Business intelligence (BI) has had a trajectory of continuous democratization, from traditional, standalone BI platforms that required significant effort to deliver static reports to a select few to modern analytics that shifted access from the few to the many and from content creation to data consumption.
But have modern analytics platforms involving more data, more users, and more use cases delivered proportional business value? In most instances, business users often give up when trying to find the right data because of the proliferation of data sources and dashboards. This is where curated data experiences — embedded analytics and embedded artificial intelligence (AI) can help — not just for customers and external use cases but in internal, user-facing applications, too.
First, before organizations can deliver embedded analytics and embedded AI, they need a universal semantic layer—an independent yet interoperable translation layer—between the data repository and the data-consuming endpoints. The semantic layer provides a consistent and trusted view of unified data by organizing, simplifying, and accelerating its consumption. Once a universal semantic layer is in place, curated data experiences are relatively easy to deliver, internally and externally. Although many organizations start with customer-facing applications, it is worth mentioning that substantial value can be derived quickly from focusing on internal processes first.
Decisions in context
The first thing many organizations do after implementing a universal semantic layer is create embedded analytics solutions that improve internal decision-making. With embedded analytics, users can access data when and where they need it most: in the context of their workflows. Instead of viewing a dashboard in the analytics platform and switching to the business application to act on it, businesses can curate the analytics experience within an application or a custom solution so there’s less jumping among applications and more immediate relevance. Taking it one step further, organizations can drive the experience using embedded AI so that users can bring up the right data within the business application using a simple voice command or chatbot.
Improved employee experience
By incorporating metrics into the process, embedded analytics increases productivity and engagement. Well-curated embedded experiences enhance performance throughout the company by providing pertinent information for particular jobs. For example, marketing teams can receive almost instantaneous updates on lead generation, purchasing patterns, and customer acquisition costs using embedded analytics. Instead of manually compiling data from many systems, marketers can make quicker, more educated decisions to produce more high-quality leads with instant access to this data, even via natural language commands with embedded AI.
Better workflows and processes
Embedded analytics incorporates data-driven insights straight into workflows to optimize business processes. Think about supply chain management and all of the intricate logistics and operational details involved. Companies can monitor inventory levels, supplier performance, and demand forecasts in real-time by embedding into supply chain management systems. A holistic perspective of the supply chain enables managers to optimize productivity and reduce expenses more skillfully. They might, for example, modify inventory levels or optimize route planning with voice instructions using embedded AI to keep things operating properly.
AI to further democratize data
When paired, the semantic layer and AI unlock profound capabilities in informing business users in real-time and context. For example, a universal semantic layer makes it possible to embed AI-assisted analytics into a tool like Salesforce, allowing for analysis on deals, prospects, and other key metrics to be done without context-switching — and via a near real-time process that can be as easy as querying an AI chatbot.
Of course, an AI-ready universal semantic layer can also power customer-facing applications that enable organizations to make the most of their data and their customer interactions. Imagine a bank embedding an AI chatbot that lets the customer create a monthly budget based on income, regular spending, and savings goals or a shopping recommendation engine that curates clothing ensembles based on inventory and customer preferences.
A final thought
AI and embedded analytics powered by a semantic layer transform the way data is used in an organization, replacing the conventional, frequently fragmented method with one that is more integrated, perceptive, and useful. Businesses can enhance employee experiences and turn isolated data interactions into targeted insights that any employee in the company can easily access to promote growth, innovation, and continuous improvement. By integrating analytics and AI directly into the operational tools that staff members use on a daily basis or into customer interactions to transform their experiences, businesses can derive the full value of data at last.
About the Author
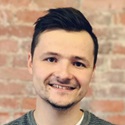
Artyom Keydunov is co-founder and CEO of Cube, a venture-funded provider of a semantic layer for data apps. Prior to Cube, Keydunov co-founded Statsbot, a data platform.
Sign up for the free insideAI News newsletter.
Join us on Twitter: https://twitter.com/InsideBigData1
Join us on LinkedIn: https://www.linkedin.com/company/insideainews/
Join us on Facebook: https://www.facebook.com/insideAINEWSNOW
Speak Your Mind