Many organizations follow an old trend to adopt AI and HPDA as distinct entities which leads to underutilization of their clusters. To avoid this, clusters can be converged to save (or potentially eliminate) capital expenditures and reduce OPEX costs. This sponsored post from Intel’s Esther Baldwin, AI Strategist, explores how organizations are using converged HPC clusters to combine HPC, AI, and HPDA workloads.
Forward looking organizations are expanding their definitions of high-performance computing (HPC) to include workloads such as artificial intelligence (AI) and high-performance data analytics (HPDA) in addition to traditional HPC simulation and modeling workloads. The challenge is to run each of these individually important workloads efficiently in the data center, without wasting resources and incurring significant CAPEX (capital) and OPEX (operating) expenditures or causing user pain. The solution is to use converged HPC clusters to run these disparate workloads in a unified data environment. Experience has shown that converged clusters can support modern HPC, AI and Analytic workloads with high runtime efficiency and resource utilization.[
The mass impact of AI and cloud computing has stimulated a remarkable maturation of industry-standard tools to support these workloads. While there is no single “one size fits all” solution for all workloads, it is possible to implement solutions that will lead to the vision of a unified environment out of disparate workload-optimized AI, HPC, and HPDA clusters.
Succinctly, it’s a very hard thing to converge these three workloads onto the same infrastructure.
- Software stack: The main challenge is that the underlying software stacks tend to optimize for different libraries, tools, and runtime behavior. Having these software stacks loaded at the right time on a cluster is difficult to do.
- Scheduling: HPC systems rely on giving blocks of time for users to run their work, if they use the whole time great, if not there is idle time and an underutilized resource. Alternatively, data analytics and AI work queue dynamically.
- Storage utilization: HPC workloads tend to rely on a high read and write rates, while data analytics and AI rely more on reading and processing.
- Overall: The skillset and ecosystems are also very different as one community prefers open source software and another commercially supported software.
Integrated Infrastructure Rather Than Piece-Meal
Unfortunately, many HPC organizations and businesses incorrectly adopt AI and HPDA as distinct entities separate from traditional modeling and simulation. This leads to siloed clusters that suffer from underutilization and requires time consuming data transfer. Instead, it is much better to converge these clusters to run as a unified environment as illustrated by the Unified Cluster Architecture panel in the figure below.
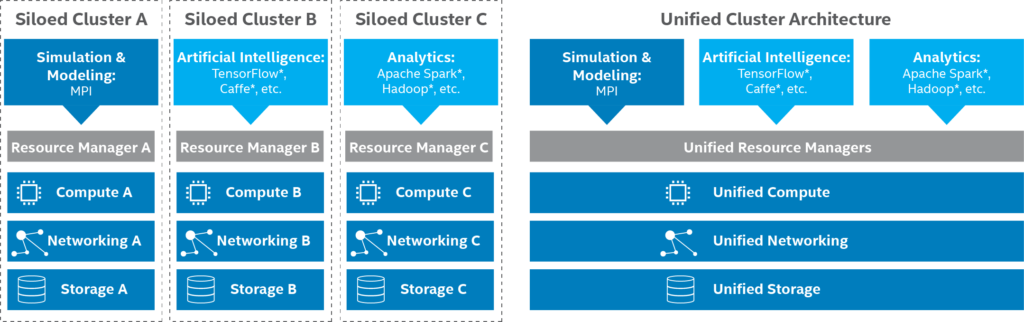
This is where the mass convergence of AI and HPDA with HPC is paying off for the consumer as vendors are now supporting workload optimized systems that can be pooled together by a software resource manager. The end result maximizes the value of existing resources because the resource manager, not humans, works 24/7 to keep the hardware busy. Meanwhile, a unified architecture of converged clusters also meets overall business needs as illustrated below:
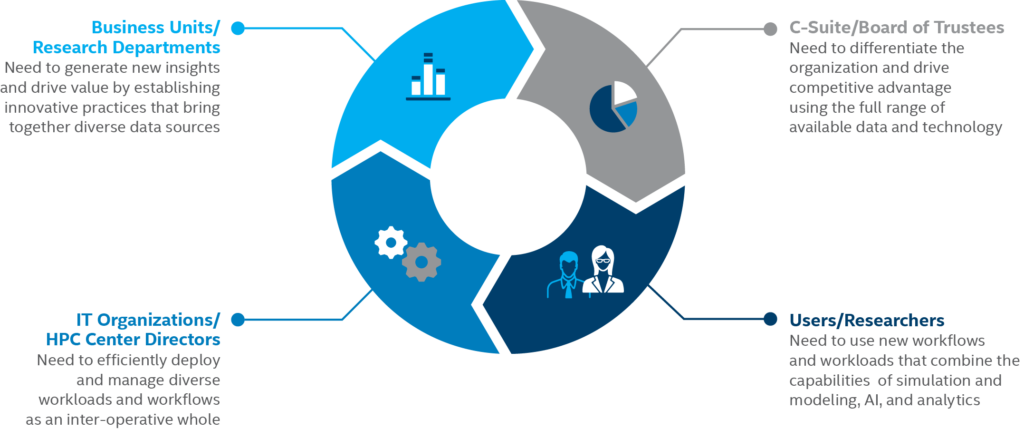
The value drivers are shown below.
