Modern businesses generate vast amounts of data, which is ultimately distributed across various cloud databases, local apps, on-prem servers, and the edge. Disconnected, disorganized and siloed data is a liability and represents lost opportunities to glean both strategic intelligence for leaders, and insights for real-time decisions. The opportunity is more pressing as companies race to implement analytics, machine learning (ML) and artificial intelligence (AI) across their enterprise.
The ideal solution to siloed data is implementing a single data plane across a business. This unified system allows enterprises to realize the grand vision they have been promised: One where data from all sources and apps can be used together for the benefit of the business. Understanding the role of cloud, mesh and fabric architectures is the first step toward a truly contemporary data-driven enterprise.
Data in Cloud
Th data in cloud approach provides flexible storage at scale. The back end is managed by a cloud provider, and access is controlled on the cloud, available to anyone with an internet connection and credentials. Data clouds can be cost-effective depending on the quantity of data and the types of workloads, which might include databases, analytics, and web or content hosting. They are also generally safe since cloud-scale providers have high levels of redundancy and security.
Data clouds offer scalability, flexibility and agility for data processing and storage. They are less valuable for data-intensive workloads, particularly analytics. The cloud also comes with challenges such as increasing expenses at scale: a 2022 KPMG Technology Survey found that 66 percent of business executives said cloud programs did not lower their IT ownership costs.
Data clouds can also suffer from performance issues which impact business performance: Google data shows that consumers will abandon sites or apps as load time increases, even if only from one second to three seconds.
The main weakness for data clouds is that most enterprise’s data will never reside on one cloud. A more holistic, hybrid multicloud approach to data management is a requirement. Companies should also watch for hidden costs around data control, compliance, and governance and the cost of rebalancing workloads away from a data cloud.
Data Mesh
The goal of a data mesh is to unite data sources, wherever they are, under centrally managed sharing and governance. Mesh is a complex architecture, but effective for improving data access control and security.
A data mesh is typically good for very structured data, such as when many databases must interconnect and communicate with each other. Mesh enables data integration, consistency, and quality across different systems. It also requires complex coordination, governance, and maintenance.
Financial service businesses often apply data mesh to interlink lines of business so structured data can be used across business functions (customer service, marketing, and so on) and still be managed securely.
Data Fabric
The fabric model is the most flexible and the right data fabric helps organizations leverage the tools they already use to accelerate their AI, analytics, and digital modernization journeys.
With data siloed across hybrid and multicloud, data fabrics can operate as a fabric of fabrics, bringing together multiple data fabrics and underlying data stores, while abstracting physical location. The data fabric model provides data management across varied environments in the public cloud, colocation, private cloud and on-premises, and seamless data management (in theory) across a choice of endpoints. With so much emphasis today on AI and ML, data fabrics are one of the clearest means for capitalizing on these workloads, particularly those that mix large volumes of structured and unstructured data across objects, streams, videos and more.
Data fabrics provide a unified layer of abstraction over disparate data sources. This can be used in multiple ways: batch, streaming, or interactive. Data fabrics also enable self-service access, discovery, and governance. Having a single source of truth for everyone in the organization is important. It means that everyone, from data scientists to developers, can work from the same single source of standardized, relevant data and can make informed decisions using the same data sets. That’s a far cry from having disparate groups working from data sets sitting in silos and departments operating as separate entities. With the right guardrails around geofencing and governance, there are no hidden costs. The cost of the fabric is the true cost.
A modern architecture
My advice for CTOs and CIOs is this: explore everything and tune out the buzzwords. Let workloads and use cases guide the decision-making process but stay alert for hidden costs and architecture limitations. Consider how much your enterprise’s data will grow over time, and the sources of that data. With emerging data sources, sovereignty regulations and use cases, an open extensible interface that can adopt new frameworks for monitoring, observability and explainability will make it possible for your architecture to adapt as workloads evolve.
If your organization, like most, expects its data volume to expand significantly, and if you need to manage structured and unstructured data (files, objects, and databases), fabrics that utilize open-source tools offer valuable flexibility, reduce vendor lock-in and are the most future proof.
About the Author
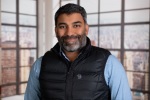
Mohan Rajagopalan is a vice president and general manager at Hewlett Packard Enterprise, where he oversees the strategic direction and growth of the HPE Ezmeral Software business. Mohan joined HPE from Splunk where he built out and led the AI/ML and next-gen analytics product areas. Before Splunk, he started and led two companies focused on bringing advanced analytics and data science into the enterprise data stack. Mohan has a Ph.D. in Computer Science and combines a deeply technical background with a passion to bring new technology to market.
Sign up for the free insideAI News newsletter.
Join us on Twitter: https://twitter.com/InsideBigData1
Join us on LinkedIn: https://www.linkedin.com/company/insidebigdata/
Join us on Facebook: https://www.facebook.com/insideAI NewsNOW
I would differ on this statement – “The goal of a data mesh is to unite data sources” – Data Mesh is a decentralized approach for Data and Organizational Management
I stopped reading after this statement