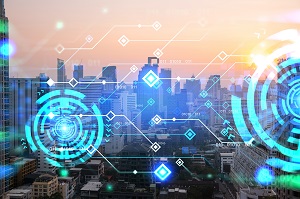
Welcome to insideAI News’s “Heard on the Street” round-up column! In this regular feature, we highlight thought-leadership commentaries from members of the big data ecosystem. Each edition covers the trends of the day with compelling perspectives that can provide important insights to give you a competitive advantage in the marketplace. We invite submissions with a focus on our favored technology topics areas: big data, data science, machine learning, AI and deep learning. Enjoy!
Younger Consumers Have High AI Expectations. Commentary by Zac Fleming, SVP of Product at TimelyMD
The generation that has never known a world without the Internet – or even without smartphones – have different expectations of technology. Whether it is social media, music, gaming, or dating, Generation Z expects that their preferred technology will deliver relevant information and an engaging experience and that AI plays an important role. Why should those expectations suddenly change when it comes to the personal technology for their health? Mental health and well-being virtual care solutions for young adults, for example, are growing in popularity considering 91% of Generation Z reported experiencing psychological symptoms due to stress. AI can play an important role in care delivery and engagement if the algorithms are designed to enable a highly personalized and patient-centric experience. For example, not all young adults are ready for – or want – 50-minute, one-on-one counseling sessions with a mental health professional. AI can be instrumental in accurately analyzing and interpreting intake screenings so their selected care pathway is most relevant and take into account students’ unique health needs, cultural background, sexual orientation, or religious practices. AI can even help guide digital-only care pathways through content and activity selection based on the young adults’ interactions with the solution. The AI continues to deliver an even more personalized experience as its algorithms learn more about young adult, which builds their engagement and motivation to improve their mental health and well-being. These engagement-building concepts have been understood and applied in other industries’ consumer-facing technology for years, it is time for healthcare to catch up.
How open-source will continue to push the envelope on making AI/ML solutions smarter and more useful across the business sector. Moses Guttmann, co-founder and CEO of ClearML
Open source has already played a significant role in the advancement of AI/ML solutions by allowing resources of different contributors to come together. As big data and machine learning usage continues to increase, businesses are relying more and more on AI solutions to improve their operations, gain insights, and make better decisions. By leveraging open source frameworks, businesses can create customized AI/ML models that contribute to faster development of product features and therefore time to market and time to revenue, while reducing development costs. The open source community also fosters collaboration, enabling developers to share knowledge, work together on projects, and build on top of existing solutions, all of which accelerates innovation. Because of this, we expect that open source will continue driving innovation across the business sector in the years to come. As AI technology matures, open source will play an even greater role in advancing different solutions and platforms. Ultimately, we expect that open source will enable businesses to increase efficiency and drive bottom-line impact. For example, the release of ChatGPT and other LLMs, as well as computer vision for public use, is expediting the democratization of AI, and this will continue to accelerate thanks to open source. As a result, AI will increasingly become more accessible to smaller businesses and enterprises alike. Organizations that lack the resources to invest in or create proprietary solutions will now be able to compete on a level playing field. Additionally, open source AI/ML solutions will continue to improve their integration with other business tools and technologies, enabling businesses to use their existing data and infrastructure to develop more market-ready solutions.
Tencent announces plans to rival Baidu & OpenAI. Commentary by Sanjeev Kumar, VP EMEA at Boost.ai
Following Baidu’s recent announcement that it is building its own ‘Ernie’ chatbot to be a challenger to OpenAI’s ChatGPT, it is no surprise to see another Chinese giant, Tencent, build out its team to develop a generative AI solution. In the last few weeks, we have seen a groundswell of interest and enthusiasm for AI-powered chatbots and voicebots, as ChatGPT has raised the profile of this technology in the minds of everyday people. Now, we’re seeing big-name tech players mobilize to build their own offerings, as the public imagination remains captured by the ergonomic interface that conversational and generative AI offers. The race is now on for the Chinese tech giants to become the go-to generative AI solution for Chinese users. Tencent is well positioned to fill this void, and the scope for adoption of any conversational AI platform it creates is huge, given its ownership of the popular social media platform WeChat and the audience it already has captured through the application. However, you don’t have to be a multi-billion-pound company to transform your customer experience with conversational AI. Now is the time for businesses of all shapes and sizes to seize the initiative and place conversational AI at the heart of their customer service offerings as well, as customers become more familiarized with chatbots and voicebots, and expectations increase for a better customer experience.
