It may not sound like news to say that fraudsters are becoming more sophisticated, but as fraud attacks constantly evolve to evade detection (and to “cash in” on consumer-facing online services and applications), it’s clear that traditional solutions are struggling to keep up.
Conventional machine learning fraud solutions rely on historical examples of attacks for model training. Given labels of what is and is not fraud, the model learns to differentiate the two to identify fraud. However, these solutions are limited to known, previously seen attacks, and cannot generalize to new evolving threats.
Fraudsters are becoming increasingly aware of behaviors that can trigger machine learning fraud detection systems. They have learned to orchestrate the fraudulent accounts in such a way that they blend in with normal user activities. In addition, useful “tools” such as device emulators, temporary email services, virtual phone numbers and IP proxies enable fraudsters to change up their attack operation easily and quickly, evading rules or reputation-based solutions.
Fraud Attacks have a Broad Spectrum
Fraud attacks follow a broad spectrum of characteristics, varying in size, duration and sophistication, depending on the intended outcome of the fraudulent activity. The sophistication of an attack takes on many dimensions: how fraudsters obtained access to the online service or platform, how they orchestrate fake/compromised accounts to evade detection, and how they scale the attack operation to be profitable.
Recent industry research takes a deep dive into the diverse spectrum of modern fraud attacks. Highly sophisticated attacks may be 2.3 times larger than their low sophistication counterparts, with the potential to cause considerable damage to an enterprise and its users.
Sophisticated attacks are particularly prevalent in the financial markets, where some 56% of attacks show high levels of sophistication. These sophisticated cases of fraud are exemplified by the creation of large numbers of fake user accounts that behave like normal. Each account may be associated with a different email address and domain, and each login from a different device or location. Without a closer inspection, they can be passed off as a normal set of isolated users and operate stealthily under the radar.
Fraud Attacks have a High Churn
Fraudsters have become good at evading static signals, and have a flexible back-end infrastructure. Among recently observed fraud signals in the last quarter, 36% were active for less than one day, and 64% were active for less than one week. Solutions that rely on historical attack information would have limited effectiveness and decay quickly, requiring constant adjustment.
In addition to high churn in fraud signals, fraudulent accounts also take extra steps to blend in with other normal users. One tactic is to allow credit accounts, for example, to build up solid ratings and increased credit limits over a few months – and then in a single activity request huge cash advances and vanish into the night, having gone undetected until the damage was done.
As another example, some fraudsters have began leveraging peer-to-peer community VPNs with residential and mobile IP ranges as IP proxies. In contrast to cloud hosting services like AWS or DigitalOcean, these IP ranges are used by hundreds of thousands of benign users.This strategy makes it difficult for machine learning systems to differentiate potentially fraudulent accounts from normal activity.
The Need for More Elaborate Detection Methods
Because attacks happen on a spectrum, businesses have to know what’s happening on their platform first, to make an educated choice about the types of solution to implement.
There is no “plug and play” solution to solve the problem of fraud detection. To deal with sophisticated, fast-evolving online attacks, a robust solution should incorporate multiple layers of defenses as well as the capability to adapt dynamically to new threats. Effective solutions must also mesh with industry domain knowledge. Standalone machine learning tools or point solutions cannot solve the business problem from day one.
Don’t sit by thinking that the old methods of fraud detection are sufficient for your organization. Fraud is becoming ever more sophisticated. If you are just keeping up, you are already behind.
About the Author
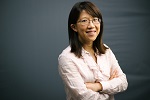
Ting-Fang Yen is a Director of Research at DataVisor, a company providing big data security analytics for online services and financial institutions. Her work focuses on network and information security data analysis, where she combines data science with security domain expertise to develop practical technologies and solutions. Her research has shaped product directions and published at top industry and academic security conferences. Ting-Fang received a PhD degree in Electrical and Computer Engineering from Carnegie Mellon University, Pittsburgh, PA.
Sign up for the free insideAI News newsletter.
Speak Your Mind