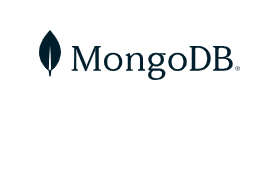
MongoDB, Inc. (NASDAQ: MDB) announced new capabilities, performance improvements, and a data-streaming integration for MongoDB Atlas Vector Search that make it even faster and easier for developers to build generative AI applications. Organizations of all sizes have rushed to adopt MongoDB Atlas Vector Search as part of a unified solution to process data for generative AI applications since being announced in preview in June of this year. MongoDB Atlas Vector Search has made it even easier for developers to aggregate and filter data, improving semantic information retrieval and reducing hallucinations in AI-powered applications. With new performance improvements for MongoDB Atlas Vector Search, the time it takes to build indexes is now significantly reduced by up to 85 percent to help accelerate application development. Additionally, MongoDB Atlas Vector Search is now integrated with fully managed data streams from Confluent Cloud to make it easier to use real-time data from a variety of sources to power AI applications. To learn more about MongoDB Atlas Vector Search, visit mongodb.com/products/platform/atlas-vector-search.
“It has been really exciting to see the overwhelmingly positive response to the preview version of MongoDB Atlas Vector Search as our customers eagerly move to incorporate generative AI technologies into their applications and transform their businesses—without the complexity and increased operational burden of ‘bolting on’ yet another software product to their technology stack. Customers are telling us that having the capabilities of a vector database directly integrated with their operational data store is a game changer for their developers,” said Sahir Azam, Chief Product Officer at MongoDB. “This customer response has inspired us to iterate quickly with new features and improvements to MongoDB Atlas Vector Search, helping to make building application experiences powered by generative AI even more frictionless and cost effective.”
Many organizations today are on a mission to invent new classes of applications that take advantage of generative AI to meet end-user expectations. However, the large language models (LLMs) that power these applications require up-to-date, proprietary data in the form of vectors—numerical representations of text, images, audio, video, and other types of data. Working with vector data is new for many organizations, and single-purpose vector databases have emerged as a short-term solution for storing and processing data for LLMs. However, adding a single-purpose database to their technology stack requires developers to spend valuable time and effort learning the intricacies of developing with and maintaining each point solution. For example, developers must synchronize data across data stores to ensure applications can respond in real time to end-user requests, which is difficult to implement and can significantly increase complexity, cost, and potential security risks. Many single-purpose databases also lack the flexibility to run as a managed service on any major cloud provider for high performance and resilience, severely limiting long-term infrastructure options. Because of these challenges, organizations from early-stage startups to established enterprises want the ability to store vectors alongside all of their data in a flexible, unified, multi-cloud developer data platform to quickly deploy applications and improve operational efficiency.
MongoDB Atlas Vector Search addresses these challenges by providing the capabilities needed to build generative AI applications on any major cloud provider for high availability and resilience with significantly less time and effort. MongoDB Atlas Vector Search provides the functionality of a vector database integrated as part of a unified developer data platform, allowing teams to store and process vector embeddings alongside virtually any type of data to more quickly and easily build generative AI applications. Dataworkz, Drivly, ExTrac, Inovaare Corporation, NWO.ai, One AI, VISO Trust, and many other organizations are already using MongoDB Atlas Vector Search in preview to build AI-powered applications for reducing public safety risk, improving healthcare compliance, surfacing intelligence from vast amounts of content in multiple languages, streamlining customer service, and improving corporate risk assessment. The updated capabilities for MongoDB Atlas Vector Search further accelerate generative AI application development:
- Increase the accuracy of information retrieval for generative AI applications: Whether personalized movie recommendations, quick responses from chatbots for customer service, or tailored options for food delivery, application end-users today expect accurate, up-to-date, and highly engaging experiences that save them time and effort. Generative AI is helping developers deliver these capabilities, but the LLMs powering applications can hallucinate (i.e., generate inaccurate information that is not useful) because they lack the necessary context to provide relevant information. By extending MongoDB Atlas’s unified query interface, developers can now create a dedicated data aggregation stage with MongoDB Atlas Vector Search to filter results from proprietary data and significantly improve the accuracy of information retrieval to help reduce LLM hallucinations in applications.
- Accelerate data indexing for generative AI applications: Generating vectors is the first step in preparing data for use with LLMs. Once vectors are created, an index must be built for the data to be efficiently queried for information retrieval—and when data changes or new data is available, the index must then be updated. The unified and flexible document data model powering MongoDB Atlas Vector Search allows operational data, metadata, and vector data to be seamlessly indexed in a fully managed environment to reduce complexity. With new performance improvements, the time it takes to build an index with MongoDB Atlas Vector Search is now reduced by up to 85 percent to help accelerate developing AI-powered applications.
- Use real-time data streams from a variety of sources for AI-powered applications: Businesses use Confluent Cloud’s fully managed, cloud-native data streaming platform to power highly engaging, responsive, real-time applications. As part of the Connect with Confluent partner program, developers can now use Confluent Cloud data streams within MongoDB Atlas Vector Search as an additional option to provide generative AI applications ground-truth data (i.e. accurate information that reflects current conditions) in real time from a variety of sources across their entire business. Configured with a fully managed connector for MongoDB Atlas, developers can make applications more responsive to changing conditions and provide end user results with greater accuracy.
