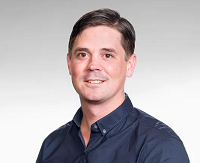
I recently caught up with David Willingham, Principal Product Manager, MathWorks to discuss the evolution of data-centric AI and how engineers can best navigate – and benefit from – the transition to data-focused models within deep learning environments.
insideAI News: What is the impact of data quality on AI modeling, and how can engineers evaluate and optimize the data entering and emerging from AI models?
David Willingham: Data-centric AI is becoming increasingly popular among engineers for solving application problems and improving workflows. The quality of data that models use to perform is crucial to the accuracy and quality of the model’s outcome. By having a data-centric approach, engineers can remove corrupt data or add features to applications to help execute a training job quicker, secure better results, and have higher quality data fed into a model – yielding better overall results.
insideAI News: How can engineers align the needs of a particular domain or application to the data needed to run a successful AI model?
David Willingham: Engineers are increasingly looking to apply AI to their domains, building models with both image and signal data. For example, engineers can leverage signal-based apps, which are non-image related, to help align the needs of domains and applications to the data needed to run the AI model. However, the apps require extraction and engineering to take the raw data and improve the application. Signaling domain experts can also convert and test an audio signal or feature algorithm to build a successful model.
This isn’t limited to just signals either. For example, MathWorks Medical Imaging Toolbox labels the domain specific data (i.e., medical images).
insideAI News: How can engineers implement successful data optimization techniques including image optimization, noise elimination and code development?
David Willingham: Engineers have to take a multi-faceted approach to data optimization and implementation due to the dynamic nature of data-centric AI. To implement successful optimization, engineers have to do multiple tests to find the final result. Once engineers find the data to train their model, it can be time-consuming to build the testing framework. At MathWorks, we recommend users look at using the experiment manager app to speed up the testing process. The low code app will experiment by running trials and tests to help find the best fit which saves the engineer time while ensuring efficiency.
insideAI News: How can engineers implement best practices emerging from data-centric AI such as reduced order modeling and data synchronization?
David Willingham: There are a few applications in industry that are benefiting from taking a data-centric approach. These include reduced order modeling, data synchronization, digital pre-distortion and image object detection.
There are certain traditional modeling techniques that are expensive and time-consuming to simulate and produce a lot of data. For example, CFD (computational fluid dynamics) models or large system models that simulate cars or aircraft. Reduced-order modeling is an approach where an AI model is trained off the data these traditional simulators produce to create an AI equivalent that will run faster whilst still producing similar results.
Data synchronization is a practice applied when multiple input data sets are being used. By merging correctly, ensuring that the data being used aligns, it increases the quality of data that is used to train an AI model and thus produces more accurate results.
Data-centric AI has also brought a new approach to designing digital pre-distortion filters, which are used to offset the effects of nonlinearities in a power amplifier in wireless communications. Typically, nonlinear behavior is characterized in advance using some form of polynomials. However neural network-based techniques are showing more promising results as the data they produce offer better performance than the traditional polynomial.
insideAI News: What are examples of practical benefits of data-centric AI within beneficial real-world applications?
David Willingham: Data-centric AI is dynamic and being applied across industries in manufacturing, aerospace, healthcare, automotive and other industries.
The benefits of data-centric AI allow for new areas of applications that have not been explored before and opens opportunities in the field of engineering from 5G communications to LiDAR, medical device imaging, state of charge estimations, and more. Data-centric AI is leading to improved data quality and model accuracy and has the potential to drive a greater impact on society through its increased use and push for collaboration.
Sign up for the free insideAI News newsletter.
Join us on Twitter: https://twitter.com/InsideBigData1
Join us on LinkedIn: https://www.linkedin.com/company/insidebigdata/
Join us on Facebook: https://www.facebook.com/insideAI NewsNOW
Speak Your Mind