I see it all the time. A company is excited to have a data lake and all the potential it promises. But things quickly go south from there as expectations don’t easily, or quickly, match up with reality. That is where the “hope” in this article’s title comes from, because, in many cases, it is actually the strategy!
The good news is that, today, it doesn’t have to be that way. The data lake has come a long way since arriving on the technology scene 10+ years ago. Back then Hadoop was king of the nascent “Big Data” trend and the challenges and complexities of actually deriving value from massive amounts of data were not well understood. Fortunately, technological innovation has since produced better, faster and more secure ways to make data-driven decisions. I like to tell people that we’re now in the era of the “modern” or “active” data lake.
The Data Lake Story is … Complicated
A data lake is essentially a single repository for all of an organization’s raw unstructured, semi-structured and structured data. Depending on how it is built, the lake performs functions such as data collection, storage, security and analysis. One thing it has not been is a single product. It’s been an approach and created with a toolkit containing a disparate collection of point solutions.
While many organizations have some form of a data lake, many others don’t. Data lake initiatives typically fail, in part, because of immense complexity, a lack of security and governance, and a proliferation of data silos. Even when they don’t fail completely, there are additional tradeoffs with storage capacity, scaling and the expense. In fact, what I typically see with data lakes is more like a data quagmire because most of these solutions can’t effectively catalog, understand or organize all of an organization’s data.
Maximizing Data Lake Utility without Compromise
“So, Christian, how do I maximize the utility of my company’s data lake?” It’s a question I am often asked in some way, shape or form. This is usually followed by more specific inquiries about one or more of the compromises organizations often make to extract value from their data lake(s): performance issues; difficulty managing and scaling; high platform license costs; processing JSON, XML and Avro data; and struggles with increasing solution complexity. And that is just a partial list.
First, I recommend doing something with the data and objects stored in their data lake,. Specifically, they need to create real-time dashboards to report on the data, run fast analytics to uncover insights and relationships, and interactively explore the data to find new trends.
Second, I suggest to organizations they run through what I call a “data lake to-do list” and honestly assess where they are with each topic area:
- Single repository, no silos
- Open formats
- Raw representation
- Multiple workloads
- Cost-efficient storage
- Schema on read
- Governance and security
- Transactional
- Data sharing
Lastly, there is performance management. Depending on the technology approach you take, keeping all this infrastructure running can be overwhelming.
How Organizations have Approached their Data Lake Strategy
1) Hadoop: The most common approach, using a toolkit of open-source solutions that, in most part, failed to deliver the highly coveted but elusive goal of a single and secure analytics repository for all structured, semi-structured and unstructured data.
2) Blob storage (e.g., AWS, Microsoft or Google): The perceived antidote to the failure of Hadoop. A single repository, yes, but lacking the power, speed and efficiencies that solutions such as the data warehouse offer.
3) Modern data warehouse: A powerful, fast and secure cloud-built data warehouse that delivers what the data lake promised along with the instant and near-infinite elasticity the cloud offers.
Smooth Sailing is Still Possible … If you Break Down the Data Barriers Impeding It
Despite the hurdles to corralling all of an organization’s data and turning it into actionable insights, the good news is that just as data volumes and complexity have grown, so have the technology available to break down the barriers that separate organizations from data-driven insights.
Whether your organization has a data lake or not, it’s likely your data journey is not as smooth as you’d like. One trend becoming more popular is using a cloud data warehouse as the data lake or even data “ocean.” Depending on the data warehouse, the benefits could extend to: ingesting all of the data in a single location, bypassing intermediate technology solutions, achieving low-latency relational analytics, and obtaining virtually unlimited, multi-workgroup concurrency scaling.
Moving on from Hope and Hadoop – Finally
I’ll leave you with one more thing: The “dream” of the modern data lake is no longer a dream. When the data lake emerged a decade ago, features such unlimited storage capacity, low-cost storage pricing, instant cloud scaling and fast analytics were indeed dreams.
Fast forward to today. While the toolkit approach of combining hope with Hadoop and assorted solutions is still out there, it still isn’t working, and it never will. The organizations that truly understand where things are going as the global economy increasingly runs on data as its fuel are leaving traditional data lakes in the technology rearview mirror and moving on to modern cloud data lakes and data oceans powered by cloud data warehouses nearly without limits. But that’s just today! I truly believe that the best is yet to come with data technology. Stay tuned.
About the Author
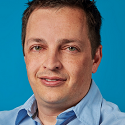
Christian Kleinerman is VP of Product at Snowflake. Christian is a database expert with over 20 years of experience working with various database technologies, currently serving as Vice President of Product at Snowflake. He has more than 15 years of management and leadership experience. At Microsoft, he served as General Manager of the Data Warehousing product unit where he was responsible for a broad portfolio of products. Most recently, Christian worked at Google leading YouTube’s infrastructure and data systems. Christian earned his bachelor’s degree in Industrial Engineering from Los Andes University, and he currently holds more than 10 patents in database technologies.
Sign up for the free insideAI News newsletter.
Speak Your Mind