In a perfect world, it should be easy to get your data and analyze it. We are not in a perfect world. In the real world, data is generated from many applications, IoT sensors, server logs, containers, cloud services, and more. Organizations today use real-time data streams to keep up with evolving business requirements. Setting up data pipelines is easy. Handling the errors at each stage of the pipeline and not losing data is hard.
With all of these data streams generated from various sources and in various formats, it’s difficult to analyze the data to get valuable insights when you’re dealing with system failures, data quality, and data intake variation. Add to this that organizations are entering the multi-petabyte and eventually exabyte range, and all of these issues are compounded.
Data provenance presents even more challenges. Many organizations could have several copies of data with the similar provenance in different places with slightly tweaked content. How do you tell how each of the copies is different or which version you want?
You have to be able to follow the data ‘through the crevices’ to see what changed, when it changed, and the cause of the problem. This can take months for the most talented data scientist – many small and medium organizations don’t have data scientists to solve these issues.
Current solutions on the market are expensive. All sizes of companies need help with tool sprawl and keeping the budget in control and observability standards will help ease the pressure.
Real-time observability is critical, and using tiered storage and the cloud is beneficial. Operational complexity can be managed from the number of engineers to the total cost to manage the systems.
The ideal world, with observability standards, will give companies observability data management strategies that offer the ability to handle storage and the management of data at rest and in motion with a cohesive infrastructure where problems are easy to troubleshoot and diagnose.
In this scenario, it’s easy to see if the system is working as intended and everything is managed in a single place, like a data fabric. It’s not practical for companies to learn to speak three different languages in order to monitor data or manage their database. You should be able to say what you want of the data and when and not spend a huge amount of time dealing with data structure or finding the needle in the haystack when business processes are interrupted.
A data pipeline that incorporates an effective approach to back-pressure management, visualization, and data provenance translates into less troubleshooting, faster recovery, and cost reduction for your business.
About the Author
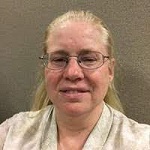
Karen Pieper is currently VP of engineering at Era Software. She holds a Ph.D. from Stanford in Computer Science and has focused her career on solving hard tech problems. She was in chip design for 20 years, working on simulation and synthesis algorithms. She then moved to AWS, Facebook, and ERA Software focusing on terabyte and petabyte scale databases and data pipelines.
Sign up for the free insideAI News newsletter.
Join us on Twitter: @InsideBigData1 – https://twitter.com/InsideBigData1
Speak Your Mind