Nearly every company is striving to become a better user of its data and make more informed decisions across the organization, and the cloud computing and technology sectors have exploded as platform providers try to make that vision a reality. So why, then, do only 24% of executives view their companies as being data-driven, and just 13% of executives believe their organizations are delivering on their data strategy?
Considering that we’re now in a modern data era where we’re discussing advanced data concepts like LLMs, some businesses thrive on not just collecting data, but striving to turn it into a strategic asset. Despite advances in techniques like neural networks and machine learning models, it’s surprising that only a small percentage of companies consider themselves fully data-driven. This inconsistency is not an anomaly, but rather a sign of significant, structural obstacles. The reason? These three major roadblocks that keep companies from tapping into the full potential of their data.
1. Isolated Environments for Data Quality:
Many ETL tools and data warehousing solutions have built-in data validation features, but they often exist as separate functions and do not cater to enterprise-wide data quality checks. Imagine a smart home system where each device is made by a different manufacturer. Your advanced lighting system doesn’t communicate with your top-of-the-line coffee maker. You may get great individual performances, but the lack of coordination turns your ‘smart’ home into a missed opportunity.
It is highly advisable to seek a comprehensive data quality solution that can efficiently address a wide range of your data quality requirements. These may include validating both business and technical rules, facilitating reconciliation processes, conducting data profiling, offering a user-friendly interface with a gentle learning curve, providing seamless integration with various tools, and expeditiously delivering substantial business value at a large scale.
2. Handle large data volumes:
As businesses deal with larger and larger volumes of data, many still use old-fashioned methods like random sampling for validation, semi-automated scripts — a technique that is inadequate for today’s needs. It’s like using a rowboat to cross the Atlantic when you have access to cargo ships with the latest navigation systems. You’ll eventually get there, but you’ll waste time, energy, and resources.
Today, the speed and other technologies that are available means that users can validate and identify exact mismatches across entire datasets at lightning speed. Use modern technologies, especially those powered by AI and machine learning. These can sort through vast amounts of data in real-time, validating, flagging, and enriching information, laying the groundwork for a strong, data-driven strategy. Now, instead of extraneous manual effort, organizations can take any dataset and rest assured that it’ll be tagged and flagged if it doesn’t follow set business rules. By relying on sophisticated distributed computing frameworks that are able to validate datasets much more quickly and effectively, companies can begin to put data to work.
3. A ballooning number of tools at our disposal:
The market is crowded with tools designed for highly specific tasks, leaving companies with a fragmented and often ineffective set of tools. For the most part, these new technologies have popped up to address singular pain points: one is suited for only data transfers, one only for transformations, one only for streaming, and the list goes on. Imagine a Swiss Army knife where each blade can do one task very well but can’t work together with the others. What you need is not a collection of separate blades, but a truly multi-functional tool, which pulls numerous features under one umbrella to facilitate better integrations and more streamlined processes.
The solution is to adopt platforms that serve as this ‘multi-tool,’ providing integrated functions that manage, validate, and analyze data under one roof.
A data product moves us past these obstacles to empower us to start using data to its full potential
Data product platforms are the ideal solution to these bottlenecks. They act as cohesive ecosystems, harmonizing fragmented data landscapes into unified, accessible, and actionable data repositories. By setting universal standards for data quality and validation, these platforms open-up opportunities for deeper insights, strong decision-making, and increased profitability and innovation. These data product platforms provide an in-depth look at the challenges of using data effectively while offering practical solutions and highlighting the high stakes involved in each barrier.
By recognizing and addressing these barriers head-on, we become not only skilled data collectors but also equipped to turn this data into actionable intelligence. This fuels growth, drives innovation, and creates a business model that is not only sustainable but constantly evolving.
About the Author
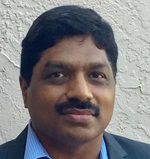
Sridhar Bankuru is the VP of Software Development at RightData. Sridhar a brings broad range of implementation experience, managed complex client’s distinctive set of challenges, solving strategic and operational issues especially in data integrations & data quality space. Before co-founding RightData, he has been part of many successful implementations playing architect / leadership roles, has experience as an accomplished data architect, hands-on as designer, and worked on performance.
Sign up for the free insideAI News newsletter.
Join us on Twitter: https://twitter.com/InsideBigData1
Join us on LinkedIn: https://www.linkedin.com/company/insidebigdata/
Join us on Facebook: https://www.facebook.com/insideAI NewsNOW
Speak Your Mind