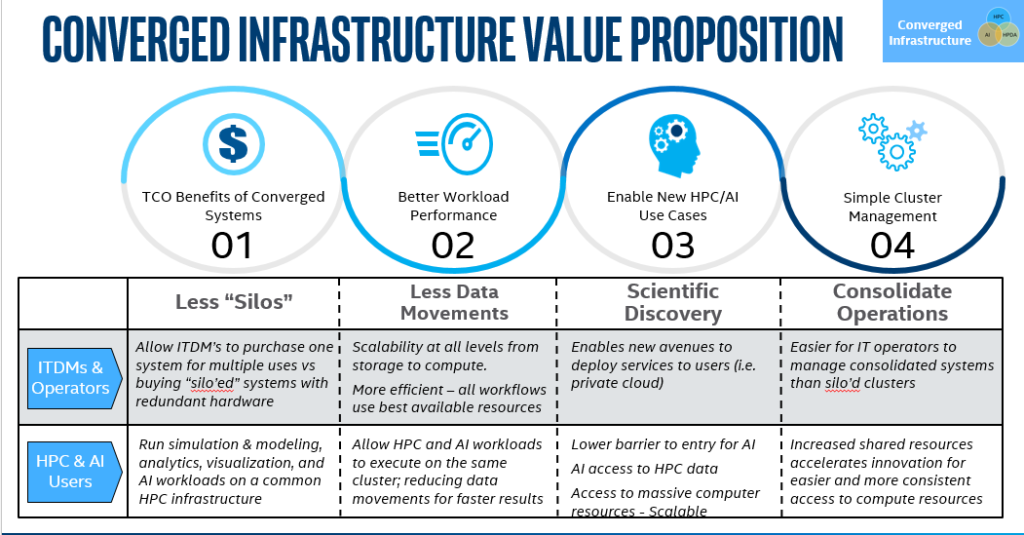
Resource management is core to efficiency
As discussed in the brief, Supporting Simulation and Modeling, AI, and Analytics on a Common Platform, a unified cluster architecture optimizes the ability to manage multiple workflows holistically. This boils down to providing a few essential changes to the software stack as shown below.
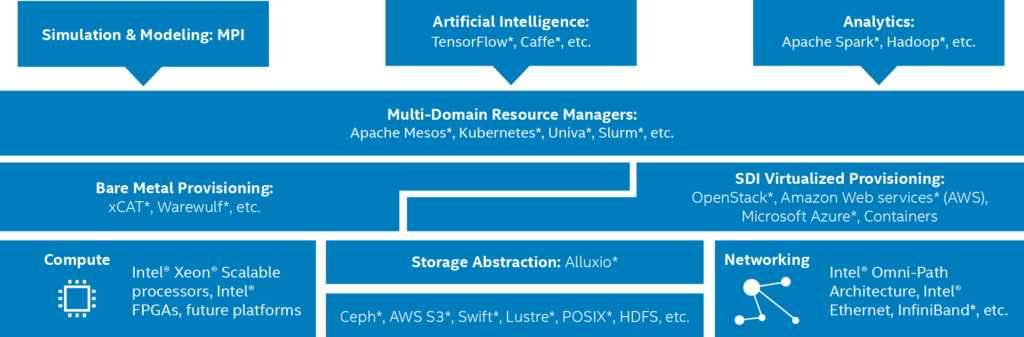
Key component: Unified job scheduling
The unified cluster manager is a necessary and critical component to unifying the data within the pooled clusters. In short, use a vendor-supported, industry resource manager to run jobs on all your systems – regardless if the jobs are managed on the local cluster by a traditional batch-oriented HPC scheduler or via a private cloud framework like Apache Mesos. To manage and optimize distributed applications, services, and big data frameworks, we focus on solutions such as the Univa Grid Engine or the open-source Magpie for SLURM.
