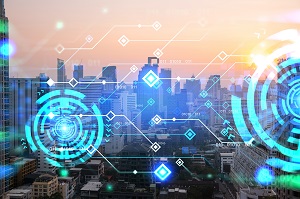
Welcome to insideAI News’s “Heard on the Street” round-up column! In this regular feature, we highlight thought-leadership commentaries from members of the big data ecosystem. Each edition covers the trends of the day with compelling perspectives that can provide important insights to give you a competitive advantage in the marketplace. We invite submissions with a focus on our favored technology topics areas: big data, data science, machine learning, AI and deep learning. Enjoy!
Elon’s ChatGPT Alternative? Commentary by Moses Guttmann, co-founder and CEO of ClearML
AI is entering a new phase of politicization focused on AI neutrality. Unfortunately, the pursuit of neutrality is a game of whack-a-mole that can never be won, leading to poorer quality models trained on incomplete datasets. This dangerous terrain is disappointing and will only lead to further politicization. Ultimately, AI ethicality, not necessarily neutrality, should be the priority when building models. As a first step, dataset visibility and model training workflow visibility are key to creating transparent AI systems, providing insights on the neutrality of the AI models.
Predicting Issues: Using Digital Twins for Large-Scale Simulations. Commentary by Dr. William Bain, ScaleOut Software CEO and founder
Engineers have used digital twins for decades in the field of product lifecycle management (PLM) to aid in the design and testing of a wide range of devices, from valves to commercial jets. However, the power of digital twins doesn’t stop with PLM. They also can enable the construction of large-scale simulations that model complex systems, like airline traffic systems, telematics operations, logistics networks, security monitoring, smart cities, and much more. By simplifying the design of these simulations and helping to deliver scalable performance, simulations with digital twins can reveal important insights about these highly dynamic systems. These simulations can help managers find critical issues buried in complex behaviors and make predictions that inform their decisions. The applications for large-scale simulations are diverse, and the need is pressing. Our modern lifestyle is supported by a complex web of huge physical systems that all must perform flawlessly. Because these systems have so many components and countless unpredictable interactions, it is challenging to predict how they will behave, especially when confronted with unexpected problems. Consider an airline system that must manage hundreds of thousands of passengers and bags, thousands of aircraft, pilots, airport gates, and the rest. Now insert weather delays, pilot scheduling issues, and other service interruptions. How do you predict the ripple effects of key decisions, such as holding and canceling flights? Simulations can help. They allow “what if” evaluation of alternative scenarios to guide decision making. And they can reveal exquisite detail about interactions that other technologies, like ML and big data analytics, may not be able to deliver.
ChatGPT leads to multicloud service race. Commentary by Matt Wallace, CTO at Faction
ChatGPT is an amazing tool, reflected in its meteoritic adoption. It goes without saying it makes glaring, painful errors at times, but I’ve begun using it daily for work and play. While it’s the early days for ChatGPT and its competitors, I also expect a variety of competitors are on the way, for both general use and specific industries/use cases and more. What is interesting to me, however, is the partnership between Microsoft and OpenAI, where the OpenAI APIs are being exposed natively on Microsoft Azure – but not other clouds. This is a trend I expect to see continuing, where cloud providers land exclusive services to try to amplify the appeal of their walled gardens. Meanwhile, organizations will have a greater impetus to adopt a thoughtful multi-cloud strategy, because the power of composing applications that leverage various APIs to access AI-powered services will be too appealing to ignore. Since these services will be integral to the use of data in enterprises, they will want to have their data available in a way that it can be more easily funneled into any AI API in any cloud.
