Healthcare organizations are collecting and storing more patient data than ever. Getting value from that data, however, remains a challenge because much of it is in unstructured form, buried within text-based documents created by clinicians across the healthcare landscape. Fortunately, advances in natural language processing (NLP) are making it possible for healthcare organizations today to derive searchable, actionable insights at scale from data that previously was inaccessible.
Still, for all the news about the amazing capabilities of a massive language model like ChatGPT, people are realizing the model can invent plausible but untrue information that is fluently combined with true information. For medical NLP applications, this serves as a cautionary tale.
Thus far, NLP traditionally used in healthcare – which is highly complex – has been disappointingly inaccurate. As medical NLP matures to use technology underlying large language models like ChatGPT, the key is leveraging deep-learning models built by medical experts knowledgeable in medical language across the care continuum and making these models explainable to medical experts. This will ensure that the output of the model can be grounded to the original sources of information in medical reports, thus delivering valuable and verifiable information to clinicians.
Hidden patient data
Healthcare organizations generate massive quantities of historical patient data that is stored in electronic health records (EHR) systems. Most of this data – roughly 80% – is in unstructured text. This makes it exceedingly difficult for clinicians at the point of care to access the data without painstakingly reading it all, which often precludes them even knowing the information exists in the data. Unstructured data isn’t actionable unless it can be retrieved, understood, and contextualized.
Historically, providers have had human experts pore through unstructured data to uncover and interpret relevant clinical information. Such a human-driven process simply can’t be scaled to allow millions of unstructured notes to be accessed and gleaned for useful information in a timely fashion.
In recent years, healthcare organizations have tried to apply NLP to unstructured clinical data. Results have been subpar, though, because the NLP technology used to date has been far from medical grade.
Let’s say one of the clinical reports indicates that a patient has had coronary artery bypass surgery. Apart from the fact that clinicians often use short forms like ‘cabg’ to refer to this type of procedure, just finding the information alone may be insufficient for a clinician, who also may want to know when the patient had the procedure, and whether there were complications. Less sophisticated NLP software would not be able to provide proper context for this data to a clinician at the point of care.
The reason is because older medical NLP software is based on terminologies – identifying all the different terms for a certain symptom or disorder, for example – that it would list out and try to find matches for in the data. While this requires some level of medical knowledge, older medical NLP would fail when presented with ambiguity in the form of data it can’t interpret. One byproduct of this ambiguity is that older NLP platforms would produce overwhelming amounts of output – exactly what a clinician at the point of care doesn’t need.
Clinical support at the point of care
Deep learning is quickly transforming how medical NLP can help both providers at the point of care and medical researchers by quickly finding relevant clinical information within massive amounts of unstructured data. Nonetheless, deep learning models are not without their limitations. They are prone to overconfidence even when they are wrong, they need to be “fine-tuned” on reliable high-quality data, and they need to be explainable to human experts so the extracted information is easily grounded in the clinical text data used to extract the information.
It is critical, then, to ensure deep learning models are trained on highly accurate medical knowledge that goes beyond recognizing terminologies to making relevant clinical connections and accurate inferences based on a holistic view of data. This presents huge opportunities for healthcare providers, clinical researchers, and payers to discover valuable information related to disease progression, treatment efficacy, population health trends, and many other use cases that would have been infeasible to identify using manual data review and analysis techniques.
The goal of medical NLP and deep learning models for medicine should not be to replace clinicians so diagnoses and reports can be produced by machines. Rather, it should provide clinicians with the most accurate and relevant clinical information about a patient at the point of care. Accomplishing this will require a collaboration between AI-powered medical NLP and clinicians with vast medical knowledge. It’s these collaborations that will finally deliver on the promise of medical NLP.
About the Author
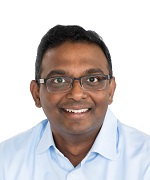
Anoop Sarkar, PhD, is the Chief Technology Officer for emtelligent, a leader in the development of clinical-grade natural language processing (NLP) software for healthcare organizations. Dr. Sarkar is a renowned expert in machine learning for NLP and also serves as Professor of Computer Science at Simon Fraser University in British Columbia. Dr. Sarkar has published more than 90 research articles with more than 4300 citations. He holds a PhD in Computer Science from the University of Pennsylvania. He published his first NLP paper in the 1993 meeting of the Association for Computational Linguistics (ACL).
Sign up for the free insideAI News newsletter.
Join us on Twitter: https://twitter.com/InsideBigData1
Join us on LinkedIn: https://www.linkedin.com/company/insidebigdata/
Join us on Facebook: https://www.facebook.com/insideAI NewsNOW
Speak Your Mind