Raw data, the fundamental building block of accurate web analytics, remains an underutilized asset in many organizations that opt for sampled data due to its simplicity and ease of management. This article explores the benefits and challenges of using raw data in analytics, and discusses its potential use cases for gaining valuable insights.
What is raw data?
Raw data is unstructured and unformatted data an organization gathers from various sources: databases, files, social media, web pages, images, etc.
Because raw data is not filtered or processed, it provides a complete view of information. It allows for in-depth analysis and accurate insights but also may be large and difficult to handle. With proper tools, raw data provides more possibilities for exploring data insights and making them useful.
Key differences between raw data, processed data and sampled data
Raw data typically comes in its most basic and unorganized form, representing the original observations, measurements, or responses. Raw data provides a complete and comprehensive dataset, allowing for thorough analysis. It enables filters and visualizations to be applied to derive new insights and perspectives. In addition, it offers the flexibility to revisit and reanalyze the data for different reporting needs. On the downside, it requires significant time and effort to process and transform into actionable insights, and handling large datasets or using APIs for raw data extraction is more resource-intensive, particularly in advanced analytics.
On the other hand, processed data refers to the transformed or analyzed form of raw data. Processed data, through data transformation and analysis, is more interpretable, making it easier to identify patterns, trends, or relationships. It is more organized than raw data but tends to be condensed or summarized, so may lack detail. You can find processed data in analytics reports, among others.
Sampled data represents only a selected subset of a larger dataset. Data sampling offers a manageable yet representative snapshot of the whole. It comes in handy in scenarios where speed and resource efficiency are prioritized.
However, it may not accurately represent the bigger picture given by the full data set, potentially leading to less precise results. Although it might not provide the details of raw data, sampled data is structured and tailored for advanced analysis, enabling analytics optimization and timely insights.
These inherent differences between data types entail certain advantages and disadvantages and define unique use cases for each type of data.
Using raw data in analytics
The primary value in data is after it has been processed and interpreted. There is little value in holding onto raw data without a way to use it. Raw data unlocks the door to more sophisticated and precise analytics, enabling a better understanding of information that often remains untapped in summarized data formats.
One practical benefit of using raw data in web analytics is that it can be exported from analytics platforms using other tools, paving the way for more extensive and insightful analyses. This approach caters to the technical complexities demanded by professionals in the field. It also remains accessible to those with less technical backgrounds, ensuring a balanced understanding across different levels of expertise.
The usual workflow when working with raw data involves:
- Identifying raw data sources – Before choosing the right analytics tool, it’s important to determine where the data will come from. This includes internal sources (like CRM systems, sales data, etc.) and external sources (market research, social media, etc.).
- Raw data collection and integration – Gathering data from the identified sources and integrating it for further use.
- Data cleaning and preparation – This involves sorting, cleaning, and organizing the raw data to make it suitable for analysis or visualization.
- Exporting data to other tools – Data exports can broaden the scope of analysis and provide more detailed insights.
- Data analysis – Using various data analysis techniques to uncover patterns, trends, and insights. This can include statistical analysis, predictive modeling, data visualization, and more.
- Interpreting the results – Understanding what the data says and connecting it with business goals.
Raw data benefits and use cases
Raw data is beneficial because it’s highly relevant, specific to the research being done, and provides fresh information. This makes it suitable for supporting data-driven decisions.
As it gives preliminary visibility to the dataset, it offers more freedom in data transformation. On top of that, raw data gives a backup to refer to when encountering problems after processing and analyzing your data.
Tracking complete customer journeys
Raw data from multiple sources can be used to track the complete customer journey across different platforms. This includes online and offline data and data from different platforms like a website and mobile app.
This type of data represents the unaltered voices and behaviors of consumers. Whether it’s the transcript of a focus group discussion or the record of online purchases during a holiday sale, raw data captures the market in its most natural state. By connecting these data points, organizations can understand how users move between different platforms and how their campaigns lead to conversions.
Attribution modeling
Organizations can use raw data for attribution modeling, either by doing it themselves or by hiring agencies that use algorithms. Attribution modeling involves using data analysis and statistical modeling techniques to determine the contribution of each marketing touchpoint in driving conversions or sales.
Custom dashboards
Unlike processed or summarized data, raw data hasn’t been subjected to any interpretation or altered by any tool. This means businesses can approach it with different analytical tools and derive various insights based on changing needs.
For example, raw data can be used to create insights dashboards in BI tools or companies’ apps for internal or external needs. Or, agencies might compile reports for their clients using raw data. These dashboards can help organizations visualize their data and extract meaningful insights.
Conclusion
While data sampling can be useful for efficiency and performance, raw data is still indispensable in specific contexts. It can lead to more accurate and insightful decisions by providing precision and depth. Both sampled and raw data have their place in data analytics. They can significantly benefit decision-making processes and play a vital role in an organization’s sustainable growth and success.
However, in the end, precise data fuels precise decisions. Raw data is a viable source for a sustainable future for your organization.
About the Author
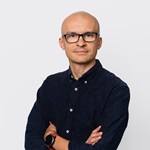
Mateusz Krempa is the Chief Operations Officer for Piwik PRO. Piwik PRO Analytics Suite, is a platform empowering organizations to analyze and streamline customer journeys. Krempa has worked in a variety of roles since 2014. Prior to joining Piwik PRO in 2017, he worked as a Project Manager at Exlabs Software Ltd. At Piwik PRO he has held the roles of Chief Revenue Officer, Head Of Operations, and Product Manager before moving to the Chief Operations Officer role. Krempa attended the SGH Warsaw School of Economics from 2010 to 2013, where he earned a Bachelor’s degree in Quantitative Methods in Economics and Information Systems. Krempa later returned to the same school from 2014 to 2016, where he earned a Master’s degree in Accounting and Finance.
Sign up for the free insideAI News newsletter.
Join us on Twitter: https://twitter.com/InsideBigData1
Join us on LinkedIn: https://www.linkedin.com/company/insidebigdata/
Join us on Facebook: https://www.facebook.com/insideAI NewsNOW
Speak Your Mind