Microsoft adding personality options to Bing AI chatbot. Anita Schjøll Abildgaard CEO and Co-founder, Iris.ai
Microsoft’s latest ChatGPT Bing upgrade comes in the form of a feature which allows users to choose from a selection of personality options that changes the tone of responses. The announcement prompts questions over whether the different personalities implicate the accuracy of the results and whether choosing this feature is a more worthwhile investment than improving the search’s factuality. The more niche searches get, the higher chance that flaws in the training data and underlying model will be exposed. Say a user wants to find the best local restaurants — for this kind of search, a general-purpose Large Language Model (LLM) may be suitable. But for more specialized fields, whether it be in business, scientific research, or beyond, the stakes are too high for anything but absolute confidence in the answer. Bing’s personality choices may further complicate this. If the responses between the personality types vary significantly in content as well as phrasing, users are either unlikely to have confidence in the responses or may believe responses that aren’t suitable. It is far more important for players in the generative search space to prioritize factuality and accuracy rather than fun features that garner attention on social media.
Treat Data as a Product. Anthony Deighton, Chief Product Officer at Tamr
Retailers know that deriving value from customer data is hard work. But the data-savvy ones also know a secret: treat data as a product. Treating data like a product means implementing a data product strategy that brings structure to the ownership, processes, and technology needed to ensure the organization has clean, curated, continuously-updated data for downstream consumption. Data product strategies define key objectives and metrics, such as increasing competitiveness by improving the customer experience or creating product differentiation.
What does this explosion of generative AI mean in the grand scheme of the workplace? Commentary by Parry Malm, CEO of Phrasee
People both overestimate and underestimate the impact ChatGPT is going to have. A relevant example is Photoshop. Back in the day, people did not want to use Photoshop because it was their job to physically be in the cutting room making collages, putting them on to a litho, and printing them out. Fast forward to 2023, and there are more designers in the world now than there ever were in the history of humankind. So what Photoshop has effectively done is created new jobs, making editing less of a specialism and more of a possibility for the average person to use. With this recent explosion of AI, particularly generative AI, the same thing is going to happen. It’s going to absolutely obliterate some job categories, but it’s going to create new job categories like this stuff always does.
Organizations need democratized access to GPUs. Commentary by Kevin Cochrane, Chief Marketing Officer, Vultr
Today, artificial intelligence (AI) and machine learning (ML) are the drivers of breakthrough innovation, but training AI and ML models requires high GPU usage. This means that enterprises looking to hop on the ChatGPT bandwagon and develop their “own ChatGPT,” might run into a GPU bottleneck. In other words, organizations are facing limited access to GPUs, when in reality, developing compute-intensive AI applications such as ChatGPT requires on-demand GPU access, at scale, in any location, without having to provision or configure them. To overcome this challenge and build a custom AI model, organizations need democratized access to GPUs. Fortunately, a new paradigm has emerged that makes cloud GPUs affordable and democratizes access to vital AI infrastructure: fractional GPUs. Fractional GPUs allow organizations of all sizes to rent just the amount of GPU compute power needed to run their AI and ML workloads, avoiding costly overprovisioning of GPU resources. By enabling organizations to easily scale build, test, and production deployment of new AI and ML models, through the cloud, and in the region where the AI teams are located (to avoid data sovereignty issues), organizations can optimize costs while also scaling to match workloads to the processing power required of models such as those underpinning ChatGPT.
How AI is Empowering Growers and Ensuring Global Food Security. Commentary by Thai Sade, CEO and Co-founder of BloomX
The intersection of technology and biology is transforming the future of agriculture, with AI at the forefront of this revolution. The application of AI in agriculture is diverse, ranging from improving pollination processes to optimizing crop and soil management practices. By leveraging AI, growers can monitor crop and soil conditions closely, detect and predict diseases and pests, optimize irrigation schedules, and minimize waste. Moreover, AI can analyze large amounts of data to provide insights into market trends and consumer behavior, empowering growers to make informed decisions about crop selection and pricing. AI-based tools enable growers to plan their planting, irrigation, and harvesting schedules and can support early warning systems for natural disasters, such as floods, droughts, and pests, mitigating risks and reducing losses. Through AI, growers can optimize crop yields, reduce waste, and enhance sustainability, ultimately playing a critical role in ensuring global food security amidst growing challenges.
