The United Nations Office on Drugs and Crime estimates that $2.4 trillion is laundered through the World’s financial markets and banking systems each year. The money then goes on to fund activities that often exploit the most vulnerable members of our communities. This includes activities such as illegal arms dealing and terrorist financing, human trafficking, forced prostitution, and drug trafficking.
It’s a global issue that the World’s authorities know is happening, but they aren’t able to do more to stop these individuals. Why? Because bad actors, with the tactics they employ to ensure anonymity, using ploys such as shell companies, are able to operate largely undetected. There are many challenges in uncovering these criminal networks. Unfortunately, the networks aren’t well documented for these shell companies. The available data doesn’t even talk about the beneficial owners, and most banks and jurisdictions often don’t even require that the information be recorded.
The secret to stopping the money flow for these heinous crimes ultimately lies in data, but there are challenges within the data itself that complicate investigations further.
THE DATA QUALITY ISSUE
The first challenge in investigating financial criminal networks and undetected relationships is figuring out what information banks and companies record. The second issue lies in the accuracy of that recording. Regardless of what is actually documented, if the data is inconsistent, it poses a major issue in linking criminal networks.
For example, say my name is Elmer Fudd but some recordings may have my name as El Fudd or Elmer G. Fudd. Many systems don’t have the ability to understand that each of these names are the same person. The same goes for addresses. When reviewing the addresses “Sesame St.” and “Sesame Street,” the computer is unable to register that the variation belongs to the same location. Financial criminals exploit these inconsistencies, and may use different taxpayer identification numbers with variations of his or her name to their benefit.
The issue of data quality is already prominent, but grows exponentially when you add in the fact that there are endless sources of information existing in siloed paper documents, disparate electronic systems, emails, and other unstructured data. With the amount of “Elmers” and “Sesame Streets” floating around, reconciling and linking these bits of information in a timely manner with manual effort alone is impossible.
CATCHING SOPHISTICATED CRIMINALS REQUIRES SOPHISTICATED NETS
Money launderers continue to evolve their techniques and by the time traditional approaches detect these bad behaviors, it’s far too late. To even keep pace with these criminals, you must be able to do 3 key things:
1. Bring together all of the data available – connecting all of the bits
2. Make it usable – addressing issues in data quality and inconsistencies
3. Expose the context and relationships within it to identify bad actors.
Considering that time is of the essence in these investigations and the magnitude of the effort that goes into pulling this off, the only answer is automation.
Network theory systems, together with AI, have the ability to rapidly clean and link large volumes of raw data in order to provide standard, consistent information that can actually be put to good use in the fight against financial crime. Sophisticated software of this nature offers the ability to integrate internal systems with external, publicly available data like offshore leaks, allowing the information to be collected in one location from a wide variety of sources. In utilizing this intelligence to automatically construct networks, entities across systems can be contextualized, making sense of the small variations in beneficial owner and company names, addresses, etc. Once the data is integrated, connections and patterns can be drawn between the data points that are the same people, linking El Fudd and Elmer Fudd as one.
Not only does leveraging AI enable improvements in data quality and record linkage, but it addresses the rapid pace at which things change and evolve in financial crime. By automating these processes, investigators can spend less time connecting the dots and win back crucial moments needed to ensure that they are able to catch criminals in time to make an impact.
THINKING BEYOND FINANCIAL CRIME
The use of network theory and AI are perfectly suited to address the pain points of investigators looking to make moves against those committing financial crimes. Issues of data quality and speed, however, are not unique to the financial crime category. Messy data causes issues in almost every place that data is used, and the cleaning and organization of this data takes so much time and effort that the massive amount of data that organizations have access to rarely gets used at scale. By automating the path to usable data, organizations and users can unlock the full potential of these assets, revealing once hidden connections between data points and generating valuable insights.
About the Author
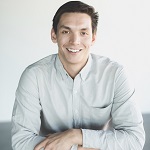
Chris Cardwell is the Product Go-To-Market Lead for unicorn data analytics company Tresata. Cardwell joined Tresata in 2017 as a senior data engineer. He has helped develop Tresata’s product strategy as a radical new approach for businesses to understand, use and monetize every bit of data at absolute scale to solve today’s most complex business challenges. During his time at Tresata, he has led multiple global implementations of Tresata software across key verticals and use cases like health, finance and consumer retail. Cardwell currently spearheads initiatives to apply the Tresata platform to solving audacious problems, including ending money laundering and financial crime through Bad Actor Discovery as a Service (BADaaS).
Sign up for the free insideAI News newsletter.
Join us on Twitter: @InsideBigData1 – https://twitter.com/InsideBigData1
Speak Your Mind