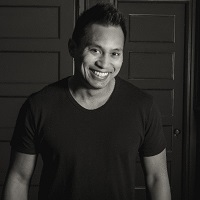
In this special guest feature, Chris Santiago, Vice President/Solutions Engineering, Unravel Data, talks about controlling cloud spend through three phases of the FinOps lifecycle. Chris is a seasoned data executive with more than 15 years experience. Currently, he is the VP of Solutions Engineering at Unravel Data, where he helps enterprises maximize their investments in data applications and infrastructure.
Data cloud costs are mushrooming out of control. While modern data stacks have allowed for outstanding innovations across verticals, for many organizations, it’s come at a steep price—literally and figuratively. Companies are getting ambushed by their monthly bills, and with limited visibility into where all the money is going, capacity and budget forecasting has been reduced to a guessing game. No wonder then that organizations are finding their three-year budgets are exhausted a few months into Year 1, and that their cloud migration projects are hitting a wall.
As cloud costs continue to rise—with no apparent end in sight—companies can’t continue to take a “pay it by ear” approach to cloud spend. In fact, Gartner estimates that 60 percent of public cloud customers will experience cost overruns in the coming year, and KPMG found that two-thirds of organizations have yet to achieve substantial ROI from their cloud transformations. To counter ballooning cloud costs, more and more companies are turning to the practice of FinOps as a means of governing their cloud spend and ensuring that what money is being spent is done so in such a way as to maximize returns.
Control costs the FinOps way
Controlling costs consists of three iterative phases across the FinOps lifecycle, each offering unique insights and opportunities to streamline costs:
- Observability: Before organizations can tackle costs, they need fine-grained visibility. Observability gives a holistic view of applications, workloads, users, data, and resources. During this phase, trends and patterns that reveal how the cost of cloud resources changes over time emerge, allowing for the next step in the process.
- Optimization. If the observability phase tells you what’s happening and why, the optimization phase is about using that information to identify where you can eliminate waste, remove inefficiencies, or leverage different cloud compute configurations that are less expensive without sacrificing SLAs.
- Governance. During this phase, teams move from reactive problem-solving to proactive problem-preventing. Guardrails are put in place, which in turn allow for sustained iterative improvements and empower employees to make better choices.
Each phase builds on the former, to create a virtuous cycle of continuous improvement and empowerment for individual team members—regardless of expertise—to make better decisions about their cloud usage while still hitting their SLAs. In essence, shifting the budget left and pulling accountability for controlling costs forward.
When done right, FinOps addresses cloud complexity and helps organizations bring cost and performance efficiency in line. However, simply knowing how much money is being spent and on what doesn’t provide the granular level of detail that organizations need to make sound data-driven decisions about their cloud usage, determine opportunities for optimization, and ultimately realize ROI from their data stack investments. For FinOps to be truly effective, teams need multi-pronged, multifaceted insight into what’s going on in and across their data apps and pipelines.
And that’s where AI-powered DataOps observability comes in.
AI-driven analytics takes observability to 11
DataOps observability captures deep and granular performance and cost details from the various systems in a data stack—horizontally and vertically—and correlates everything into a meaningful “workload-aware” context. Applying AI to these fine-grained details allows data teams to track, visualize, and ultimately allocate resources according to actual usage requirements versus perceived need. FinOps teams can then accurately predict their capacity requirements, implement automated governance guardrails to keep costs under control, and even launch corrective actions automatically.
AI-driven observability provides deeper, more meaningful context throughout the FinOps lifecycle:
- Observability: With a multilayer view into their data stack, teams can track actual spend versus budget, allowing them to know in advance—and in real time—whether a workload, user, or project’s usage is on track, at risk, or dipping into the red. They can identify who the big spenders are, whether it’s a project, team, user, or application. This granular information identifies how many and what size resources are needed to actually run a job versus what’s been allocated, how long optimized jobs actually take to run so data teams can determine whether running on spot instances will save money, and even if they’re auto-scaling efficiently.
- Optimization. Workload-aware optimization allows teams to target cost-saving opportunities that go beyond simply slashing deployed-but-no-longer-in-use resources to pinpoint code inefficiencies, and right-size overprovisioned clusters. AI-driven observability doesn’t just tell you what to fix, but how to fix it.
- Governance. While reducing costs reactively is good, controlling them proactively is better. AI-powered automation not only converts optimization into insights, it also converts analytics into impact, empowering employees to make better choices, and in some cases fixing the problems for them. Without cost governance, controlling costs can be a bit like herding cats, only less effective.
Enabling self-service across the organization frees up more experienced team members to spend less time troubleshooting and more time innovating. It’s that power that frees data teams from the business equivalent of coupon-clipping to do what they do best.
FinOps isn’t just about saving money, it’s about making money. And that’s exactly what AI-driven observability empowers companies to do.
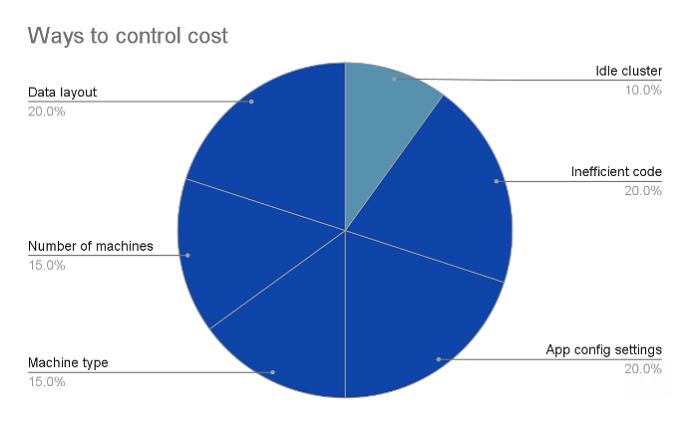
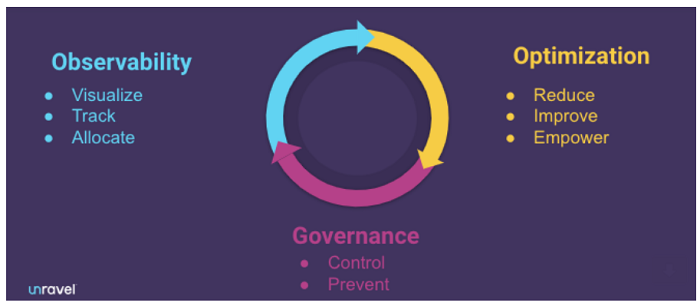
Sign up for the free insideAI News newsletter.
Join us on Twitter: https://twitter.com/InsideBigData1
Join us on LinkedIn: https://www.linkedin.com/company/insidebigdata/
Join us on Facebook: https://www.facebook.com/insideAI NewsNOW
Speak Your Mind