January was National Radon Action Month, a time when the US Environmental Protection Agency (EPA) encourages homeowners to test for and mitigate against the deadly effects of radon gas. While modern radon monitoring devices can detect the presence of the radon with speed and accuracy, reports reveal that too few people take advantage of the technology. In response, scientists are turning to statistical prediction methodologies for help with preventing radon-related deaths.
The dangers of radon gas
Exposure to radon is the first leading cause of lung cancer among non-smokers According to the EPA, radon-related lung cancer deaths total 21,000 per year in the US alone. Exposure occurs when uranium and radium in soil break down over time and release colorless, odorless, and tasteless radon gas. Outdoors, radon quickly dissipates. However, when it finds access into buildings through cracks or other holes in foundations, it can accumulate to dangerous levels. The EPA has found that 1 in 15 homes in the US has elevated levels over their recommended threshold for taking mitigation actions. .
Detecting the presence of radon
A wide variety of solutions exist for detecting the presence of radon in homes and other buildings. Basic radon detectors, sometimes referred to as passive detectors, expose special materials to the air in a building for a period of time ranging from a few days to a few months. The detection materials are then sent to a lab to be analyzed and assess radon levels.
Radon monitors, sometimes referred to as active detectors, use more advanced electronic technology to continuously measure the levels of radon in air over extended periods of time. Because a wide variety of factors can affect the level of radon in air, continuous radon monitoring is typically seen as a better method for protecting against the dangers of radon, especially given that radon levels are known to fluctuate with air pressure and temperature conditions.
Predicting radon levels with geogeny studies
To assist in identifying areas where radon is most likely to be found at dangerous levels, scientists have recently begun employing statistical machine learning methodologies. While radon can be present in well water and natural gas, it predominantly makes its way into homes and other buildings through the soil upon which a building is constructed. When radon detection data is combined with data on local soil composition, machine learning algorithms can help to identify what environmental conditions are most likely to result in elevated radon levels.
In a recent study in South Korea, scientists combined data from radon testing with environmental data including mineral composition of the soil, elevation, water table, and lithology of rocks, allowing probabilistic and deep learning algorithms to determine what combination of factors led to the highest levels of radon.
In another study in the US, researchers even considered other factors such as atmospheric variables, urbanization, community economic well-being, and monthly and yearly variations in assessing spatial and temporal radon level variations. The data they gathered was used to develop better prevention and mitigation control strategies aimed at alleviating and reducing occupants’ exposure to radon in urban areas.
Active Wi–Fi enabled electronic-based radon detectors, which continuous monitor radon levels are available with geolocation. With proper permissions, their capabilities will enable the construction over time of increasing detailed mappings of the most risk-prone areas. With further incorporation or creation of other databases, potentially one day it will be possible to issue alerts to certain types of home construction which have the potential for elevated radon risks that are most likely to present during certain seasons or under certain weather conditions. ,
The dangers of radon are evident. Unfortunately, too few people are aware of them. With the help of active monitoring and machine learning techniques, future researchers may for the first time have the tools to focus actions where they are most needed. These may involve future public policy measures based on prediction to target outreach, subsidize testing, and possibly even mitigation, on a regional and/or income level basis. Such measures might possibly target homes, schools, and offices, to make the greatest possible difference to health outcomes for the greatest number of people given available funding. Those involved in public policy, radon advocacy, and public health may wish to take note.
About the Author
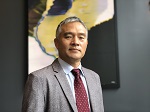
Insoo Park is CEO of Ecosense Inc., an innovator in the radon gas monitoring industry providing people peace of mind through its intelligent and highly accurate radon detectors for homes, educational campuses, assisted living centers, community centers, and commercial buildings. The company’s smart real-time radon detectors utilize a patented ion chamber detection technology with high accuracy performance capable of delivering the first radon result in minutes not days.
Sign up for the free insideAI News newsletter.
Join us on Twitter: @InsideBigData1 – https://twitter.com/InsideBigData1
I was looking for information on machine health monitoring. I read your article and it provides me the great information about the vision for remote health monitoring with devices. Very impressed with this article.