The C-suite is rapidly being convinced of the power and effectiveness of applying automation to the data management function across the enterprise. Decision makers have witnessed what missing the full potential of data management automation means – remaining stalled in time-consuming, tedious, manual business tasks. Over time, the combined slowness of these tasks can be costly, and organizations are less able to handle dynamic and quickly changing data. Research by Gartner estimates that automation will reduce data management manual tasks by 45 percent through 2022.
In this article we’ll take a look at how’s and why’s that organizations from many industries are jumping on the automation for data management movement. It’s important that stakeholders understand how well automation performs repetitive data management responsibilities, what tasks still require a human in the loop, and how to evaluate data management automation capabilities.
Ways Automation Can Be Applied to Data Management
Automation tools serve as mechanisms for streamlining enterprise-wide data management tasks, and are designed to speed up handing business operations and reduce costs. Automating data management allows enterprises to make sense of large quantities of data by mechanizing redundant work and leaving high-level tasks to humans.
At a high level, automation for data management can target the follow areas:
- Extend & activate data workflows – automatically gathering required data and rapidly publish analyzed data to relevant systems
- Redeploy data reconciliation efforts – reducing manual effort of data reconciliation and shift focus to exception remediation
- Ensure continuous data compliance – transitioning data compliance processes from periodic activities to real-time monitoring
Specific applications of automation to data management include:
Data extraction: automation tools are able to import/load/ingest data sources that can be challenging such as web scraping, APIs, legacy applications, and complex documents (PDFs).
Data transformation and integration: many enterprises deal with a variety of database systems observing a number of different standards. It’s often necessary to connect these disparate systems, including line-of-business systems like Oracle (e.g. PeopleSoft GL), SAP, Salesforce, mainframe green screen applications, etc. Automation for data integration addresses the need to unify data sources through pipelines, and to extend data prep capabilities by structuring and aggregation from legacy sources. You can use automation to migrate/process data according to enterprise specifications without people actually having to touch the data. Human workforce can therefore focus on higher-value activities.
Data quality: cleaning data, making data consistent, resolving issues of missing data, profiling data, and linking data. In addition to transforming the data, these types of tasks ensure organizations align with well-defined rules and maintain logs detailing the processes used to make the data useable.
Inferencing: automation can assist in taking actions based on insights by AI/ML algorithms, e.g. trigger automations from BI and dashboard platforms.
Automating these kinds of data management tasks saves the manual efforts needed to make the data consumable, either at the start or end of the data pipeline. This leads to the reduction of costs and optimization of the time spent by data scientists and analysts.
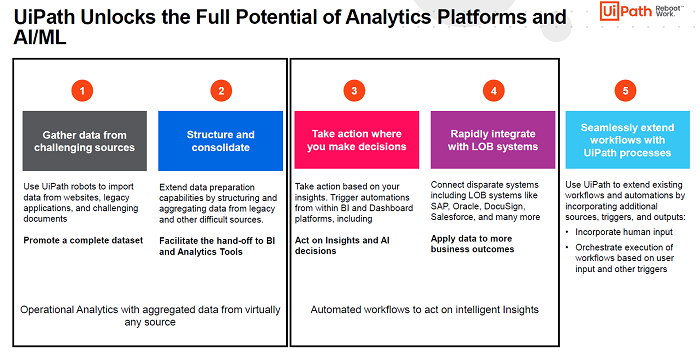
Operationalizing AI/ML with Automation
Automation tools for applications in data management work to simplify operations through AI/ML, technologies that have become quite sophisticated in identifying data patterns and adapting to business rules by matching data, detecting and correcting errors, and mapping different data elements. AI/ML allows businesses to effectively increase their ability to manage dynamic data, as both technical and business data management tasks complete in real-time.
Automation is emerging as a complementary technology ideally matched for transforming the potential of AI/ML into operational reality. A well-adapted automation platform allows enterprises to insert different AI models directly into various process stages that might otherwise require human action and/or review.
Data Management Automation Use Case Examples
There are a plethora of use case examples of applying automation to data management tasks and workflows. For instance, an invoice processing workflow can use automation along with AI models for extracting both structured and unstructured data from invoice forms for the purpose of classifying irregular or missing data, and for routing the invoice to the appropriate payment approver based on the information found on the invoice along with the payment amount. Integrating this level of intelligence to accounting workflows, businesses can significantly improve process speed, efficiency, and ultimately accuracy.
Automation solutions from UiPath can play an integral role in such applications. The UiPath AI Center combines automation with AI/ML capabilities. Enterprises may utilize custom and/or widely available AI models within the AI Center platform along with UiPath Studio for use with any relevant automation processes. AI Center also helps manage AI models with respect to the needs of ModelOps functionality, i.e. the focus on the governance and life cycle management of a range of operationalized AI models including versioning, updates, and retraining models.
To assist customers along the path toward building intelligent automation processes, UiPath has built upwards of 25 out-of-the-box ML models, in addition to many intelligent models provided by partners via the UiPath Marketplace. For example, the Document Understanding solution includes models for extracting data from invoices, purchase orders, receipts, and utility bills.
UiPath also has strategic partnerships with technology partners like Tableau, Alteryx, Qlik, AWS, and Snowflake to expand the data management potential.
In Summary
With a well-conceived data strategy and helpful automated data management tools, enterprises can better keep competitive and aligned with an evolving marketplace in order to expand opportunities. The reality is that automation tools don’t solve every data management business need, but the tasks they do address make a significant difference to the bottom line.
Speak Your Mind