Machine learning is growing, and businesses are taking advantage of the impressive results some of these data analytics tools provide. One of these tools is the recommendation engine, which can be used to improve businesses across industries, from media to e-commerce and more.
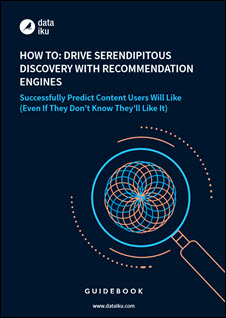
Download the full report.
A new report from Dataiku provides a high level overview of recommendation engines and how they can predict content users will like, and drive more eyes to your sites and online material. The report, “How to: Drive Serendipitous Discovery with Recommendation Engines,” starts with the what and why of the recommendation engine. Ultimately, recommendation engines, also known as recommender systems, are used to predict what users might like, which can be especially useful when there are a lot of choices available — for example, Amazon or Netflix. A recommendation engine can also be used to choose which content to surface without giving the user a choice.
After studying the “what” behind the recommendation engine, the “why” becomes clear. Using these tools, businesses can expose viewers to products or content they might not have otherwise found, or known to look for.
There are two primary types of recommendation engines — Collaborative Filtering and Content-Based — that the report covers in detail, along with sub-types of each. The Collaborative approach primarily makes recommendations based on actions from other people, according to the report, while content-based systems make recommendations based on the user’s purchase or consumption history.
Recommendation engines can be an effective way to expose users to content they may not have otherwise found, which in turn can forward larger business goals like increasing sales, advertising revenues, or user engagement.
The next question is “how”? Thankfully, Dataiku points out building a recommendation engine can actually be relatively straight forward. That said, the report gives seven steps to consider when completing a data project for building a recommendation engine. These steps cover stopping to understand your business needs, as well as enriching data, and go all the way to deployment.
After outlining the seven steps to recommendation engine deployment, Dataiku also covers some potential pitfalls of these machine learning tools. For example, it’s important to pay close attention to scalability when designing a recommendation engine because as your dataset grows, computation costs can grow exponentially. Agility is also something to consider in the planning stages.
[clickToTweet tweet=”Recommendation engines can be an effective way to expose users to content they may not have otherwise found.” quote=”Recommendation engines can be an effective way to expose users to content they may not have otherwise found.”]
Lastly, the new guide from Dataiku covers some of the latest trends and developments in recommendation engines that businesses should consider. These include developments in context-aware recommendation engines, which aim to “provide even more precise content given the context of the user in a particular moment in time,” the report states.
The full report from Dataiku covers the following topics:
- The What and Why of Recommendation Engines
- How to Start Developing Your Own
- What are the Pitfalls?
- Looking Forward
Download the report, “How to: Drive Serendipitous Discovery with Recommendation Engines”, courtesy of Dataiku.
Some great content here, Sarah! Recommendations are a huge part of website personalization that is increasingly critical in today’s trend, as visitors and customers expect websites to adapt to their interests.
While it is possible to develop your own solution, its also worth considering if the IT hours involved are really worth it, when there are comprehensive personalization solutions that include a fully functional recommendation engine plus many other website personalization tools, and some of them aren’t even very expensive.
For example, Personyze has over 50 algorithms for products and content, that can be embedded on the site, in emails, or even in popups:
https://www.personyze.com/recommendation-engine-algorithms/