Despite Some Hurdles, MLOps Solutions are Ready for Take Off. Commentary by David Magerman, Managing Partner & Co-Founder, Differential Ventures
There’s no question that machine learning operations (MLOps) is a burgeoning sector. The market is projected to reach $700 million by 2025 — almost four times what it was in 2020. Still, while technically sound and powerful, from an investment perspective these solutions haven’t generated the expected revenue, which has raised concerns about future growth. Although MLOps tools are critical to companies deploying data-driven models and algorithms, many who are deploying ML-driven solutions lack deep knowledge and experience and don’t recognize the need for the more sophisticated tools or understand the value of the low-level technical integration. They are more comfortable with tools operating on externalities, even if they are less effective, since they are less intrusive and represent a lower adoption cost and risk if the tools don’t work out. On the contrary, companies with ML teams who possess deeper knowledge and experience believe they can build these tools in-house and don’t want to adopt third-party solutions. Additionally, the problems that result from MLOps tools’ shortcomings aren’t always easy to identify or diagnose — appearing as modeling versus operations failures. The outcome is that companies deploying ML-based solutions, whether technically sophisticated or inexperienced, have been slow to adopt. But things are starting to change. Companies are now recognizing the value of sophisticated, deeply integrated MLOps tools. Either they have experienced problems resulting from not having these tools or they have seen competitors suffering from their absence in many high-profile failures, and are now being forced to learn about the more complex MLOps solutions. As a result, those MLOps companies that have survived the revenue winter so far should see a thawing of the market and a growth in sales opportunities.
The lack of trust in AI is carving way for white-box model. Berk Birand, co-founder and CEO of Fero Labs
The importance of trust in AI varies depending on the cost of failure. If you’re using the technology for art, such as creating imagery for video games or movies, the stakes are low. When you get a result you don’t like, you can just start over. However, in situations like health care or manufacturing, mistakes are costly. Trust becomes incredibly important. In those situations, white-box methods are more useful. Not only are they explainable, so you can see how the result was produced, they also include features like confidence intervals, which show how confident the model is in its prediction. These features are crucial to help users make important decisions in high-stakes situations.
How the pandemic brought the equivalent of the gold rush to digital transformation. Commentary by Wilko Visser, CEO of ValueBlue
What a single source of truth means for your data: Data is intangible and exists everywhere in an organization. Yet, the capturing of data is often not centralized. The decentralized nature of data makes it very hard to control. Without central insight into all the captured data and a mechanism to avoid redundancy and check data quality, data organization gets out of hand quickly. Without a central system of record, you’ll often only detect this when it’s too late. A single source of truth allows you to track where data originates and designate origin of truth. This prevents redundancy and incoherence of data, thus improving the quality and traceability of that quality. This is extremely important because data-driven decision-making is only as good as the quality of the data it’s based on. Making data-driven decisions without a single source of truth will often result in poor decisions. On top of that, a single source of truth that’s available always and everywhere also speeds up your decision-making process. Those organizations that can make the right decisions fast will thrive.
Evolution of ChatGPT. Commentary by Anatolii Ulitovskyi, a Founder at UNmiss
As ChatGPT continues to evolve and improve, its impact will be felt across various industries, transforming old job roles such as writers, accounts, and web developers to controllers, operators, and editors. As more businesses and individuals begin to explore the possibilities of ChatGPT, we can expect to see a shift towards more creative, higher-value work that requires a human touch.
AI Co-Pilots and Predictive AI Will Transform the Way We Work. Commentary by Artem Kroupenev, VP of Strategy, Augury
We are reaching a point where every profession will be enhanced with hybrid intelligence and have an AI co-pilot which will operate alongside human workers to deliver more accurate and nuanced work at a much faster pace. These co-pilots are already being deployed with clear use cases in mind to support specific roles and operational needs, like AI-driven Machine Health solutions that enable reliability engineers to ensure production uptime, safety and sustainability through predictive maintenance. As AI becomes more accessible and reliable, it would be extremely difficult, and in some cases even irresponsible for organizations to not operate with these insights given the accuracy and reliability of the data. Executives are beginning to understand the value of AI co-pilots for critical decision making and its value as a key competitive differentiator, which will drive adoption across the enterprise.