Digital Transformation and AI Won’t Replace Human Employees, But It Will Make Your Company More Competitive. Commentary by Pavel Kirillov, CTO and co-founder, NineTwoThree Venture Studio
With the recent hype around ChatGPT and the massive layoffs in the tech industry, there is a collective illusion that AI is ready to replace all employees and a “gold rush” sense of urgency to invest in anything “powered by ChatGPT”. Not going to lie, ChatGPT is an impressive milestone in AI and a great tool for some use cases. However, in the game of “doing more with less”, 1 dollar invested in digital transformation will likely yield much more than the one invested in ChatGPT for the majority of established businesses. We’re seeing companies approaching ChatGPT and AI as some kind of silver bullet that will let them cut their workforce in half. In reality, the same companies still do things with pen and paper, Excel, fax machines, printed business cards, outdated software and hardware not integrated with each other, and processes where employees copy and paste data from one form to another. Digital transformation is a safer and more predictable path to unlock new sources of value for customers and create new efficiencies so your existing workforce can do more to stand out in an increasingly competitive marketplace.
How to Create a More Inclusive and Equitable AI Landscape. Commentary by Triveni Gandhi Ph.D, Responsible AI Lead, Dataiku
While AI has revolutionized industries and created business value, it’s important to note that AI built without equity and inclusion can do more harm than good. Since AI is trained on existing data, and anti-Black sentiment is a systematic worldwide problem, these discrepancies often show up in certain applications of AI. For example, facial recognition tools have shown to have higher error rates among images of darker-skinned women, leading to a variety of harms, including wrongful arrests and mislabeling. One of the most immediate ways to create better tech is to include more diverse and varied voices at the table when building and deploying new products. This means hiring more women, people of color, and people from non-traditional backgrounds on all kinds of teams — from business users to developers to implementers. Transparency is another important factor. One of the simplest ways to put this into practice is by visualizing how input data varies across key social groups — such as race, gender, education levels, or even zip code — to assess any underlying disparities before moving forward with a project. Once we acknowledge the limitations and potential harm of AI, we can move forward on actionable solutions and ways to make AI more equitable and inclusive.
Should we pause the AI race? Commentary by Shubham A Mishra, Co-founder & Global CEO, Pixis
We cannot stop, and must not, stop progress but what we can do is plan for this progress. This can be possible only if all of us mutually agree to pause this race and concentrate the same energy and efforts on building guidelines and protocols for the safe development of larger AI models. In this particular case, the call is not for a general ban on AI development but a temporary pause on building larger, unpredictable models that compete with human intelligence. The mind-boggling rates at which new powerful AI innovations and models are being developed definitely calls for the tech leaders and others to come together to build safety measures and protocols. It is a reasonable ask considering generative AI does have the power to confuse masses by enabling the pumping of propaganda or ‘difficult to distinguish’ information into public domain.
World Backup Day on 3/31. Commentary by Jason Lohrey, CEO Arcitecta
As we recognize World Backup Day on March 31st, the focus is on preventing data loss. However, it’s important to understand that the way we have done backup for the past twenty years is broken – especially at scale. As the amount of data continues to increase – both in the number of files and the amount of data generated – backup systems that scan file systems are no longer feasible, particularly as we enter the realms of billions of files and petabytes or more of data. Exponential data growth is driving the need for systems that can prevent data loss without the mechanisms and limitations of traditional backup. A glaring limitation example is that all interim data changes that occur between backups, snapshots or clones will not be captured and cannot be restored in the event of a system failure, user error, cyberattack or other data loss event. For far too long, business leaders have had to accept a level of data loss, as defined by recovery point objectives (RPO), and some downtime, as defined by recovery time objectives (RTO.) New business resilience technologies are now enabling rapid data recovery that approaches the ideal of an RPO and RTO of zero – even at very large scale. Going forward, business leaders can mitigate risk to their organizations by changing their focus from successful backups to rapid, near instantaneous successful data recoveries.
