Natural Language Generation (NLG) is arguably the nexus point of natural language technologies. It utilizes Natural Language Processing (NLP), is a prerequisite for conversational AI, and largely requires Natural Language Understanding (NLU) for meaningful responses to interrogatives or commands.
When included within a holistic platform, however, the proper implementation of NLG does more than generate natural language communication from cognitive computing systems. Similarly, its capabilities transcend merely understanding natural language to actually produce relevant responses to questions and user dictates.
Credible solutions are able to couple this technology with a plenitude of analytics approaches to analyze datasets on their own, then present the results in natural language. The enterprise value of this widespread functionality is reviewed in a recent Forrester analyst report, “The Total Economic Impact of Arria Natural Language Generation”.
The findings reveal this solution delivers a 209 percent ROI over three years, with a Net Present Value of more than $3 million. It also indicates users obtain an 80 percent reduction in the labor required to manually generate reports.
The ability to synthesize queries in natural language, analyze a plethora of data sources, and present the results in natural language, is inseparable from the organizational value this technology yields. Due to its inherent linguistic appeal it also supports an array of use cases, from increasing the scale of reporting to democratizing analytics throughout the enterprise.
“What people want to do is articulate what they want in a fast, natural sort of way, rather than applying filters and drilldowns and toggling sliders and things,” Arria NLG CTO Neil Burnett explained. “For the most part, people are really good at articulating themselves in words.”
NLU
The triad of capabilities with which holistic NLG solutions like Arria NLG’s are able to avail the enterprise of via natural language technologies begins with NLU. This subset of NLP enables users to communicate with the NLG platform in natural language. This application of NLU is contiguous to Natural Language Query, which allows users to ask natural language questions that are transformed to queries of the underlying data sources involved.
With these natural language capabilities, users aren’t necessarily limited in the nature of their questions or in how they phrase them—both of which are inhibitors for traditional BI tools, which don’t support natural language commands or questions at all. “It’s not like a predefined set of questions, like a chatbot system,” Burnett explained.
Backend Analytics
The backend analytics that Arria NLG offers are regularly updated to allow users to expand their assortment of questions to get answers that are helpful. This point of distinction is vital to the value derived from NLG systems. Other implementations of this technology depend on BI or Artificial Intelligence tools for data analysis—which Arria’s solution integrates with as well. However, the company’s solution can also perform its own analytics of data—such as those in a CRM system like Salesforce, for example—to provide increasingly tailored responses to the sort of information users seek.
With this approach, “the first and most important thing you do is analyze the data,” Burnett commented. “You bring your data to us afresh, or your data updates underneath. There’s no need to retrain our system. Our system is sitting there ready and waiting to analyze the data and respond.” The CTO characterized the type of analytics involved in this solution as statistical analysis, trend analysis, and anomaly analysis, among other types.
Generating Natural Language
The final component of this approach is the proper phrasing of responses in natural language, which is highly important for providing users results in a manner they understand. Burnett identified a use case in which “your new monthly sales figures come in; there’s no work on the system. It’s going to treat them as completely new because it knows how to analyze those things, what’s going to be important to you, and how to articulate what it’s found there.”
The delicacy of employing the correct language, diction, and semantics for the task of generating natural language is at the core of NLG. The whole point of this technology for analytics applications is to take what very well may be complicated statistical findings and make them comprehensible to technological laymen that are business-savvy. “It’s important to choose and use the right words that fit with what the data wants to say,” Burnett revealed. “It could be as simple as thresholds or ways to call something significant, which will vary depending on what aspect you’re looking at.”
Triple Threat
Arria NLG’s solution—and the recent Forrester research quantizing its value to enterprise users—illustrates that comprehensive NLG solutions offer three principle benefits. They support queries in natural language, responses in natural language, and backend analytics, devoid of external tooling (which can be employed if desired), to get the right answers users need from their data.
About the Author
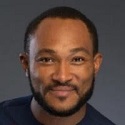
Jelani Harper is an editorial consultant servicing the information technology market. He specializes in data-driven applications focused on semantic technologies, data governance and analytics.
Join us on Twitter: @InsideBigData1 – https://twitter.com/InsideBigData1
Sign up for the free insideAI News newsletter.
Speak Your Mind