It’s seemingly a weekly occurrence that a business suffers a major cyberattack and makes headlines. Companies are more challenged than ever to keep their business secure. As a result, 71% anticipate their cybersecurity budgets to increase in the next three years, despite investments in other IT segments decreasing.
Using AI in cybersecurity tooling is not new. We are still in the early stages of seeing the benefit that AI can bring to the levels of protection offered. In the future, AI will be instrumental in keeping businesses protected from known and evolving cybersecurity attacks.
Businesses face two challenges that AI is better suited to handling than traditional approaches:
- Detecting emerging attack types, including zero-day attacks.
- Adding intelligent filtering to very large datasets before they are handed over for human analysis.
Securing the business using predictive tools
By design, AI is good at learning what “normal” behavior looks like, detecting anomalies and spotting new types of activities. In a security setting when something unusual happens, that event can be reported as a potential attack. This generally works fine when the type of attack is expected – in other words, a known approach used by cybercriminals. Businesses learn more about attacks after they happen, including the type of attack and how it occurred. As a result, they fortify their systems to prevent it from happening again. This is where traditional security tooling is useful as it looks at a prescribed set of rules for what is and isn’t allowed.
But what happens if something falls outside those parameters? This is AI’s sweet spot.
Let’s say, for example, people are only allowed to enter the house through the front door. Anyone who enters in the expected way – walk up to the door, put a key in the lock, and open the door – is legitimate. An attacker, however, will likely undertake a different series of actions. These actions could include approaching the door and getting a key out the same as other lawful entrants. However, if that attacker also looks through the window to see if anyone is home or first tries the door to see if it’s unlocked, this combination of behavior – even though each behavior is legitimate – could signify an attack. This pattern of actions might not seem suspicious to a passerby but is recognized by AI as unusual behavior.
Businesses that can respond to new threats as they occur improve efficiency and lower risk. AI tools have the capacity to step in and increase detection rates, especially when combined with traditional measures. In other words, AI allows you to broaden what is a potential risk and stay ahead of the cybercriminals.
Companies can also use AI as part of the threat hunting process. They can process high volumes of endpoint data to develop a profile of each application within an organization. Using behavioral analysis and other machine learning techniques, threats can be identified faster when they breach a system.
Amongst the security threats that companies must contend with today are bots. Automated bots make up a large amount of internet traffic and can be used for attacks such as stealing data, taking over accounts using stolen credentials, creating fake accounts and committing other types of fraud.
Businesses can’t fight these automated threats solely with human responses. The quantity is too vast and the behavior too sophisticated. The only effective strategy is to fight fire with fire, which is where AI can help. By looking at behavioral patterns, a company can differentiate between good bots (such as search engine scrapers), bad bots and real humans. This also allows them to develop a comprehensive understanding of their website traffic, so they stay one step ahead of the bad bot threat.
For example, a global gaming and betting site had a problem with bots continually scraping its site for odds. Arbitrage bettors used this information to place bets on every outcome of specific events to guarantee a profit. Not only does this cheat the system but the bots then represent a large portion of the site’s overall traffic, adding to infrastructure costs. Consistently identifying the scraping behavior in real-time involved a huge amount of unstructured data that was hard to classify across websites and threat levels. A team of data scientists determined that only by looking at a request-by-request level of website interactions did the intent of an individual’s behavior become apparent. Machines alone struggled to identify behaviors like scraping at scale and in real-time but combined with the right human interaction and analysis they were able to combat the situation.
Processing and analyzing large amounts of data and integrating human processes
Humans are good at detecting when items are similar but not identical. So is AI. AI has an advantage, though. It can apply human-like analytical decision-making to large amounts of data and then rule out alerts that it has learned as no/low risk, or group similar warnings to identify an underlying issue.
Learning how humans have previously responded can allow AI systems to understand patterns of behavior and make appropriate recommendations on actions to take. The best solution is a combination of human intuition and deductive intelligence augmented by AI, for example, a human doctor supported by AI analysis of patient data is better than either operating alone.
This capability isn’t easy to develop. It requires an understanding of the scope of the problem, the data that can be obtained, and the power of applying AI – a combination of attributes that require a significant investment in time and expertise to produce reliable results. Those results then need to be regularly tested, validated, optimized, and improved. The challenge with validating results, however, is that hackers try and hide their activities, so determining the level of threat with any degree of accuracy requires some assessment, rather than a hard and fast yes or no.
Introducing AI into security is not a silver bullet but it will play an essential role in good cybersecurity practices. It won’t replace existing approaches but will augment them and form the basis of emerging tooling. Doing so will not be easy – taking full advantage of AI will take time and investment to create tools that solve these issues.
However, over time, there will be steady growth, and the value-add will become increasingly more evident. AI will also not replace human involvement; it will just supplement it and allow people to focus on the areas that add the most value, such as making decisions from the data and learnings that AI provides.
About the Author
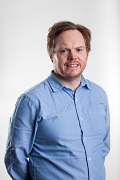
Andy Still is Chief Technology Officer and Co-Founder of Netacea where he leads the technical direction for the company’s products and provides consultancy and thought leadership to clients. He is a pioneer of digital performance for online systems, having authored several books on computing and web performance, application development and non-human web traffic.
Sign up for the free insideAI News newsletter.
Join us on Twitter: @InsideBigData1 – https://twitter.com/InsideBigData1
Speak Your Mind