As data sets become larger and more complex, organizations are scrambling to create new systems to store, analyze, and pull value from them. This has created a hodgepodge of solutions that accomplish certain tasks, but fall short in other areas. What is needed is a new approach that picks up where today’s solutions leave off.
The latest entry to the lexicon is the data lakehouse. This new destination combines features from two architectures familiar to anybody who’s worked in the data business – data lakes and data warehouses. Advocates say a lakehouse combines the flexibility and low cost of a data lake with the ease of access, familiarity, and support for enterprise analytics capabilities found in data warehouses. Some argue that it helps organizations unchain and expand use of data in warehouses and keeps data lakes from turning into swamps.
Given that the two input components – the data warehouse and the data lake – have their benefits and shortcomings, the question is really how does blending the best capabilities of each component in the process address tomorrow’s challenges?
The components and where the process falls short
Data lakes store data in its raw state. They’ve tended to keep data, in its original forms (structured, unstructured, image files, PDFs, databases), that hasn’t been used but may be considered for operational use in the future.
Data warehouses store fundamental, core data that runs the business, such as customer records and supply chain bills of materials. Raw data must be processed against a schema to fit into the data warehouse before its ever queried and analyzed. In both cases, to prepare the data for use, some sort of data hub technology is required.
Data hubs are gateways through which data can be merged, transformed, and enriched to move it to another destination. IT data integration specialists use them to create integrations from across the enterprise where none naturally existed. Data hubs complement data warehouses, data lakes, and, by extension, data lakehouses, as they support orchestrated, stateful use of data against the data model at each stage of the data pipeline.
Analytics hubs help channel data to a broader set of users – to analysts and data scientists as well as to citizen or power user analogs of these two roles. They operate like point-to-point switches. They depend on an external source – a cloud data warehouse, data lake or data lakehouse – to store input data.
Unlike the prior three terms, you don’t hear the term ‘analytics hub’ much because they really aren’t hubs at all. They don’t support the curation of data in stored views from multiple projects for long-term use. Rather, they specialize in enabling the execution of a series of analytics projects by non-IT users, including analysts, data scientists, and citizen developers with BI and reporting tool skillsets.
Without the ability to easily get data from multiple data sources and tie composite elements of data from those various sources for presentation out to the analytics tools, you don’t really have an analytics hub, chiefly because you don’t have a data hub. However, they are great for non-IT users who are really focused on the analytics and rely on IT to give them the access and support for the data they need. Increasingly, with overburdened IT and far more diversity and change to datasets, this reliance is a major roadblock.
Broken promises
While all four solutions serve certain functions, none can operate without significant support from IT. In other words, the idea of a single shared virtual repository of data – accessible by a multi-disciplinary set of users and tied to their preferred analytics tools of choice – promised by data lakes, data warehouses and data hubs doesn’t exist. The industry hasn’t created an actual analytics hub that serves all of the stakeholders’ needs.
Data lakehouses solve some issues, but not all. They evolve the concept of data lakes, bringing together some features and functionality found in data warehouses while also serving the needs of data science. Data scientists can benefit from ease of use, allowing them to pursue broader sets of queries and experiment with the way data and will be incorporated into process automation and orchestration. But, as data use increases, so does the need for functionality inside a data hub and a data warehouse.
The real solution – particularly given the rapid cycle of continuous development and continuous improvement – is to blend elements from all of these technologies into one.
Introducing the data analytics hub
Rather than create siloes – data hubs for IT and analytics hubs for non-IT users – organizations need a new vehicle that brings together all the elements in a way that data lakehouses promise. This can be defined as a “data analytics hub.” Data analytics hubs can be used by a broad array of IT and business across multiple datasets. They can draw elements from the four technologies: data hubs, analytics hubs, data lakes, and data warehouses.
Like a data hub, a data analytics hub connects to different data sources. But unlike a data hub, it provides persistence in a cloud repository. It also allows for the curation of diverse data types that can be ingested in both batch and streaming modes with self-service, low-to-zero-code options through drop-down menus for non-IT users.
Like a data lake, a data analytics hub’s cloud storage repository can handle all data types and leverage industry standards for data movement and analysis. However, unlike today’s data lakes, data analytics hubs also support end-user facing business intelligence (BI) and advanced analytics workloads through use of SQL. It could be described as a bi-directional hub, supporting multiple inputs and outputs, solving for all permutations of input data and output tools used by a diverse set of non-IT users.
Data analytics hubs support most popular BI, reporting, visualization, and advanced analytics tools. However, unlike today’s data hubs, data lakes and data warehouses, data analytics hubs provide user-friendly self-service tools that enable non-technical users to link any data source to any end-user tool — without the need for IT intervention, on either a one-off or day-to-day basis.
In short, a data analytics hub gives organizations what they need in today’s evolving data-centric environment – the ability to store, manage and analyze data on a holistic basis by diverse virtual teams. It combines the critical data collection and analytical features of these well-known solutions but exposes all those features in ways that key business users can access easily and incorporate into programs and processes.
About the Author
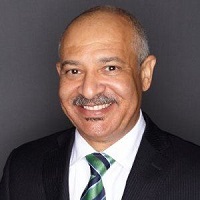
Lewis Carr is Senior Director of Product Marketing at Actian. In his role, Lewis leads product management, marketing and solutions strategies and execution. Lewis has extensive experience in Cloud, Big Data Analytics, IoT, Mobility and Security, as well as a background in original content development and diverse team management. He is an individual contributor and manager in engineering, pre-sales, business development, and most areas of marketing targeted at Enterprise, Government, OEM, and embedded marketplaces.
Sign up for the free insideAI News newsletter.
Join us on Twitter: @InsideBigData1 – https://twitter.com/InsideBigData1
Speak Your Mind