This guest article from Matt Miller, Director of Product Marketing for WekaIO, highlights why focusing on optimizing infrastructure can spur machine learning workloads and AI success.
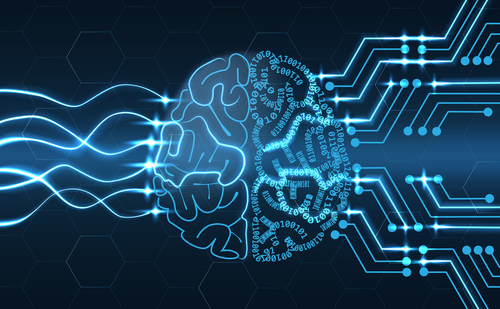
Recent years have seen a boom in the generation of data from a variety of sources: connected devices, IoT, analytics, healthcare, smartphones, and much more. In fact, as of 2016, 90% of all data ever created had been created in the previous two years. Gaining insights from all of this data presents a tremendous opportunity for organizations to further their businesses, expand more quickly into new markets, to advance research in healthcare or climate — just to name a few. However, the urgency of managing the sheer amount of data, coupled with the need to more and more quickly glean insights from it, is palpable. According to Gartner, organizations have been reporting unstructured data growth of over 50% year over year, while at the same time an Accenture survey found that 79% of enterprise executives agree that not extracting value and insight from this data will lead to extinction for their businesses. This data management problem is particularly acute in the areas of artificial intelligence (AI) and machine learning workloads where there are both extreme compute requirements and the need to store massive amounts of data that will be analyzed in some form.
Legacy storage can’t keep up
The growth of data puts IT organizations under tremendous stress to stay responsive to their businesses. In order to deal with this growth in unstructured data, many organizations have turned to scale-out storage systems, where capacity can be expanded by adding new connected devices, but yet has not addressed the performance challenge at hand to deal with data growth and access of that data quickly. Gartner also states that by 2022 more than 80% of enterprise data will be stored in scale-out storage systems in enterprise and cloud data centers, up from 40% in 2018. However, legacy storage was designed to solve yesterday’s problems and cannot handle the unforeseen problems now being encountered with new workloads. They can neither feed data into compute resources fast enough, resulting in wasted compute time for expensive CPUs or GPUs, nor can they scale to petabytes of capacity when performance counts. Managing metadata also becomes an issue when dealing with literally billions of files within a data set.
This is often referred to as the enterprise “performance tax”. In order to keep up with the massive increase in performance and capacity requirements, organizations are having to invest heavily in yet more infrastructure – increasing capital expenditures as well as management complexity.
Storage and data architects are faced with three mains challenges in servicing these new requirements:
Driving data center agility
As mentioned, the combination of the growing size of data and the increasing urgency organizations are under to glean insight from it, creates challenges with storing, protecting, and processing this data. Legacy storage lacks the performance and management functionality to keep data available and shareable among key workloads. In these scenarios, data tends to be stored in islands, or silos, making it very onerous and time-consuming to take advantage of modern machine learning (ML) workflows.
Accelerate data transformation for AI and ML workloads
Modern analytics workloads – specifically ML and deep learning – have transformed how data needs to be used within organizations. These new workloads require extremely large data sets, faster and more parallel access to that data, and algorithms for training that pave the way for learning.
However, legacy storage solutions cannot take advantage of today’s high bandwidth networks, so organizations have turned to parallel file systems that offer a much higher performance profile and support for multiple types of data that can help streamline the process of preparing data for use in AI/ML workloads.
Optimize investments in infrastructure
Finally, regarding the “performance tax”, organizations want to optimize their investment in high value GPU and/or CPU infrastructures that typically are required in AI and ML workloads. Having these resources sit idle or underutilized carries a real bottom line impact if the underlying storage infrastructure cannot keep data fed into them.
New storage solutions should be able to remove this I/O bottleneck, take advantage of higher performance networking (such as 100GbE or InfiniBand), and keep those I/O-starved GPUs and CPUs ‘fed’. This will help organizations avoid huge new investments in infrastructure and lower their ‘taxes’.
