As the tech industry hype cycle continues to churn my in-box every day, I find myself reflecting on the meme du jour of “artificial intelligence.” My initial reaction to over-hyped terms is to resist giving them more credence than they may deserve. “AI” associated with just about everything falls in line with my reticence. Somehow all new products and/or services are related to “AI”: AI-based, AI-powered, AI-fueled, AI-motivated (oh come on!). Whether the product/service truly includes AI technology under the hood is debatable. Having an “if” statement in the underlying code does not an AI system make. That’s how I got the motivation for one of my regular columns here at insideAI News, “AI Under the Hood.” I wanted to challenge companies to come clean with how exactly AI is being used. Some companies take the bait, while others … to be charitable, view their use of AI as “proprietary.”
But as I think more about the AI frenzy in 2022, I consider this accelerating trend as more than just a fad. One big reason is that, unknown to many, AI is actually a very old term dating back to 1956 (although the discipline has morphed considerably since its early days). It was this year that Darmouth Summer Research Project on AI took place in New Hampshire. The seminal meeting attracted a small group of scientists met to discuss new ideas and the term “artificial intelligence” was first coined by American academic John McCarthy. During the intervening decades since, AI survived a number of so-called “AI winters,” and this long journey has delivered the technology in its current form today. There might be something to this AI stuff! AI actually includes both “machine learning” and “deep learning.” The slide I got below from an NVIDIA GTC keynote address encapsulates the lineage well.
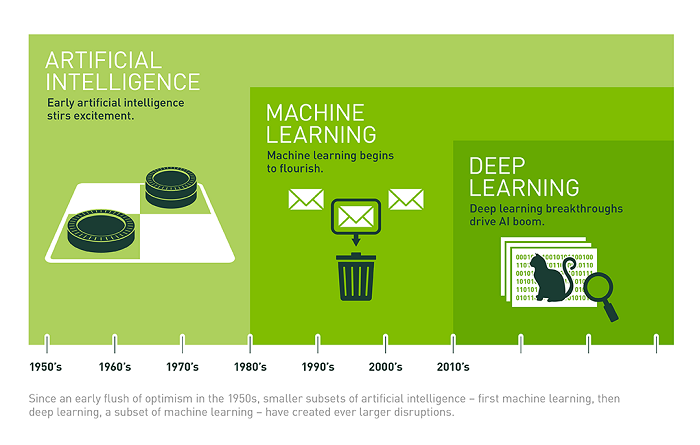
AI, it seems, has some real longevity. That should command some respect, and it’s a strong reason why I’m embracing the term today, albeit for different reasons than some of my PR friends. As a data scientist, I know this field’s long history, and I’m happy, maybe even energized, to give it a pass. I’m still on the fence with other hyper-hyped terms however, like “metaverse,” “web3,” “cyber currency,” and “blockchain.” I have a ways to go to see if they’re able to earn their street cred success.
Giving credit where credit is due, NVIDIA helped propel AI out of it’s most recent “winter” around 2010 when the company wisely figured out that its GPU technology that found such success in the gaming arena could be applied to data science and machine learning. Suddenly, the limitations in doing AI, more specifically deep learning, in terms of compute and large capacity data storage was no longer a worry. GPUs could take on those linear algebra equations found in contemporary algorithms and run them in parallel. Accelerated computing was born. Training times dropped from weeks or months for the larger models, to hours or days. Amazing! Personally, I don’t think we’ll be seeing any more AI winters unless one is triggered by the lack of progress with AGI.
As a data scientist, even I have my qualms about the term “data science” and whether it will survive the test of time. When I was in grad school my field of study was “computer science and applied statistics” because “data science” wasn’t a thing yet. I am pleased today because now I can tell people what I do and earn their interest and respect to a large degree. If I’m at a cocktail party and some asks the proverbial “So, what do you do?” I can now proudly respond with “I’m a data scientist,” and many people will kind of get it. Before, saying I work in computer science and applied statistics would result in stares and quick exits. Now, I find I get questions, and sometimes I even draw a circle of people around me to hear what I have to say about technology trends. Suddenly I’m cool with a cool professional designation.
Not everyone is confident with “data science” longevity. A few years back my alma mater UCLA was designing a new graduate program to accommodate the accelerating interest in what people were calling data science. At the time, many schools were working fast to create a new “Masters in Data Science” program, but UCLA took a more strategic, long term approach. After much consideration, they opted for “Masters in Applied Statistics.” It’s really the same thing, it’s all in a name. I understand their decision. Who knows what my field will be called in 10 years, but “applied statistics” will be around for the foreseeable future!
For a detailed history of the field of AI, please check out the compelling general audience title “Artificial Intelligence: A Guide for Thinking Humans,” by Melanie Mitchell, CS professor at Portland State. A wonderful read!
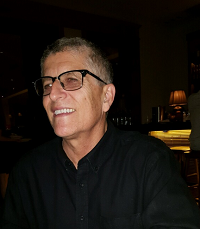
Contributed by Daniel D. Gutierrez, Editor-in-Chief and Resident Data Scientist for insideAI News. In addition to being a tech journalist, Daniel also is a consultant in data scientist, author, educator and sits on a number of advisory boards for various start-up companies.
Sign up for the free insideAI News newsletter.
Join us on Twitter: @InsideBigData1 – https://twitter.com/InsideBigData1
Nice article, Very knowledgeable and informative article about Artificial Intelligence.
Can u help me to become an Data scientist…
Greetings! Yes, as I tell all my “Intro to Data Science” students, reading insideBIGDATA regularly is a great way to be state-of-the-art in the field. Keeping current is one important way of becoming a data scientist.
Hello there! Myself Ram. I had wanted all my college day’s.. Now I have decided to focus on my career. So I choose data scientist as my role. If you tell exactly what to do I will surly follow it with out any regards… I don’t have a proper guidance.. Can you please help me with this… If you did so surly I’ll be a data scientist soon😅