In the legal world, and in particular the world of electronic discovery, artificial intelligence (AI) has been around for more than a decade. It is no longer unusual or controversial for organizations to use AI technologies in litigation, especially where large or complex data sets are involved. Legal teams now routinely turn to AI to defensibly accelerate the process of identifying documents likely to be responsive to requests for evidence.
Innovations like technology assisted review (TAR), for example, rely heavily on machine learning and natural language processing to make connections and identify patterns within a body of data in a matter of seconds. This is work that would take even the most qualified human reviewers many, many hours to do manually, and with less accuracy.
Apart from sheer computing power, one of the most useful features of AI technology like machine learning is its ability to quickly “learn” and continuously improve the accuracy of its outputs with the essentially passive assistance of human reviewers. In continuous active learning (CAL), now a feature of leading eDiscovery platforms, even the process of “training” machines to find what you’re looking for is performed algorithmically with no direction from human document reviewers beyond the coding or labeling they perform in the process of manual review. This is a remarkably efficient and cost-effective way to teach machines to identify responsive information, and it has enormous potential for other vital corporate functions. A notable example is compliance.
The usefulness of active learning as a proactive compliance and information governance tool has only recently begun to be explored and appreciated. Across the corporate landscape, reactive approaches to potential problems hidden in data stores are far more common—and ultimately more costly and risky. Companies will typically wait until a whistleblower complains or an employee happens upon a potential problem, and then respond by launching an internal investigation.
AI technology can help your organization avoid this scenario. You can use it to:
- Look for potential “privacy holes” in your data. This is especially relevant as national and local governments enact regulatory frameworks like the recently passed California Consumer Privacy Act (CCPA) and the EU’s General Data Protection Regulation (GDPR). If your organization is sharing information between a California office and a New York office, for example, you can use AI to run regular checks to identify and flag transfers of data that may contain personally identifiable information (PII). This would be particularly relevant for organizations working with distributors or resellers across multiple national or international jurisdictions.
- Proactively identify potential issues in the HR domain, such as mental distress, inappropriate or offensive messages, a rapid decline in employee performance, or perhaps a misuse of corporate accounts. You can also use AI to efficiently collect and analyze information from custodians who are the most likely to be involved in a particular instance of potential misconduct.
- Identify data security weaknesses or potential data breaches before they explode into an existential crisis that poses a serious financial, legal and reputational threat to the company. This is especially relevant in the context of recent pandemic-related work-from-home mandates, which have introduced new security vulnerabilities as more data is accessed and transmitted across multiple locations with multiple devices, including employees’ personal devices.
- Address and correct instances of human error due to poor due diligence, ineffective processes, lack of training, or other systemic shortcomings. At large financial institutions, for instance, compliance officers must monitor massive amounts of data related to transactions, customers, and operations. AI is very good at identifying anomalies, oversights and outright calculation errors in data that might otherwise be missed.
- Analyze the workflows and work product of compliance officers, use that information to categorize those activities and the associated data, and alert those officers to impending deadlines, updates, events, and other time-sensitive tasks.
This handful of examples represents only a small fraction of potential use cases for AI in compliance and governance activities. Every industry will present a different set of use cases. Nevertheless, enterprises in just about every vertical face daunting compliance challenges requiring the identification of data-based risks in vast repositories of structured and unstructured data. This data is generated by hundreds or thousands of applications operating within diverse and often poorly integrated systems. This is the kind of environment where AI shines.
If your organization is already using an eDiscovery platform with built-in AI tools, it might make sense to explore how you can use those tools for broader data management, information governance, and risk mitigation purposes. As you run regular “health checks,” you will get a better understanding of your data and your approach to data-based compliance will be more proactive and cost-effective. That means fewer investigations in response to potential issues and, in many cases, less litigation overall.
About the Author
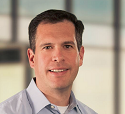
David Carns is the Chief Revenue Officer of Casepoint. He joined Casepoint as a Director of Client Services in 2010, rose the ranks to Chief Strategy Officer until his most recent promotion in 2019. In addition to being a recovering attorney, David possesses a lifelong passion for technology and its advancements. His career has always found him at the intersection of technology and the legal field given his intimate knowledge of both. Carns holds a Juris Doctorate from The John Marshall Law School and a Bachelor’s degree in Philosophy from DePauw University.
Sign up for the free insideAI News newsletter.
Join us on Twitter: @InsideBigData1 – https://twitter.com/InsideBigData1
Speak Your Mind