By Russell Ruben, director of automotive and emerging segment market, Western Digital
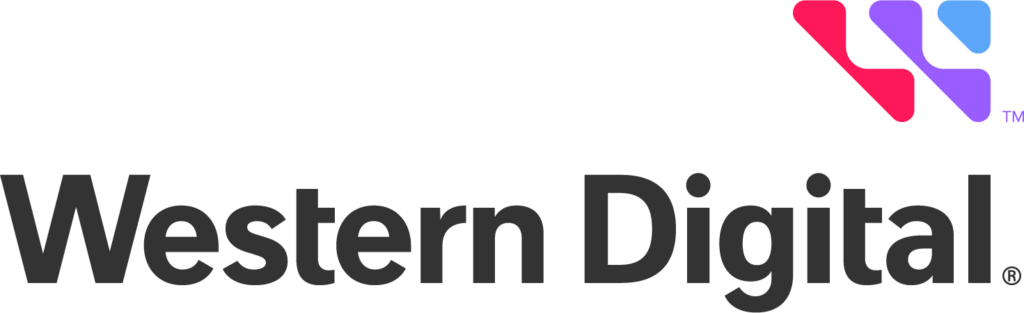
While car makers and buyers consider how much storage a vehicle has to fit physical belongings in, it’s no longer just cargo storage that’s important. Cars can now support more lines of software code than fighter jets.
Such technological advancement means that auto manufacturers need to properly equip their vehicles with well-designed data storage that will meet the specific performance, reliability, and capacity needs for a particular automotive systems.
To do so, they must first understand the importance of workload analysis. This will help support the durability and longevity that automotive systems require, enabling features that allow monitoring and providing the best user experience possible to address new applications’ needs.
Where’s all this demand for car storage coming from?
As with many other industries, consumer expectations have been a significant catalyst for storage demand in the auto industry. For example, consumers are used to having smart devices in their hands, and now expect the same level of intelligence from their vehicles.
Think lane-keep assistance, fast-charging batteries, cameras, voice recognition and Wi-Fi, are just a few of the systems common to modern cars. Western Digital estimates that NAND storage, used to handle the demands for vehicle features like these, could reach 2TB by 2025 in high-end vehicles. Commercial-fleet vehicles will likely require even more storage as their systems monitor drivers and collect data that helps optimize efficiency and operations.
As a result, storage requirements will need to be reevaluated, tested and analyzed accordingly as entirely new applications and capabilities arise utilizing cutting edge sensors, 5G, AI, machine learning and big data analytics.
Why workload analysis is so important in product development
Storage workload analysis during product development enables a proactive evaluation of how the system is accessing the storage device and the resulting useful life of the media that can be expected. This analysis identifies the potential of exceeding the specifications of the storage device.
Conducting workload analysis as a repetitive routine on evolving product generations and different setups allow identification of changing trends and unexpected patterns. Adjustments to the application software or increasing the storage-device capacity can then be made to reach the optimal years of useful life.
Some of the questions workload analysis can help answer include:
- How much data is read and written per day in a target system?
- Do unexpected or unique data patterns exist in the target system?
- What are the data transfers and what’s the implication on the write amplification factor?
Overall, real-life workload analysis gives a clearer picture of the automotive target system and what it needs from storage. Therefore, different storage solutions are required to support different use cases.
For example, some data may be needed for varying amounts of time depending on manufacturer or regulatory requirements. Data needed for a few seconds is stored in dynamic random-access memory (DRAM). Other types of information, like mapping, operation and applications must be stored longer on NAND Flash solutions.
The stages of workload analysis
Workload analysis is composed of four key stages:
- Bring up the infrastructure: To enable a tracer that will capture all desired storage events on the target automotive system
- Workload definition: To define the profile of user activities on the target system
- The workload experiment: To capture huge trace logs while running the defined workload
- System analysis: To perform post-processing system called write amplification factor (WAF) to uncover insights and draw conclusions around system and storage patterns and behaviors to help determine the expected useful life of hte storage device
The process, which should be conducted routinely, ensures that vehicles receive the best storage for the intended application and workload. It also gives engineers insights on how they are access the storage device and things that can be done in their designs to extend the useful life of the storage device. This allows for the identification of changing trends and unexpected patterns, prevents unnecessary issues in the automotive storage systems and prepares the vehicle to handle ever-evolving data demands.
Proactive workload analysis in the field
Another workload analysis approach may be done based on statistics and diagnostic information read directly from the storage device. Examples include lifetime statistics and end-of-life notifications, which can be read from storage devices in easy and standard methods.
Monitoring the progress of such statistics from storage devices in the field is an extremely powerful ability that enables proactive identification of field issues in case of unexpected excessive use of the storage, defects or any other abnormalities in the system.
Early identification of field issues allows the implementation of preventive actions before devices may reach their end of life and may suffer failures, which can require vehicle product recall. On top of monitoring field usage, such device diagnostics may also be used in the lab as an extra analysis tool. During workload analysis, these diagnostics can be used to fine-tune tests and qualification, identify setup issues, and analyze product behavior.
Keeping up with automotive storage demands
Workload analysis will only become more important as use cases become more demanding and more ground-breaking applications emerge. Enhanced infotainment, advanced driver-assistance systems (ADAS), data-recording sensors and cameras, 3D mapping, and vehicle-to-everything communication (V2X) are already transforming the industry. All will generate and require large amounts of data, and data will need to be kept in the vehicle storage to support real-time decisions.
As applications change and workloads become more intense, vehicle data storage becomes increasingly critical to the reliability, durability and user experience of the entire system.
Performing workload analysis during the vehicle development stage makes it easier to predict the expected behavior of the storage and its useful lifetime. Answering these kinds of questions produces better solutions for end users in the automotive industry.
As vehicle innovation continues over the next few years, driven by advances in sensors, 5G, AI, machine and deep learning and big data analytics, so must storage. Storage technology needs to keep up with the rapid pace of development as it becomes increasingly critical to support more and more demanding applications with mixed usages than ever before.
Speak Your Mind