Key Component: Reducing Brittleness and Data Movement via a Unifying Storage Abstraction
Without a unified view of the data, the system becomes brittle due to human error (such as incorrect path names in the scripts that access data) or spends inordinate amounts of time moving data between clusters. One solution that many vendors recommend to provide a unified storage abstraction is the open-source Alluxio storage abstraction software.
Look to the costs of running separate clusters to determine the direct value for your organization.
Experience has shown that running multiple, siloed clusters as shown in Figure 1, adds significantly to the capital expenditure (CAPEX) associated with purchasing and deploying the systems in the first place, as well as the operating expenditure (OPEX) to keep them running.[
For example, most organizations that need infrastructure for deep learning networks don’t run these workloads on a 24×7 basis. The part-time nature of these workloads means that the special-purpose infrastructure often stands idle and may require rarefied skills to support, both of which can be costly to the business. In many cases, the performance of modern Intel Xeon processors used in most servers, and especially those with built-in Intel Deep Learning Boost, eliminates the need for specialized accelerators for AI. Overall, basing your clusters on general-purpose servers it can the most efficient way to support a wide mix of workloads.
The Ground Zero Impact — Expect Significant Retooling
The cumulative impact of these drivers is bringing about a massive retooling in the data center. Server investment levels and growth are projected to increase significantly to support HPC-AI-HPDA workloads through 2021.[
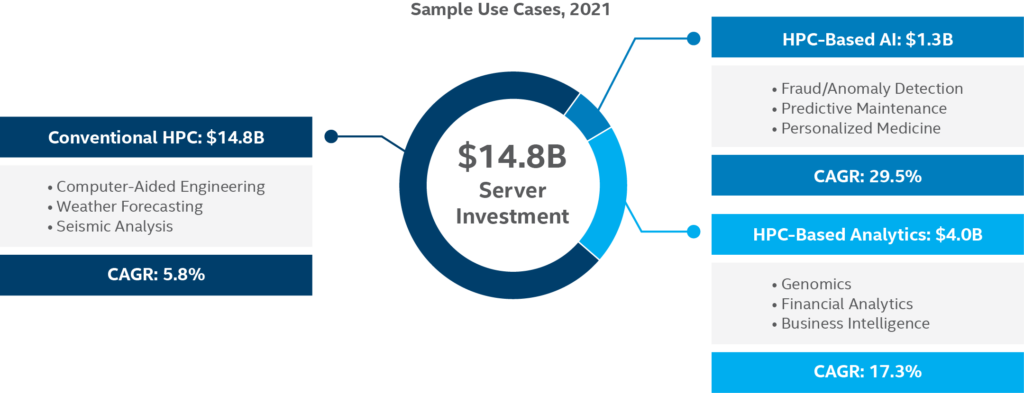
Summary
As discussed in the Supporting Simulation and Modeling, AI, and Analytics on a Common Platformbrief, many organizations follow an old trend to adopt AI and HPDA as distinct entities which leads to underutilization of their clusters. To avoid this, clusters can be converged to save (or potentially eliminate) capital expenditures and reduce OPEX costs.
Intel technologies’ features and benefits depend on system configuration and may require enabled hardware, software or service activation. Performance varies depending on system configuration. No product or component can be absolutely secure. Check with your system manufacturer or retailer or learn more at intel.com.
Esther Baldwin is an AI Strategist at Intel Corp.
Speak Your Mind