The AI economy will benefit incumbents – if they can leverage their proprietary data. To prepare for ‘AI eating software,’ enterprises need to standardize data collection processes across the organization.
AI is the next commodity
Last June, OpenAI released GPT-3, their newest text-generating AI model. As seen in the deluge of Twitter demos, GPT-3 works so well that people have generated text-based DevOps pipelines, complex SQL queries, Figma designs, and even code. While the examples are obviously cherry-picked, this still represents powerful out of the box functionality.
This baseline predictive indicates rapidly changing market dynamics. The AI capabilities of OpenAI, public clouds, and even open-source variants are rapidly evolving. Regardless of the ultimate vendor, many predictive tasks will become easily accessible via some ‘Open’ AI API in the coming years. If prediction succeeds compute (as compute succeeded electricity), the new base unit of the enterprise becomes machine intelligence.
This commoditization would fundamentally shift the market dynamics of industries built on AI platforms. Specifically, AI products will no longer differentiated by many of their economic moats. Rather than technical differentiation from models or algorithms, the competitive edge will now come from proprietary data sources and the speed at which companies can engineer features.
Technical (in)differentiation: 10x products build technology moats and win markets. But if two companies built chatbots built on a powerful (generic) API, there would likely be 10%, not 10X, differences in technology.
Strong AI APIs limit technologic differentiation because it becomes dramatically harder to improve much beyond baseline performance. In fact, many tuned GPT-2 models perform worse than an untrained GPT-3 at their specific task.
(Dis)economies of scale: Companies benefit from scale because of fixed infrastructural and hardware costs. But adding AI to a product introduces variable costs for cloud infrastructure, and model training.
If AI is consumed via API, there would be a linear per-prediction price. And if AI were consumed via a personally managed OSS model, the cost to pay for the necessary infrastructure remains linear. Even if the cost of API access decreases as competitors trained their own models, machine intelligence remains a fixed marginal cost. While the API pricing may decrease, AI-powered product margins don’t benefit from economies of scale. In fact, scaling AI systems means dealing with a long-tail containing increasingly hard to wrangle data for edge cases.
These open-access models give rise to undifferentiated AI products. As historical moats no longer provide an enduring competitive advantage, competing products can more easily emerge.
While AI API providers and distribution platforms are the logical beneficiaries from this evolving technology, established incumbents stand to benefit. In Christensen’s terms, ‘Open’ AI is a sustaining innovation that benefits the existing players. Enterprises can easily integrate AI into their product, outcompeting entrants with their preexisting branding, distribution, and data. Further, many inhibiting factors for AI in startups don’t hold for incumbents.
Incumbents can handle the long-tail
AI products are expected to handle the long-tail of user intent. Think about search: while it’s trivial for to return answers to commonly answered questions eg. “price of GameStop”, Google is also expected to handle your incredibly complex, never-been-seen-before query. In fact, some 40–50% of functionality in AI products resides in this long-tail.
As a solution, companies are commonly recommended to ‘bound problems’ or collect data to solve the ‘global long-tail’ problem (the same problem shared between everyone). Enterprises can more easily do both.
Bootstrapping a product’s capability with AI means that the problem space is already bounded. Because the intended functionality had to exist without AI, the domain should already be sufficiently narrow to benefit from more advanced techniques.
Solving the global long-tail is easier for enterprises because their product should already be able to generate the data necessary to solve the problem. Their proprietary data can be used to increase model coverage.
The hidden costs of AI
Despite the clear value add of AI, there are additional cost considerations. A16Z’s The New Business of AI kicked off a flurry of conversations around the unit economics of AI. Martin Casado and Matt Bornstein argue that AI startups represent a new type of business that operates like “Software + Services” due to lower gross margins, scaling challenges, and weaker defensive moats.
The additional complexity of training and deploying ML models requires additional operational costs above typical software development costs. Managing, processing, and training with these vast corpuses of proprietary data is expensive. Because of these fundamental differences, AI businesses consistently report gross margins in the 50–60% range, as opposed to the 60–80+% typical of SaaS.
Increased cost of infrastructure: Today’s massive ML models are computationally intensive, requiring a large portion of the operating budget to be spent on cloud infrastructure. While many startups don’t report specifics, it’s estimated that roughly 25%+ of revenue is spend on cloud resources. Further, the operational complexity for managing data and metadata (as well as models) quickly exceeds that for simple SaaS or legacy products.
Increased cost of labor: Gross margins are further lowered by the need for a ‘human in the loop’. During model training, the data that powers supervised learning often requires manual cleaning and labeling. And in production, humans still play a large role as joint decision makers. Many ‘AI’ products are no more than ML-produced suggestions fed to human moderators with final authority. Even if AI improved to a point where it technically required less assistance, current debates around fairness and bias show that human oversight will likely remain to counteract the ethical implications of these black box models.
Increased cost of deployment: Whereas a typical SaaS product can be deployed to a customer instantly with zero marginal cost, AI products can require time and resources spent during on-boarding. Understanding and fitting models to the customer’s data distributions can be a time intensive process. Because inputs from each new deployment can vary dramatically, each customer represents a new long-tail of edge cases to consider.
Preparing your enterprise for AI
Collecting this proprietary data is necessary but expensive. Standardize and simplify your data collection processes to best position your enterprise for these changing market dynamics. Specifically, the steps below will help you improve the unit economics of your future AI-enabled products.
Tame your infrastructural complexity: The common trope with ML systems is the vast complexity. As captured in the quintessential Hidden Technical Debt in Machine Learning Systems, the actual model represents only a small fraction of the total infrastructure. The rest is held together with (hopefully not) pipeline sprawl and spaghetti code.
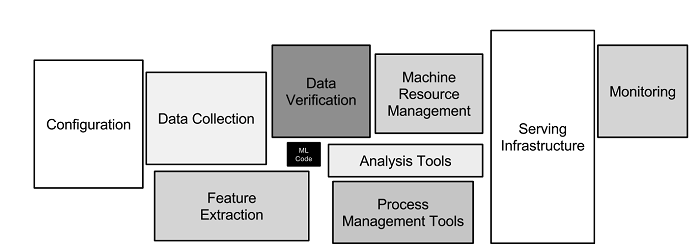
Automating this infrastructure helps reduce time to serve. If proprietary data and features are the new competitive edge, reducing complexity for feature engineering gives your product massive leverage. Luckily, whole products and industries have already emerged to solve this problem.
Structure your data: Structure your data up-front or invest in mechanisms to cope with permanently unstructured data. Adding this metadata on top allows creates a structured transactional layer. This helps both for feature development from internal data discovery as well as for ingestion into BI systems that aid analytic decision making.
Standardize your data collection: The benefit of incumbency comes in large part from your proprietary data. This unique corpus is a defensible moat that new entrants will struggle to recreate. However, your data is only a strategic benefit if you can both collect and process it. With ad-hoc collection processes or lack of a systematized approach, you won’t be able to leverage the full extent of your data. And without collection systems, you’re completely ceding your strategic position in the market. Without either of these in place, your product is at risk of being cannibalized by challengers.
About the Author
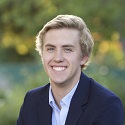
Taggart Bonham is Product Manager of Global AI at F5 Networks. His background is ML software engineering and venture capital, focusing on the AI and smart city sectors. At F5, his focus is on building and scaling AI platforms to enable customers to unlock the power of their data with application insights.
Sign up for the free insideAI News newsletter.
Join us on Twitter: @InsideBigData1 – https://twitter.com/InsideBigData1
Speak Your Mind