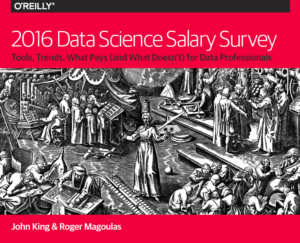
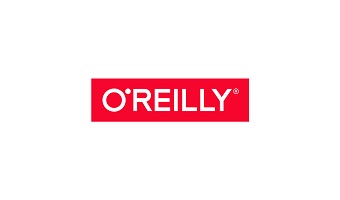
IN THIS FOURTH EDITION of the O’Reilly Data Science Salary Survey, the input was analyzed from 983 respondents working in the data space, across a variety of industries— representing 45 countries and 45 US states. Through the results of a 64-question survey, the survey explores which tools data scientists, analysts, and engineers use, which tasks they engage in, and of course—how much they make.
Key findings include:
- Python and Spark are among the tools that contribute most to salary.
- Among those who code, the highest earners are the ones who code the most.
- SQL, Excel, R and Python are the most commonly used tools.
- Those who attend more meetings, earn more.
- Women make less than men, for doing the same thing.
- Country and US state GDP serves as a decent proxy for geographic salary variation (not as a direct estimate, but as an additional input for a model).
- The most salient division between tool and tasks usage is between those who mostly use Excel, SQL, and a small number of closed source tools—and those who use more open source tools and spend more time coding.
- R is used across this division: even people who don’t code much or use many open source tools, use R.
- A secondary division emerges among the coding half— separating a younger, Python-heavy data scientist/analyst group, from a more experienced data scientist/engineer cohort that tends to use a high number of tools and earns the highest salaries.
All information that you supply is protected by our privacy policy. By submitting your information you agree to our Terms of Use.
* All fields required.