through the data to calculate the same features that another data scientist in the company has already created.
With a Feature Store, a data scientist can immediately start on a new problem by exploring the features that are already available. In many cases, someone in the past will have already created the relevant features, so the data scientist can easily produce a training set and start building models right away.
If the features they need aren’t there yet, they can always create their own features or collaborate with data engineers which will strengthen the Feature Store for the others in the future.
Enable Pipeline Integrity
Alongside the time and energy drain of unnecessary work, lacking a consistent way to calculate features can lead to models that vary wildly between data silos.
For example, in a retail company, one team may calculate “total customer revenue” by subtracting returns from sales, where another team calculates it just using sales. Both are valid metrics, but if they are both called “total customer revenue”, the result is inconsistently calculated metrics in different data pipelines.
A Feature Store addresses this by adding traceability, visibility, and versioning into the data pipelines that feed features. In addition, naming constraints are built into feature stores that stop one team from overwriting the work of another; the second team must name their calculation something new to distinguish their work.
But Feature Stores go beyond making the lives of data scientists easier; they also allow for better predictions from machine learning models.
Enhance Data Freshness
If your machine learning model is trained on data that is inaccurate or outdated, your model is going to make mistakes that could cost you. Having the most recent data is absolutely essential in a business environment. If a customer bought a product from an ad they saw yesterday, but the advertising data doesn’t update until tomorrow, they could be shown a product today that they already own. Anyone who has been in this position knows how annoying it is to be shown– and if it continues to happen, they might be discouraged from supporting that company in the future.
With a Feature Store managing your data pipelines, you and your team are assured that the newest data is always retrieved. The pipeline is scheduled to run with the cadence of the data; monthly features are calculated monthly, daily metrics are calculated once a day, and real-time features are updated instantly, so your predictions are always based on the newest data.
Facilitate Time Consistency
Timing is everything for machine learning models. Human brains make decisions based on what we know in the moment and what we’ve learned from the past; we cannot make decisions based on information from the future. Machine learning models learn the same way.
When creating training data, it is extremely important to take this into account. The set of features used for training must be the values that were known at the time of the event.
A Feature Store solves this problem by producing training data sets with time-consistent feature values taken from each Feature Set’s history at the point in time of the events being modeled.
By keeping the historical values of all features, a Feature Store allows you to create accurate training sets, which in turn translate to accurate predictions.
Provide Model Explainability
One of the most powerful benefits of having time-consistent data is that it enables trust when checking machine learning models.
Let’s say you run a bank, and a bank regulator comes to audit your software’s performance. The regulator wants to check that your model’s process for granting a customer’s loan request is unbiased. If you have a feature store with time-consistent data and transparent data lineage, it’s really easy for the regulator to check the underwriting process, and ensure that there is no discrimination innate in the data or software.
An even more powerful combination is linking your Feature Store with your machine learning workflow system. This strong link allows you to create a repository of all of the activities and notebook artifacts that went into training a model. You can examine the lineage of the model in question all the way back to the data that trained that model. Being able to analyze this data is crucial to ensure that your model is not built on biased data, so you can show your regulator why your model came to the conclusion it did.
Conclusion
So, why do you need a feature store? Not only does it save data scientists time and energy, it allows machine learning models to make more accurate predictions that can increase a company’s revenue. On top of that, automating key parts of the machine learning pipeline allows models to be created more quickly and at a lower price, allowing you to scale enterprise AI 100x faster. Finally, keeping all of these steps clearly visible and open to scrutiny makes it easy to ensure regulatory compliance, which builds trust in your customers and critics alike.
About the Author
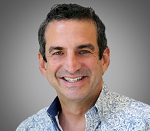
Monte Zweben is the CEO and co-founder of Splice Machine. A technology industry veteran, Monte’s early career was spent with the NASA Ames Research Center as the deputy chief of the artificial intelligence branch, where he won the prestigious Space Act Award for his work on the Space Shuttle program. Monte then founded and was the chairman and CEO of Red Pepper Software, a leading supply chain optimization company, which later merged with PeopleSoft, where he was VP and general manager, Manufacturing Business Unit. Then, Monte was the founder and CEO of Blue Martini Software – the leader in e-commerce and omni-channel marketing. He was Chairman of Rocket Fuel Inc. and serves on the Dean’s Advisory Board for Carnegie Mellon University’s School of Computer Science.
Sign up for the free insideAI News newsletter.
Join us on Twitter: @InsideBigData1 – https://twitter.com/InsideBigData1
Speak Your Mind