Opportunities to use health data for patient benefit have never been more abundant, and recent advances in synthetic data generation are accelerating their realization.
Data from across the healthcare spectrum, including lab tests, drug adherence, and social determinants of health, have the potential to drive outcomes ranging from improved drug development efficiency to more informed policy decisions. While health data hold tremendous utility, patient privacy is paramount, and thoughtful safeguards must be implemented accordingly. For many use cases, synthetic data offers a path to extracting value from health data without the need to implement weighty safeguards.
Synthetic data is created via machine learning models that take a real dataset as input and generate a new “fake” dataset that is representative of the original real dataset. At a high level, synthetic data has two critical properties:
i) It preserves valuable patterns and relationships between variables in the underlying dataset that make it suitable for extracting insights that speak to the nature of the underlying real dataset. For example, the synthetic data generation process could be configured to preserve the mean of a numeric variable like patient height, or the correlation between two clinical events.
ii) There is no record-level correspondence between the real dataset and the output synthetic data. This protects against the risk of identifying patients in the underlying real dataset.
Recent advances in generative machine learning have elevated the quality of synthetic data to a point where it can be used for a variety of analyses. One archetypical use case that is applicable across a range of healthcare use cases is the generation of larger and more varied datasets than one has access to, such as in rare disease research or studies of populations within a small geographic area.
To take a concrete example, suppose a life sciences company wants to overlap disease registry data with medical claims data to understand outcomes of a specific disease in the context of the care that a patient received. Obtaining a dataset of sufficient volume for this analysis may be challenging if the disease is rare or if there is limited overlap between the two datasets.
This is a case where synthetic data can effectively augment the original dataset by generating new datasets to bolster the analysis. One way to think about this is to consider the synthetically generated datasets as plausible alternative real datasets. That is, if we imagine that the real dataset is a random sample from some larger dataset (for example, a random patient subset of a larger population), then the synthetic datasets can be viewed as alternative random samples from the same larger dataset. Moreover, if the researcher knows that the small real dataset is biased in some way (for example, if the patient set was disproportionately male), they could configure the synthetic data generation process to output datasets that counteract this bias.
In this case, the ability to generate high-quality synthetic data prevents the researcher from having to choose between lower confidence analysis and spending more time or money to obtain more real data.
Although there are many instances where synthetic data is able to provide utility with reduced privacy risk, it is not a magic solution that enables one to discount privacy entirely. One must protect against the inference of patient information from the input dataset that can occur if the engine is configured to preserve values from the input data with unconstrained fidelity. In recent years, quantitative metrics have been developed to evaluate such inference risks, and synthetic engines can be configured to limit the maximum permissible value of these risk metrics.
In the past twenty years, we have seen a rapid increase in the digitization and standardization of health data. With this groundwork laid, more recently, there have been concerted efforts to connect siloed health data sources in support of more impactful use cases. Synthetic data serves as a powerful complementary tool for the analyses that these use cases require, bringing us closer to maximizing data utility within the healthcare ecosystem.
About the Author
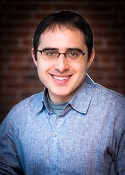
Jonah Leshin is Head of Privacy Research at Datavant, a company focused on compliant connectivity of healthcare data. Jonah is a published author in both medical informatics and theoretical mathematics. He holds a PhD in mathematics from Brown University.
Sign up for the free insideAI News newsletter.
Join us on Twitter: https://twitter.com/InsideBigData1
Join us on LinkedIn: https://www.linkedin.com/company/insidebigdata/
Join us on Facebook: https://www.facebook.com/insideAI NewsNOW
Speak Your Mind