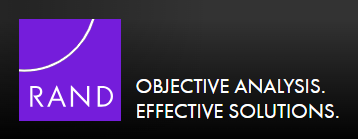
By some estimates, 80% of AI projects fail – more than double the rate of non-AI IT projects – but why? A group of RAND researchers investigated this issue and found five key problems leading to failure:
- Misunderstanding or miscommunicating the problem the AI needs to solve
- A lack of data need to adequately train an effective AI model
- A bias toward the latest and greatest technology, rather than focusing on solving real problems for their users
- A lack of adequate infrastructure to manage data and deploy completed AI models
- Applying the technology to problems too difficult for AI to solve
To avoid the same pitfalls, the authors offer a handful of recommendations for both industry and academia including choosing enduring problems; focusing on the problems instead of the technology; investing in infrastructure up front; understanding AI’s limitations and creating partnerships between academia and government.
See the full report here.
Sign up for the free insideAI News newsletter.
Join us on Twitter: https://twitter.com/InsideBigData1
Join us on LinkedIn: https://www.linkedin.com/company/insideainews/
Join us on Facebook: https://www.facebook.com/insideAINEWSNOW
Speak Your Mind