Why humans must remain involved and supervise AI to mitigate risks. Commentary by Jon Aniano, SVP Product for CRM Applications at Zendesk
While ChatGPT is being experimented with in almost every industry, it hasn’t been trained to provide a 1:1 conversational experience that meets customers needs – and is far from being 100% accurate. We are seeing a bigger industry shift from AI as a means of automation to a means of creation and the questions remain: What will happen to humans? What will happen to jobs? Despite the hype that GPT has created, it is still imperative that humans remain involved and supervise AI to be ethical and responsible in order to mitigate top CX risks. According to our latest CX Trends Report, 75% of customers expect AI interactions will become more natural and human-like over time, and the ideal evolution of AI will enable customers to ask increasingly complex questions. One of the main CX risks is AI not understanding elements of a unique business. For AI to work efficiently, it needs to understand any of the nuances to how each business operates and what its approach to support and policies are. The way to ensure this is to fine tune AI and ground it in facts. Another main CX risk is AI making mistakes if left unsupervised. AI isn’t perfect and it’s going to keep improving, but ensuring humans are part of the process is critical to its success. It’s important to not just let AI run wild and instead, focus on reinforcement learning of AI models with human feedback. In this approach, AI is learning from human guidance and getting more accurate and efficient with every interaction. One solution to measure for success is to expose a confidence level when AI is involved so humans understand where AI may be making a mistake and know that when confidence isn’t high they absolutely need to review. Lastly, there are CX risks if AI is manipulated or tricked. Due to bad actors trying to corrupt AI (likely unintentional), the foreign engagement is something that it’s not trained for. This is why it’s crucial to narrow the scope of where AI operates and what questions it’s set up to answer and escalate to humans when appropriate. While this technology is still working out the kinks, brands can rely on other forms of AI and automation based on knowledge bases that provide instant answers or drive customers to agents when more 1:1 assistance is needed.
The ChatGPT Caveat – The Importance of Human Oversight. Commentary by Prakash Ramamurthy, Chief Product Officer of Freshworks
ChatGPT has taken the technology industry by storm with its ability to generate content that can at times be indistinguishable from humans. This technology has exciting potential to improve the customer experience with more personalized engagement at scale. Many companies are already integrating chatbots with AI-powered features that allow support agents to leverage AI to assist in developing quicker responses and enhancing the overall customer experience. These features also free up agents’ time and reduce the unnecessary workload and stress that can lead them to burnout, especially when eight in 10 (82%) IT pros report being burnt out. However, ChatGPT currently relies on large language models that draw on the internet—much of which is loaded with bad information. It’s crucial to have human oversight in place to review and edit responses written by ChatGPT to ensure that the information provided is accurate and not causing harm to customers or the brand’s reputation. By doing so, companies can integrate ChatGPT responsibly and leverage its benefits to drive long-term value and boost customer retention.
Communities, collaborations, and companies: Upskilling company talents via data science communities. Commentary by Rosaria Silipo, Head of Data Science Evangelism at KNIME
Communities are often seen with suspicion by the corporate world: a free, public, technical, engaging environment more useful for students to learn than for companies to make a profit. This vision has become obsolete. At a time when data science is critical to accelerating modern decision-making, the need for companies to rely on data science communities has never been higher. Data science techniques and tools are constantly evolving, but keeping up with these changes doesn’t always require hiring more data scientists. Instead, organizations should rely on external and internal data science communities. Many public data science communities live their own independent lives outside of the company. They are a great source for tutorials, examples, use case solutions, blueprints, constructive discussions. There is always something to learn and inspiration to get as an active member of a community. In exchange for all those benefits, there are usually two payments required: sharing your questions and answers publicly and giving back expertise when others ask questions. Some organizations operate with more sensitive data or generally may be hesitant to participate in public communities. In this case, the alternative is to create an internal data science community, where data analysts, data engineers, data scientists, and consumers discuss and learn from each other. In both types of communities, collaboration and communication among the technical data experts is key and it must be paired with an understanding of consumer needs, as consumers are the ultimate end-users and judges of if data science can be put into action effectively.
Upskilling: How to Win the Battle for AI + Data Talent. Commentary by Doug Bryan, AI Strategist, Dataiku
ChatGPT has set off a frenzy around AI—but across tech as well as other sectors like government, there’s a dramatic shortage of AI and data science professionals to implement and optimize AI processes. One way enterprises can get around this shortage is by creating internal programs that promote upskilling among existing data science workers and greater adoption across departments. Hiring hundreds or thousands of data scientists is not realistic for most organizations, especially when budgets are now tighter than ever. Many companies are looking to overcome that gap by upskilling other staff to be data workers. A successful upskilling program needs a common AI/ML platform that ensures workers of all skill levels – from business analysts to graduate-level data scientists – want to use it. An upskilling program should also be designed with the goal of getting 10 to 100 times more people involved with AI development by providing self-service training. When an upskilling program works well, it creates a virtuous cycle where business analysts acquire AI skills, they create value, which increases awareness of the value of AI, and new users are identified or raise their hand to be upskilled. Companies that do this well will generate a sustainable flow of AI talent and drive ROI across many business units and functions.
