A group of AI researchers from DarwinAI and out of the University of Waterloo, announced an important theoretical development in deep learning around “attention condensers.” The paper describing this important advancement is: “TinySpeech: Attention Condensers for Deep Speech Recognition Neural Networks on Edge Devices,” by Alexander Wong, et al. Wong is DarwinAI’s CTO.
By integrating deep attention condensers into their GenSynth platform, the Darwin team was able to construct two highly efficient automatic speech recognition (ASR) models for edge devices. The smallest of these models, termed TinySpeech-B, is over 200x smaller and 20x less complex than previous models with the same accuracy. Moreover, as the models uses 8-bit low-precision parameters, its storage requirements are over 800 times lower than equivalent networks.
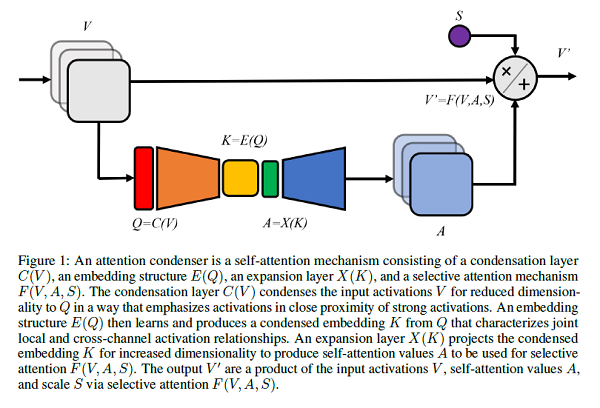
“This is an important theoretical advancement that allows a deep learning model to hone in on the “important” aspects of input the way a human might,” said Sheldon Fernandez, CEO of DarwinAI. “In the main, this facilitates more effective and trustworthy decision-making in AI, resulting in neural networks that are both more efficient and robust”.
Mcuh liek you can pasre this snetnece evne thuogh its phoentcially flwaed, attention condensers are unique, stand-alone architectures that allow a deep learning model to better focus on “what’s important,” facilitating more effective and trustworthy decisions.
In a broad sense, this breakthrough is related to Moravec’s paradox (see figure below), an observation by AI researchers that high-level reasoning in humans requires little computation whereas low-level sensorimotor skills require a lot. By using human-like shortcuts, we’ve demonstrated that attention condensers can dramatically accelerate AI and reduce its complexity.
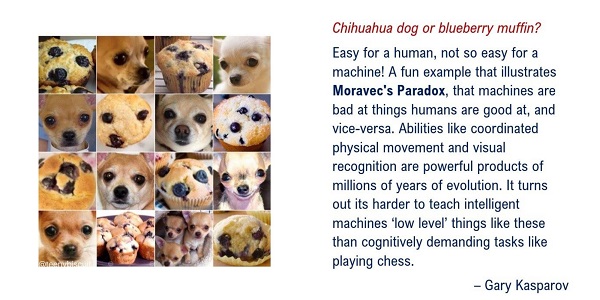
Sign up for the free insideAI News newsletter.
Speak Your Mind