There is a reason the enterprise doesn’t build their own cloud computing infrastructure. Last decade, IT infrastructure teams sought to build their own private clouds because they thought they could do it cheaper and better suited to their business versus public cloud. Instead, they ended up taking longer and costing more than expected to build, requiring more resources to maintain, and having less of the latest capabilities in security and scaling than what was provided by the public clouds. Instead of investing in core business capabilities, these enterprises ended up investing significant time and headcount to infrastructure that couldn’t match expanded business needs.
Many enterprises are now repeating that same do-it-yourself approach to most things MLOps by creating custom solutions cobbled together from various open source tools like Apache Spark.
These often result in model deployments taking weeks or even months per model, inefficient runtimes (as measured by inferences run over compute and time required), and especially lack the observability needed to test and monitor the ongoing accuracy of models over time. These approaches are too bespoke to provide scalable, repeatable processes to multiple use cases in different parts of the enterprise.
The case of the misdiagnosed problem
In addition, conversations with line of business leaders and chief data and analytics officers have taught us that organizations keep hiring more data scientists but aren’t seeing the return. As we delved deeper, however, and started asking questions to identify the blockers to their AI, they quickly realized their bottleneck was actually at the last mile – deploying the models to use against live data, running them efficiently so the compute costs didn’t outweigh the gains, and then measuring their performance.
Data scientists excel at turning data into models that help solve business problems and make business decisions. But the expertise and skills required to build great models aren’t the same skills needed to push those models in the real world with production-ready code, and then monitor and update on an ongoing basis.
This is where ML engineers come in. ML engineers are responsible for integrating tools and frameworks together to ensure the data, data pipelines, and key infrastructure are working cohesively to productionize ML models at scale (see our more in-depth breakdown comparing the roles of data scientists versus ML engineers available here).
So now what? Hire more ML engineers?
But even with the best ML engineers, enterprises face two major problems to scaling AI:
- The inability to hire ML engineers fast enough: Demand for ML engineers has become intense, with job openings for ML engineers growing 30x faster than IT services as a whole. Instead of waiting months or even years to fill these roles, MLOps teams need to find a way to support more ML models and use cases without a linear increase in ML engineering headcount. But this brings the second bottleneck…
- The lack of a repeatable, scalable process for deploying models no matter where or how a model was built: The reality of the modern enterprise data ecosystem is that different business units use different data platforms based on the data and tech requirements for their use cases (for example, the product team might need to support streaming data whereas finance needs a simple querying interface for non-technical users). Additionally, data science is a function often dispersed into the business units themselves rather than a centralized practice. Each of these different data science teams in turn usually have their own preferred model training framework based on the use cases they are solving for, meaning a one-size-fits-all training framework for the entire enterprise may not be tenable.
How to get the most value from AI
Enterprises have poured billions of dollars into AI based on promises around increased automation, personalizing the customer experience at scale, or delivering more accurate and granular predictions. But so far there has been a massive gap between AI promises and outcomes, with only about 10% of AI investments yielding significant ROI.
In the end, to solve the MLOps problem, Chief Data & Analytics officers need to build the capabilities around data science that are core to the business, but invest in technologies that automate the rest of MLOps. Yes, this is the common “build vs. buy” dilemma, but this time the right way to measure isn’t solely OpEx costs, but in how quickly and effectively your AI investments are permeating throughout the enterprise, whether generating new revenues through better products and customer segments or cutting costs through greater automation and decreased waste.
About the Author
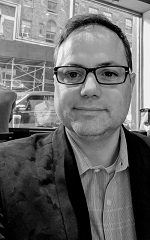
Aaron Friedman is VP of Operations at Wallaroo.ai. He has a dynamic background in scaling companies and divisions, including IT Outsourcing at Verizon, Head of Operations for Lowes.com and JetBlue, Head of Global Business Development at Qubole, and growing and selling two system integration companies.
Sign up for the free insideAI News newsletter.
Join us on Twitter: @InsideBigData1 – https://twitter.com/InsideBigData1
Speak Your Mind