The proverbial AI “Arms Race” has brought about equal parts excitement and concern within the AI community. Most recently, the ongoing implementation and development of Generative AI tools, such as ChatGPT, Bard, and Bing AI, have made both AI evangelists and skeptics further dig into their stances. For advocates of AI adoption, these tools show the potential for AI to do great things, while skeptics may argue that if these tools go unchecked, they will cause more harm than good to the AI community and the world at large.
Fortunately, there is a solution that can help make both parties happy: reinforcement learning. This concept is heavily lenient on the human element of AI: from data collection to testing and re-training, reinforcement learning makes sure the human behind the AI will help create ethical, robust models moving forward. Through a more human-centric approach to training, AI practitioners can be confident that they are driving good behavior and mitigating the risk of bad or harmful behavior within their AI models. Now that we have an understanding of what reinforcement learning is, we can further examine the different use cases where it can have a true impact on the AI training and development process.
One of the foremost scenarios of when reinforcement learning can provide substantial benefits is through ongoing training and upkeep of chatbots, such as the aforementioned ChatGPT, Bard, and BingAI tools. For example, when interacting with an AI chatbot, the expectation of most (if not all) people is that the conversation will be as authentic as possible. After all, authenticity drives a good user experience.
What would happen, however, if a chatbot interaction started to hallucinate? It’s likely that if that were the case, you won’t want to interact with that service again, and recommend that your peers, friends, and colleagues do the same. With that in mind, AI practitioners must take it upon themselves to ensure that these bad experiences do not occur. Chatbots benefit from reinforcement learning, especially with human feedback loops included, as these learning methods help train the models to understand different emotions, signals, requests to help businesses deliver quality experiences, and models are molded by the people who train them. Therefore the trainer needs to be diverse – culturally, ethically, and geographically. Some key areas of focus include putting ethics, responsibility, diversity & inclusion as the foundation to drive innovation, inspiration and trust.
While chatbot training and reinforcement is perhaps the most popular instance where reinforcement learning can have an impact on AI, there are other use cases where it can make a difference as well. Other examples include using reinforcement learning to improve AI-generated image and text captions, helping train AI performance in online gaming, computer vision in robotics, recommendation systems for shopping or watching shows, and helping improve the training and retraining process by helping generate properly labeled and sorted training data.
In sum, the key benefits of reinforcement learning, especially for companies entering into the Generative AI space, is that it will provide consistent, ongoing oversight that will help practitioners identify key areas of improvement throughout the AI lifecycle. Taking it a step further, however, we can look at this from an ethical lens.
Despite constant back-and-forth on when (and whenever) AI will be sentient enough to understand the implications of its own words and actions, the path to long-term sustainability and growth for AI will always involve human reinforcement and teaching. By building, developing, and maintaining effective AI models through human reinforcement, the industry can help ensure that Generative AI – and the AI industry as a whole – are providing profound, ethical impact on its users daily.
About the Author
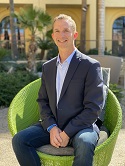
Kim Stagg joined Appen in August 2022 as VP of Product, responsible for product management for Crowd, Trust, and Enterprise Data Warehouse. He brings with him over 20 years of global experience in product and software. His core expertise is bringing complex modeling, analytics, and statistical techniques to commercial applications through SaaS. Kim holds a PhD in Hydrogeology & Computer Science from the University of Birmingham, an MSc in Engineering Geology from the University of Leeds, and a bachelor’s degree in geology from the Imperial College London.
Sign up for the free insideAI News newsletter.
Join us on Twitter: https://twitter.com/InsideBigData1
Join us on LinkedIn: https://www.linkedin.com/company/insidebigdata/
Join us on Facebook: https://www.facebook.com/insideAI NewsNOW
Speak Your Mind