In the modern data landscape, there has been much discussion of the need to break down data silos for faster, more effective results. Though modern innovation is beginning to bring down some of those technological walls, adjusting one’s mindset to embrace a new paradigm is important. It is important not to build new barriers to replace the old.
Historically, because of the limitations of available technology, data-driven business needs would be broken up into individual, more easily surmountable use cases. To go from the inception of an idea to the delivery of business value, businesses would string together a chain of dependent solutions in series.
This model is like a relay race. With each “leg,” the baton is carried by different technologies and people until it is handed off to the next. Just like in a high-stakes 4×100 race, these baton passes are bumpy, risky, and slow. In response, data teams deploy real energy and big brains to design and implement these intersections. Marrying one part of the solution chain to the next requires precise execution.
Cloud computing has changed this process only slightly. It has simplified the joining of technologies but is unable to paper over the inconsistent architectures, data dispersion, performance compromises, and need for custom masterminds. The cloud has made the relay easier to engineer, but it has not fundamentally changed the race.
To eliminate the dislocation and risk associated with the relay race approach, we must we need to break down the mental barriers and compartments we have created for ourselves to truly see the borderless world in which the data lives and should be worked within. This requires us to ditch the bucketing that has grounded our historical view of data projects and embrace three truths:
- Data exists in a continuum of time. Data is often in a state of flux, changing from moment to moment. A human heartbeat, signals from an IoT device, the processed words at the coffee shop drive-thru make clear that data is dynamic. At times, the static, historical batch data matters. At others, the new, up-to-the-second, event-like stream data takes precedence. More often than not, however, both – and everything in between – matter. We can’t limit our imaginations by thinking of these data separately.
- Data will be produced by and used for a variety of technologies. The software industry pushes the notion that applications and analytics are distinct universes. We all know otherwise. They are simply different manifestations of data meets software. Processes consume and publish data and benefit from having a variety of languages and tools readily available. Categorizing use cases may be helpful for human communication, but terms that erect artificial barriers should be left at the door when contemplating systems.
- Data is used by people, not roles. Developers, DBAs, quants, data scientists, business analysts are not actual people. They are simply labels of roles, intending to give guidance about skills, interests, and responsibilities. But the labels are messy and not comprehensive. Team sports don’t look like a relay race. Data systems must support having many players on the court at the same time, each doing different tasks.
Technologies that attempt to unify disparate solutions will not win. Because addressing interesting problems requires complex teams – and because tomorrow’s challenges are impossible to predict today – a single, shared, central framework for data-driven solutions is needed.
In embracing singularity, however, we can demand depth, range, speed, interoperability, and continued evolution. We must view data infrastructure solutions as a dynamic whole or be relegated to a slower, siloed relay race. When all data problems are viewed through the lens of how data needs to meet software, and diverse teams are well equipped to work together, we can deliver a frictionless data world free from the current limits of intermediation.
About the Author
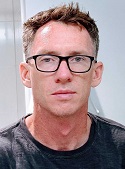
Pete Goddard is the CEO and co-founder of Deephaven Data Labs, a data company building software for modern data teams. After founding quantitative trading company Walleye Capital in 2005, Pete and his engineering team were searching for ways to help quants, data scientists, developers, and portfolio managers discover and evolve strategies and signals more quickly. After witnessing how Walleye benefited from the solution they built, Pete took those engineers, the data system, and its related IP out of Walleye and formed Deephaven as an independent company.
Sign up for the free insideAI News newsletter.
Join us on Twitter: @InsideBigData1 – https://twitter.com/InsideBigData1
Speak Your Mind