Generative AI: The Next Frontier for Enterprises. Commentary by Raghu Ravinutala, CEO & Co-founder, Yellow.ai
ChatGPT and generative AI have taken the world by storm. By far, it has been the fastest adoption of a new technology. With such fast adoption, LLMs (Large Language Models) fine-tuned for proprietary enterprise and domain data will gain more enterprise adoption. Language models like BERT and GPT-2 have already been leveraged in advanced conversational AI systems like ours, primarily for understanding conversations and generating training content for new intents. However, with the advent of extremely large language models (175B+ parameters), these systems have demonstrated the ability to generate verbose, human-like text. As a result, the incremental cost of generating new content rapidly comes down. In an enterprise context, this will drive use cases such as generating support articles, hyper-individualized marketing campaigns (imagine unique content for each user), and HR policies. The general-purpose LLM will not work for these; instead, we will need domain-specific LLMs that are built or fine-tuned on massive amounts of proprietary data. The extreme low cost of generating versions of interactive text will lead to companies dynamically generating multiple variants of text to convey the same information or take the same action and using reinforcement learning to optimize for the variants that lead to the best conversion. We can see support and marketing interactions autonomously and continually improve conversions with continuously improving text variants. Furthermore, when it comes to voice automation for customer support, generative AI will enable dynamically changing personalized voices that will replace the stale, similar-sounding robotic voices of call customer support, thereby elevating the customer experience at scale.
Data-driven businesses are the future – how do you get there? Commentary by Maryam Khalili, Sr Manager, Data and Analytics for Appnovation
42% of organizations surveyed by Insider Intelligence want to establish a data-driven business culture, but most companies don’t have a clear and effective strategy to advance their data maturity. Data maturity spans a spectrum, and progressing your data strategy requires having a firm grasp of the starting point. Companies are at all different stages: whether they’re using data on an ad-hoc basis or have difficult-to-access or disconnected data. Maybe the data is centralized and a part of decision-making, but not clean. Ideally, companies want to be data-guided. Data is like water; it flows clearly and quenches the thirst for insight with actionable findings. Data-guided organizations use all the information at their disposal: whether measuring marketing campaigns, establishing KPIs, setting product pricing, investing in shelf placement, or evaluating changes across a range of channels. An essential element of becoming a data-guided organization is clean data, allowing for ease of use, consistency, and accuracy. It makes the data more trustworthy, and the insights gleaned from it more meaningful. Second, consider people are involved – there is a lot of sensitivity around data sharing, even internally. Don’t lose sight of the fact that you’ll likely end up with a dashboard or digital product that you want people to use. Be sure to show them the benefits. Finally, data can only tell you what is happening. Data sets the stage, but research puts on the show. Ultimately, advancing your data maturity requires a continual process of refining, defining, and utilizing your data with intentionality and purpose. While it doesn’t happen overnight, becoming a data-guided organization stands to enhance your business in ways that can make you unstoppable.
Why Enterprise Search will be a key proving ground for AI & LLMs. Commentary by Jeff Evernham, VP of Product Strategy at Sinequa
There is huge potential for AI to revolutionize the workplace, making it easier for businesses to manage their knowledge, save time and improve efficiency. Generative Large Language Models (GLLMs) have many applications outside of searching for information – including drafting written content, debugging code, or powering creative applications. However, search stands to reap significant benefits from GLLMs, which add convenience by synthesizing search results into an easy-to-read summary for users. But even more important is how other kinds of LLMs are improving search by understanding language better than ever before. Adding LLMs to search makes finding knowledge faster, more focused, and more forgiving. This is important because search is at the core of almost everything we do, and it is the crux of efficiency and productivity in the enterprise. As LLMs continue to advance, we’ll see the quality of our search tools rise, and many new applications for LLMs to help augment our human abilities so that we are better informed and more effective. However, while the new generative AI bots are exciting concepts, there are still limitations that are yet to be addressed by the industry –most significantly, the accuracy of these models. Both Google and Microsoft’s launch events included factual errors, highlighting just how prevalent so-called ‘hallucinations’ are with generative AI. These companies are racing to rein in these tendencies as they go to market, but until these legitimate concerns about accuracy are resolved, enterprises will be cautious about applying generative AI to their business.
Sign up for the free insideAI News newsletter.
Join us on Twitter: https://twitter.com/InsideBigData1
Join us on LinkedIn: https://www.linkedin.com/company/insidebigdata/
Join us on Facebook: https://www.facebook.com/insideAI NewsNOW
Speak Your Mind