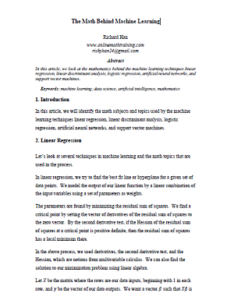
Machine learning is a wildly popular field of technology that is being used by data scientists around the globe. Mastering machine learning can be achieved via many avenues of study, but one arguably necessary ingredient to success is a fundamental understanding of the mathematics behind the algorithms. Some data scientists-in-training often try to take a shortcut and bypass the math, but that route is shortsighted. In order to get the most out of machine learning, you really need that important perspective for what the algorithm is really doing behind the scenes. This perspective is only available with the math. Ever wonder what those tuning parameters actually do? The math has all the answers.
What math subjects are used in machine learning, and how are they used? In this research paper authored by Richard Han, Ph.D., we look at the mathematics behind the machine learning techniques linear regression, linear discriminant analysis, logistic regression, artificial neural networks, and support vector machines.
All information that you supply is protected by our privacy policy. By submitting your information you agree to our Terms of Use.
* All fields required.