A major, accelerating shift is underway in retail. As consumer spending behaviors rapidly move online, traditional and online retail outlets are in a heated arms race to adopt and develop deep learning technologies in order to maximize engagement, increase sales and ultimately, drive revenue.
Recently, conversations about AI have dominated the tech scene with people like Mark Zuckerberg, and nearly every major tech company founder, participating in the debate. Everyone agrees that AI will transform our lives. From an advertising point of view, global giants, such as Google and Microsoft, have already deployed new AI technologies inside their ad systems and they are projected to dramatically boost ad revenue.
What is Deep Learning?
Deep Learning, an advanced form of artificial intelligence (A.I.), is all around us, and it’s growing increasingly ingrained in how we live and work. Deep Learning tech is “the brains” behind the automated traffic control on our city streets, our sophisticated translation systems, and the fast, accurate facial recognition at our airports. It’s also an integral technology for any breed of self-driving car. Additionally, Deep Learning can also vastly improve both the shopping and e-commerce experience.
How is Deep Learning being used in e-commerce?
Some practical examples of the predictive power of Deep Learning in action include the ability to increase online ad response without increasing your cost of sale or decreasing your ROAS, delivery of more personalized offers for shoppers based on browsing history or habits, or instant, deep insights about a particular consumer based on their recent purchases or shopping behaviors.
What companies are implementing Deep Learning in eCommerce and how it is being applied?
Wish.com: Using Deep Learning to Only Show Users What They are Interested in
The Wish app uses data mining and deep learning to show users (who are ultimately shoppers) products they are likely to buy, save, or share. Showing just a handful of products at once, Wish is benefiting both buyers and sellers. For the buyers, Wish eliminates clutter. It doesn’t show shirts, pants, skirts, or belts — as a few examples — that are not apt to interest the shopper. The shopping experience is streamlined. For merchants, this means that products are presented to the most likely buyers, potentially encouraging sales. For small and mid-sized online sellers, Wish’s popularity — perhaps half a million daily users — may demonstrate how personalizing pleases customers and ultimately may increase sales.
Wish employs big data principles, deep learning, and state-of-the-art search technologies to create a highly-visual, entertaining, and personalized browsing experience for each user.
“The more we invest in data to personalize the recommendation experience, the more we see improvement in conversion rates,” according to Peter Szulczewski, CEO and Co-Founder.
RTB House: Transforming Advertising Engagement Through Deep Learning
RTB House is another example of innovators exclusively using Deep Learning and proof that what seemed like sci-fi just a few years ago seems now to be a natural process to make online activities more efficient than ever before.
The driving force of RTB House is its disruptive performance marketing AI platform built on 100 percent Deep Learning and what it delivers for marketers. Its self-learning algorithms, inspired by biological neurons in the human brain, analyze and learn internet users’ behaviors in real time to generate outstanding results. Its platform identifies high-value shoppers and boosts results via one-to-one personalized ad creatives. Due to Deep Learning algorithms, performance is up to 50 percent more efficient than standard machine learning AI-based approaches.
“Deep Learning is even more advanced than machine learning, and can handle much larger quantities of data very quickly for common retail applications,” said Gary Burtka, VP of US Operations at RTB House. “But Deep Learning, a form of AI that mimics the workings of the human brain, has important implications for retailers. And some already are putting it to work.”
Additional Examples of Deep Learning Transforming the Retail Sector:
- Optimizing Post Sales Support – Macy’s is piloting chatbot-guided shopper assistance that uses natural language processing technology co-developed by IBM Watson and Satisfi Labs. Other retailers have rolled out more operational-focused uses such as how Amazon and Walmart use A.I. to optimize their supply chains, delivery routes or checkouts.
- Personalizing Sales Engagement – One of the first tasks assigned to Andrew Pole, a statistician and predictive analytics expert retained by Target, was a better understanding of new parents, who are invaluable customers for the big-box retail chain. Pole focused on studying pregnant women in their second trimester to capture their brand loyalty for years to come. By analyzing shopping habits, they were able to create a rather accurate “pregnancy prediction” score, optimize Target’s supply chain and boost the personalization of customer offers. Since that early pilot that back in 2002, Target constantly analyzes a vast trove of data in order to make shopping more fun and relevant for its guests. Its tens of millions of shoppers lead to billions of transactions and interactions that cannot be put to use without the power of A.I.
- Visual Search and Building New Online Experiences – Wayfair launched a visual search tool that lets customers take photos of products they like and find visually similar ones on its website or mobile app. Those search requests can be tricky when shoppers are asking to make a room more “bohemian” vs. “nautical” vs. “minimalist” via search terms they input. But advanced algorithms and computer vision make it possible for Wayfair to deliver just that.
About the Author
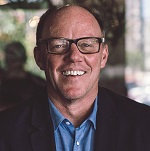
Garth Holsinger, founder of Enterprise Alpha, is a consultant focused on helping emerging technology startups connect to the F500. He is a Partner at Prota Ventures, an operator-investor group that incubates new startups and products, and invests in early-stage ventures. He was Sr. EIR at Bionic, a fast-growing consulting firm helping F500 enterprise clients grow and launch new ventures. He has held key positions at fast-growing, Silicon Valley startups including Klout and Livefyre, where he led growth and corporate partnerships. He lives in both the Nevada desert and in Brooklyn, New York.
Sign up for the free insideAI News newsletter.
Speak Your Mind