Enterprises all over the world are generating enormous amounts of data (80% of the world’s data is in enterprise) but they are struggling to extract insights from it. In many cases, this is because they are still attempting to process the data using outdated technologies. Graph technology offers a solution and is set for explosive growth in the years ahead, Gartner predicts that by 2025, graph technologies will be used in 80% of data and analytics technologies.
Graph technologies work by organizing unstructured data sets as linked structures of nodes and edges, and processing the data using algorithms and machine learning techniques, specialized to linked data structures. In spite of their power, two problems currently limit the wide-spread adoption of graph technologies: a lack of understanding among technology buyers of what graph technology can do for them, and the difficulty that many graph platforms have in interoperating seamlessly with third-party libraries and other systems in data processing pipelines.
To address the first problem, the graph community must highlight use cases in which graph technologies have been deployed successfully. For example, one example of a use case is in cybersecurity. Intrusion detection in computer networks is often performed by building data provenance graphs that track how data is generated and used in computer networks, and then analyzing these graphs for forbidden patterns of data access. Another use case is in the financial services industry, where graph technology can be employed to detect financial fraud. The key idea is to build and analyze interaction graphs that connect people, accounts, and transactions, and then look for patterns such as rings of suspicious transactions to ensure that transactions are legitimate. Highlighting and explaining these kinds of successes are essential if the full potential of graph technology is to be realized across other industries.
But graph technology’s uptake across verticals and sectors is also hamstrung by the limitations of many existing graph platforms. Some graph platforms are single-machine systems, which limits the size of the data sets that can be processed in a reasonable amount of time. The solution is to build scale-out graph platforms in which graphs are shared or partitioned across the memories of different machines of a cluster, permitting systems to handle much bigger datasets more efficiently.
Another problem with many existing graph platforms is their limited interoperability with other libraries and data processing systems. For example, all major pharmaceutical companies are actively building and exploiting medical knowledge graphs, but they also make extensive use of third-party cheminformatics libraries that support functionality like the computation of chemical similarity scores. It is essential for the graph platform to interoperate seamlessly with such libraries because a typical data processing pipeline involves using a graph database query to select a subgraph of the knowledge graph, passing the subgraph to the cheminformatics library to compute features for the nodes of the graph, and then passing the resulting property graph to a graph convolutional network that computes a vector-space model for the graph.
A different issue is interoperation with other data processing systems. In many data processing pipelines, graph computing is one of a series of operations that must be performed on the data, with other operations being performed on other big-data processing systems. In this context, a lack of seamless integration between systems can become expensive because substantial time and computation may be spent transferring data between different systems. One example is an identity management company that attempted to employ a graph technology platform that did not have an advanced integration with Spark, a data computing language. The company was still able to get the job done, but with more machines,energy expended, and a higher cost of operation. Once the company shifted over to a graph technology platform that had native Spark integration, the client was able to perform data transfer and analysis more efficiently, which led to a substantial reduction in costs.
Graph technology’s need is apparent – and will be even more so in the coming years with 95% of businesses stating that managing unstructured data is a serious challenge. Highlighting successes and promoting interoperability with other libraries and data processing systems will ensure that graph technology has a bright future.
About the Author
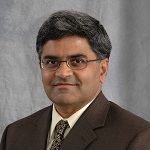
Keshav Pingali is CEO and co-founder of Katana Graph, the AI-powered Graph Intelligence Platform providing faster, deeper and more accurate insights on massive and complex data. Keshav holds the W.A.”Tex” Moncrief Chair of Computing at the University of Texas at Austin, and is a Fellow of the ACM, IEEE and AAAS.
Sign up for the free insideAI News newsletter.
Join us on Twitter: @InsideBigData1 – https://twitter.com/InsideBigData1
Speak Your Mind