Organizations Already Innovating with MongoDB Atlas Vector Search in Preview
Dataworkz enables enterprises to harness the power of LLMs on their own proprietary data by combining data, transformations, and AI into a single experience to produce high-quality, LLM-ready data. “Our goal is to accelerate the creation of AI applications with a product offering that unifies data, processing, and machine learning for business analysts and data engineers,” said Sachin Smotra, CEO and co-founder of Dataworkz. “Leveraging the power of MongoDB Atlas Vector Search has allowed us to enable semantic search and contextual information retrieval, vastly improving our customers’ experiences and providing more accurate results. We look forward to continuing using Atlas Vector Search to make retrieval-augmented generation with proprietary data easier for highly relevant results and driving business impact for our customers.”
Drivly provides commerce infrastructure for the automotive industry to programmatically buy and sell vehicles through simple APIs. “We are using AI embeddings and Atlas Vector Search to go beyond full-text search with semantic meaning, giving context and memory to generative AI car-buying assistants,” said Nathan Clevenger, Founder and CTO at Drivly. “We are very excited that MongoDB has added vector search capabilities to Atlas, which greatly simplifies our engineering efforts.”
ExTrac draws on thousands of data sources identified by domain experts, using AI-powered analytics to locate, track, and forecast both digital and physical risks to public safety in real-time. “Our domain experts find and curate relevant streams of data, and then we use AI to anonymize and make sense of it at scale. We take a base model and fine-tune it with our own labeled data to create domain-specific models capable of identifying and classifying threats in real-time.” said Matt King, CEO of ExTrac. “Atlas Vector Search is proving to be incredibly powerful across a range of tasks where we use the results of the search to augment our LLMs and reduce hallucinations. We can store vector embeddings right alongside the source data in a single system, enabling our developers to build new features way faster than if they had to bolt-on a standalone vector database—many of which limit the amount of data that can be returned if it has meta-data attached to it. Because the flexibility of MongoDB’s document data model allows us to land, index, and analyze data of any shape and structure—no matter how complex—we are now moving beyond text to vectorize images and videos from our archives dating back over a decade. Being able to query and analyze data in any modality will help us to better model trends, track evolving narratives, and predict risk for our customers.”
Inovaare Corporation is a leading provider of AI-powered compliance automation solutions for healthcare payers. “At Inovaare Corporation, we believe that healthcare compliance is not just about meeting regulations but transforming how healthcare payers excel in the entire compliance lifecycle. We needed a partner with the technological prowess and one who shares our vision to pioneer the future of healthcare compliance,” said Mohar Mishra, CTO and Co-Founder at Inovaare Corporation. “MongoDB’s robust data platform, known for its scalability and agility, perfectly aligns with Inovaare’s commitment to providing healthcare payers with a unified, secure, and AI-powered compliance operations platform. MongoDB’s innovative Atlas Vector Search powers the reporting capabilities of our products. It allows us to deliver context-aware compliance guidance and real-time data-driven insights.”
NWO.ai is a premier AI-driven Consumer Intelligence platform helping Fortune 500 brands bring new products to market. “In today’s rapidly evolving digital age, the power of accurate and timely information is paramount,” said Pulkit Jaiswal, Cofounder of NWO.ai. “At NWO.ai, our flagship offering, Worldwide Optimal Policy Response (WOPR), is at the forefront of intelligent diplomacy. WOPR harnesses the capabilities of AI to navigate the vast oceans of global narratives, offering real-time insights and tailored communication strategies. This not only empowers decision-makers but also provides a vital counterbalance against AI-engineered disinformation. We’re thrilled to integrate Atlas Vector Search into WOPR, enhancing our ability to instantly search and analyze embeddings for our dual-use case. It’s an exciting synergy, and we believe it’s a testament to the future of diplomacy in the digital age.”
One AI is a platform that offers AI Agents, Language Analytics, and APIs, enabling seamless integration of accurate, production-ready language capabilities into products and services. “Our hero product – OneAgent – facilitates trusted conversations through AI agents that operate strictly upon company-sourced content, secured with built-in fact-checking,” said Amit Ben, CEO and Founder of One AI. “With MongoDB Atlas, we’re able to take source customer documents, generate vector embeddings from them that we then index and store in MongoDB Atlas Vector Search. Then, when a customer has a question about their business and asks one of our AI agents, Atlas Vector Search will provide the chatbot with the most relevant data and supply customers with the most accurate answers. By enabling semantic search and information retrieval, we’re providing our customers with an improved and more efficient experience.”
VISO Trust puts reliable, comprehensive, actionable vendor security information directly in the hands of decision-makers who need to make informed risk assessments. “At VISO Trust, we leverage innovative technologies to continue our growth and expansion in AI and security. Atlas Vector Search, combined with the efficiency of AWS and Terraform integrations, has transformed our platform,” said Russell Sherman, Cofounder and CTO at VISO Trust. “With Atlas Vector Search, we now possess a battle-tested vector and metadata database, refined over a decade, effectively addressing our dense retrieval requirements. There’s no need to deploy a new database, as our vectors and artifact metadata can be seamlessly stored alongside each other.”
Sign up for the free insideAI News newsletter.
Join us on Twitter: https://twitter.com/InsideBigData1
Join us on LinkedIn: https://www.linkedin.com/company/insidebigdata/
Join us on Facebook: https://www.facebook.com/insideAI NewsNOW
Speak Your Mind