WekaIO can help solve big problems
WekaIO has made its mark with a new approach to storage that helps solve these previously unforeseen problems with data- and performance-intensive workloads. The Weka file system is a software-based, scale-out storage solution optimized for high-performance workloads such as AI, ML, and analytics.
Legacy storage solutions cannot take advantage of today’s high bandwidth networks, so organizations have turned to parallel file systems that offer a much higher performance profile and support for multiple types of data that can help streamline the process of preparing data for use in AI/ML workloads.
Weka offers the simplicity of legacy storage, but it delivers higher performance than all-flash-storage, cloud scalability and simplified management never envisioned by other product vendors. In production environments, WekaIO has shown to deliver 10 times the performance of a traditional NAS system with linear scaling as the infrastructure grows.
How Weka Works
Weka has built a distributed, scalable, high-performance software platform that connects multiple servers with locally attached solid-state drives into a POSIX-compliant global namespace for performance and simplified management. The software is deployed on standard commercially available servers providing true hardware independence and best cost. The software supports internal tiering to any commercially available S3 object storage solution delivering massive scalability and great economics for an ever-growing data catalog. The below graph provides an architectural overview of a typical deployment for deep learning environments.
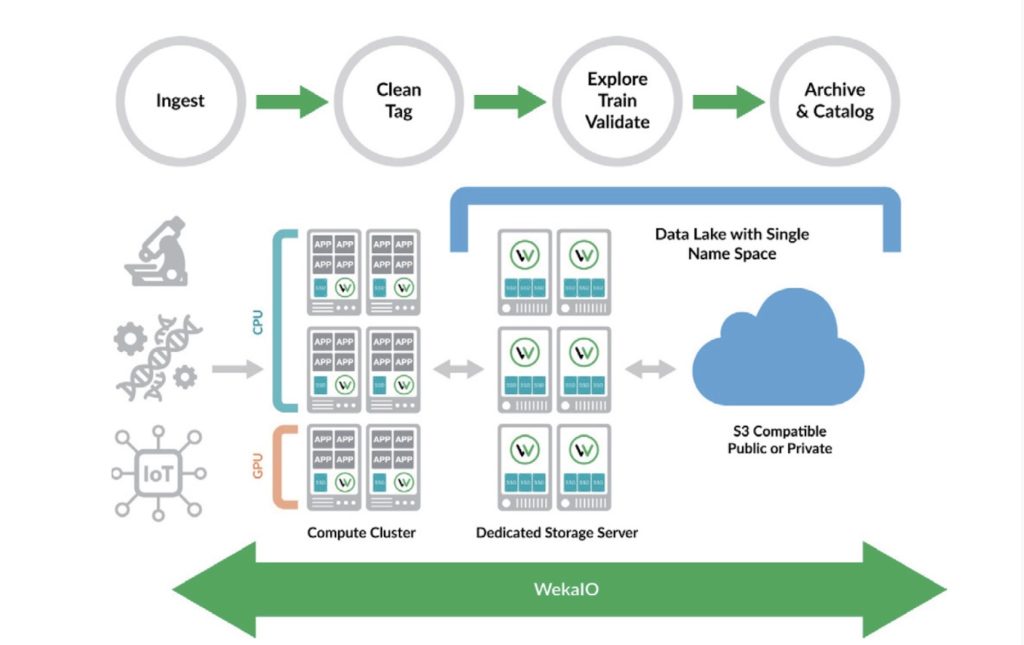
Today’s high-performance workloads demand a modern storage infrastructure, that delivers the performance, manageability, scalability and cost efficiency demanded in the era of data transformation—turning a company’s data into real value for the business. Legacy storage, such as NAS and parallel file systems designed in the disk era, simply won’t hit the mark.
For more information on how WekaIO can help modernize your infrastructure for AI/ML workloads, go to the Get Off Your NAS page to learn more.
Matt Miller is the Director of Product Marketing for WekaIO, responsible for marketing strategy and positioning. Matt has spent nearly 20 years in the storage industry in both product management and product marketing roles, for companies such as HPE, Nimble Storage, NetApp, Sun Mircosystems and Veritas.
Speak Your Mind