Addressing the biggest challenges for database and analytics management today. Commentary by Max Liu, CEO and co-founder of PingCAP
The recent excitement surrounding ChatGPT signifies an AI technology breakthrough that not only reduces the required programming skillset and democratizes access to data insights, but also improves the three fundamental components of volume, variety, and velocity. Generating the most actionable and valuable insights by effectively managing databases and optimizing analytics poses significant challenges within these three key areas. These challenges arise due to the exponential increase in the quantity and diversity of data, the demand for real-time analysis, and the necessity to merge data from multiple sources. To address these challenges, advanced technology such as in-memory databases, distributed databases, and cloud analytics platforms are leveraged to enable efficient data management. Nevertheless, adopting such complex data architecture is now common practice and presents a new challenge of balancing data capacity and speed. Additionally, the need for more data engineering talent makes it difficult for organizations to adopt a data-driven approach, invest in skilled personnel, and implement effective data governance and security measures. Hybrid Transactional and Analytical Processing (HTAP) is an innovative database architecture that enables organizations to handle both online transactional and analytical processing tasks within a single system. By doing so, it simplifies technology stacks and reduces data silos, enabling companies to gain actionable insights from real-time updates and drive faster growth. HTAP is a powerful tool that can be used to address these challenges by facilitating more efficient and streamlined data processing, leading to cost-effectiveness, faster business operations and quicker decision-making. HTAP can provide companies with the speed, agility, and efficiency needed to gain a competitive edge and to respond quickly to constant changes in today’s fast-paced and data-driven business environment.
Large Language Model Hallucination is a Data Labeling Problem. Commentary by Alex Ratner, CEO, Snorkel AI
Hallucination is one of the major challenges any company that leverages large language models is bound to face. This is because models like GPT-3 and ChatGPT are trained to produce the most plausible-sounding text given some prompt or context. They are not designed to optimize for the accuracy of facts, numbers or stats in that output. They are also not well trained to say “I don’t know”. And often, the response falls short of the truth. This is because, in the end, the model is as good as the data it is trained on, and a lot of the data we produce in the world is unstructured—meaning, it’s unlabeled and unclassified. In short, this is in large part a data labeling problem. A significant blocker enterprises face in using AI including latest foundation models or large language models is the vast volumes of data required to train a model. The vast majority of data labeling is still done by hand, which is costly, time-consuming and error-prone. Ultimately, a model isn’t discerning like a human; it can’t tell the difference between good data versus, say, data containing toxic content.
Is data observability recession-proof? Commentary by Sam Pierson, CTO at Talend
As the business landscape changes, so do the needs of an enterprise and the availability of data to support those needs. The ability to respond quickly and decisively to problems and opportunities is fundamental to success, and data observability is a key component of that ability that shouldn’t be overlooked. Organizations and data teams need to take a proactive approach to data quality, and they must be able to manage the entire data lifecycle. Data observability enables data teams to monitor data flows and react quickly to emerging issues before they create downstream impacts to the business. In this environment, you can’t afford to experience data quality issues. By implementing a data observability strategy, you will optimize business outcomes through this downturn by delivering tailored insights and by remediating broken pipelines. You will also uplevel data governance to increase privacy. Unlocking accessibility and speed of data delivery will be at the core of many advantages. Of course, data observability is a continuous process and must have discrete checkpoints to achieve long-term success. These checks should address the following: accuracy, timeliness, accessibility, consistency, and completeness. Being empowered by data is a superpower in today’s market.
Sign up for the free insideAI News newsletter.
Join us on Twitter: https://twitter.com/InsideBigData1
Join us on LinkedIn: https://www.linkedin.com/company/insidebigdata/
Join us on Facebook: https://www.facebook.com/insideAI NewsNOW
Speak Your Mind