Top Data Scientists Honored for Advanced Research and Applied Data Science in the Field of Knowledge Discovery in Data and Data Mining
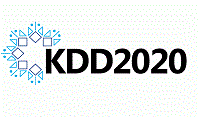
KDD 2020, the premier interdisciplinary conference in data science (which took place virtually Aug. 23-27, 2020), announced the recipients of the SIGKDD Best Paper Awards, recognizing papers presented at the annual SIGKDD conference that advanced the fundamental understanding of the field of knowledge discovery in data and data mining. Winners were selected from more than 2,000 papers initially submitted for consideration to be presented at the conference. Of the 338 papers chosen for the conference, three awards were granted: Best Paper in the Research Track, Best Paper in the Applied Data Science Track, and Best Student Paper.
“There was unprecedented interest in presenting advanced peer-reviewed papers in data science at KDD 2020 and the quality of submissions was outstanding,” noted Dr. Michael Pazzani, Chair of the best research paper selection committee of KDD 2020 and distinguished scientist at UC San Diego. “The award committee deliberated long and hard to coalesce on the papers we felt surpassed all others in terms of potential impact on the industry and superior understanding of the field of knowledge discovery in data science.” Anima Anandkumar, Bren professor at Caltech and a director of machine learning research at NVIDIA, joined Dr. Pazzani on the organization committee as chairperson for the Applied Data Science track.
The SIGKDD Best Papers of 2020 are as follows:
- Research Track: “On Sampled Metrics for Item Recommendation,” by Walid Krichene (Google) and Steffen Rendle (Google) — Having investigated sampled metrics in great detail, researchers show that sampled metrics are inconsistent with their exact version, in the sense that they do not persist relative statements. The paper illustrates that it is possible to improve the quality of sampled metrics by applying a correction, which is obtained by minimizing different criteria such as bias or mean squared error, and provides a novel perspective for understanding the problems of sampling in recommendation evaluation with an innovative analysis and solid results.
- Applied Data Science Track: “Temporal-Contextual Recommendation in Real-Time,” by Yifei Ma (Amazon), Murali Balakrishnan Narayanaswamy (Amazon), Haibin Lin (Amazon) and Hao Ding (Amazon) — The authors present a black-box recommender system that can adapt to a diverse set of scenarios without the need for manual tuning. The paper addresses two fundamental challenges when putting recommender systems in the real-world: how to efficiently train them even with millions of unique items and how to cope with changing item popularity trends, while also introducing a compact model to address real-time and diverse metadata needs.
- Student Paper: “TIPRDC: Task-Independent Privacy-Respecting Data Crowdsourcing Framework for Deep Learning with Anonymized Intermediate Representations,” by Ang Li (Duke University), Yixiao Duan (Beihang University), Huanrui Yang (Duke University), Yiran Chen (Duke University) and Jianlei Yang (Beihang University) — The students present a task-independent privacy-respecting data crowdsourcing framework with anonymized intermediate representation. The goal of this framework is to learn a feature extractor that can hide the privacy information from the intermediate representations, while maximally retaining the original information embedded in the raw data for the data collector to accomplish unknown learning tasks.
The technical program committees for the Research Track and the Applied Data Science Track identified and nominated a highly selective group of papers for the Best Paper Awards. The nominated papers were then independently reviewed by two separate committees led by Professor Michael Pazzani, UC San Diego (Research Track) and Professor Anima Anandkumar, California Institute of Technology (Applied Data Science Track).
Sign up for the free insideAI News newsletter.
Speak Your Mind