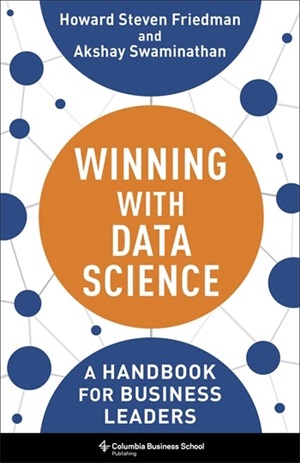
About 90% of businesses say they plan to increase their investments in data, analytics and AI, but many businesses are struggling to gain value from data science investments. These failures lead to a waste of money, time and opportunity for the company.
Data science efforts can fail for two main reasons: business-related reasons and data-related reasons. In this first of two articles, adapted from our book Winning with Data Science, we will discuss some of the most common business-related reasons for these failures and what you can do to avoid these pitfalls. Our follow-up article will explore some of the data-related issues.
Before detailing some of the key business-issues, it is important to emphasize that business customers (otherwise known as stakeholders or collaborators) play a crucial role in the success (or failure) of data-related projects. They are the data science team’s customers and so they need to be actively engaged in all parts of the project. Good customers communicate their needs, invest time, ask the right questions, challenge assumptions, and learn the language.
Some important business-related mistakes in data projects include:
- Focusing on hot tech instead of problems: It’s easy to get distracted by the next big thing in tech, but data projects should solve a critical business problem, like increasing revenue or reducing expenses. Starting with the solution, whether that be Generative AI or deep learning, and then looking for the right problem is a recipe for trouble.
- Not defining success: The data science teams need to know the goals of the project and how success is measured. Being clear about five dimensions are important: the expected use of the product, time expected to build, cost of the project, the quality required and the satisfaction of the stakeholders. Your definition of success should be achievable so open communication and feedback from the data science team is critical
- Not being engaged: Simply hiring a data science team is not enough. The data science customer has to stay engaged in the process and participate in project management meetings to understand the key milestones, challenges and opportunities. Being an engaged customer will go a long way to ensuring success.
- Not asking questions: Communication is a critical part of any data science project. The customer should always ask questions that span the range of project management, product decisions, modeling, and specific recommendations made by the data science team. Most importantly, if the answers aren’t clear, then the customer should continue to ask questions – data scientists should be able to explain what they are doing and why in a language that is readily understood by non-specialists.
- Accepting assumptions: The business customer should challenge assumptions made by the data science team. After all, the business customer shows up with subject matter knowledge and experience. They have a deep understanding of the business and may understand why one definition of an outcome variable is better than another or can explain what is the minimum model performance needed in the real world.
- Not Learning the Language: Business customers should learn some of the basic terms of data science. This does not mean they need to code in Python, use Github or perform head-to-head tests of Generative AI models. It means they should understand key terms related to features, outcome variables, performance metrics and basic statistics.
- Ignoring Ethics concerns: Failing to fully address ethics concerns can result in potential biases, fairness issues, and privacy concerns that can damage trust and reputation. The last thing you want is to have your company appear on the Wall Street journal front page as an example of algorithm bias or AI ethics failures. Failing to pay attention to ethics concerns can create problems for the business owner, the company itself and those the company’s customers.
The mistakes above all stem from failures in planning, preparation and communication. The good news is that these mistakes are avoidable. Before initiating a data science project, we recommend the business customer takes the time to review this list above and decide what steps they will take to avoid the pitfalls and increase the chances of success.
Adapted from Winning with Data Science by Howard Steven Friedman and Akshay Swaminathan, published by Columbia Business School Publishing. Copyright (c) 2024 Howard Steven Friedman and Akshay Swaminathan. Used by arrangement with the Publisher. All rights reserved.
About the Authors
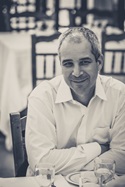
Howard Steven Friedman is a data scientist, health economist, and writer with decades of experience leading data modeling teams in the private sector, public sector, and academia. He is an adjunct professor, teaching data science, statistics, and program evaluation, at Columbia University, and has authored/co-authored over 100 scientific articles and book chapters in areas of applied statistics, health economics and politics. His previous books include Ultimate Price and Measure of a Nation, which Jared Diamond called the best book of 2012.
.
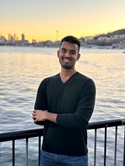
Akshay Swaminathan is a data scientist who works on strengthening health systems. He has more than forty peer-reviewed publications, and his work has been featured in the New York Times and STAT. Previously at Flatiron Health, he currently leads the data science team at Cerebral and is a Knight-Hennessy scholar at Stanford University School of Medicine.
.
Sign up for the free insideAI News newsletter.
Join us on Twitter: https://twitter.com/InsideBigData1
Join us on LinkedIn: https://www.linkedin.com/company/insidebigdata/
Join us on Facebook: https://www.facebook.com/insideAI NewsNOW
Speak Your Mind