The symbiosis between data governance and cloud computing is apparent to any organization with significant cloud investments. The cloud has a plethora of resources that enhance data governance, which is critical for regulatory compliance, risk mitigation, and long term profitability of data assets.
Simultaneously, however, data governance hallmarks of metadata management, data cataloging, and data stewardship are requisite for maximizing the cloud’s utility by illustrating where data are and employing them for singular use cases like customer 360s, real-time product or service recommendations, and advanced analytics.
Although it’s difficult to say which of these capabilities is more advantageous to the enterprise, they clearly complement each other.
For data governance, the cloud’s chief value proposition is that “from a standpoint of connectivity, or data integration, or data quality, or cataloging, everything’s included so you have an all-in-one solution that’s possible because we’re in a microservices world where you can deploy efficiently and have things kind of seamlessly interoperable,” Informatica MDM General Manager Manouj Tahiliani revealed.
The cloud is also a significant contributor to dispersing resources across locations which, if left unchecked, could easily result in silos and ungoverned use. According to Profisee CTO Eric Melcher, the cloud creates “the much more distributed enterprise where you’ve got lots of applications: our HR applications, CRM applications, ERP applications, our customer experience platform. We’ve got applications across the line. And by the way, the data’s not in that server in the corner, it’s now all over the place. So, I need governance to understand where everything is.”
Data governance is essential for keeping cloud deployments orderly, while the cloud enriches the very means of effecting governance by allowing organizations to position resources wherever they’re most advantageous for modern computing—particularly when supported by governance staples of metadata management, data cataloguing, data modeling, and data stewardship.
Metadata Intelligence
Metadata is utilitarian for governing data in cloud settings. It’s particularly beneficial for assembling data across numerous sources to use in a single domain such as customer, product, supply chain information, and more. Tahiliani referred to the notion of “metadata intelligence” as foundational to implementing data quality and data integration. Coupling metadata intelligence with Master Data Management exploits the sundry of cloud sources by enabling organizations to govern how they “get data from different sources, curate it, and share it across the enterprise,” Tahiliani observed.
Metadata plays a couple different roles in this basic functionality. It’s the key to connecting data to various downstream systems through MDM’s logical model. Firms can also “use the power of metadata to help them discover elements that need to be mastered, rapidly provide integration between the system using that metadata knowledge and share that data, as well as use metadata to use machine learning capabilities around matching and data quality rules,” Tahiliani commented. These capabilities are instrumental for combining sources for customer insight, for example, in a well governed fashion.
Data Cataloging
Metadata also helps the data cataloging process Tahiliani described, which identifies what data are where in cloud settings. In fact, there are several cloud tools that reinforce this aspect of data governance by empowering organizations so “the first thing they do is catalog what they have,” Melcher noted. “Now that we’ve cataloged it, let’s start classifying and understanding what it is and figuring out what’s sensitive and who knows about the data here and there.” Once data are classified, it’s relatively straightforward to tag them and ascribe ownership to them, which provides the framework for suitably governing data across any variety of cloud sources.
Credible catalogs can point at sources to provide this functionality or allow sources to input their metadata for cataloging purposes. The latter is particularly effectual with MDM because it lets organizations push “the logical definitions of Master Data…and register assets to what the end user would actually expect,” Melcher mentioned. The benefit of this approach is data already adheres to MDM conventions of data quality, definitions, and other aspects of its logical model, which aids in creating catalogs and classifications that enhance enterprise use cases—while ensuring those use cases are well governed.
Data Modeling
MDM is also useful for unifying the different data models that abound throughout cloud sources so organizations can combine them for analytics, for example. Data modeling is a pivotal aspect of data governance that can be particularly time consuming (delaying time to value) with traditional methods. MDM, however, lets organizations create models for products or any other domain partly by using data discovery mechanisms. “As you’re creating the models and what attributes you need to have, the discovery aspect allows you to understand across the source systems for those different entities what’s the metadata that’s being held,” Tahiliani explained. “That provides information about how often it’s used and updated to really determine if that should be an attribute within your master data model.”
The most expressive data models unify terminology and include specific vocabularies to describe customers or supply chain needs, for instance. The metadata intelligence Tahiliani alluded to assists this facet of data modeling while allowing organizations to redress disparities in how entities are represented “when you know which source systems the metadata you’re pulling in is from, and what the vocabulary is across those source systems,” Tahiliani added.
Data Stewardship
All of the above dimensions of data governance—metadata intelligence, data cataloging, and data modeling—are invaluable to data stewards attempting to ensure governance standards are met across the enterprise in heterogeneous cloud, edge, and on-premise applications. The enhanced data modeling traits pertaining to definitions and attributes that are found in MDM (including information from data cataloging tools) are critical to data stewards validating data governance protocols.
For example, if a steward needs at-a-glance information about the data model for a customer entity, he’ll see “this is a description of a customer associated with this glossary term called business partner or whatever the case may be, and Bob in Sales is an expert on what customer is,” Melcher indicated. With this approach MDM “participates in governance by pushing…metadata into a catalog, but then, as governance occurs, the output of governance is then available for end users to consume back in [MDM],” Melcher concluded.
Master Data Governance
Data governance is indispensable for making good on the cloud’s premise of real-time access to a distributed data landscape with elastic scalability in a pay-per-use pricing model. Additionally, the cloud contains many tools—such as cloud native MDM solutions, data cataloging instruments, and others—that streamline governance capabilities to decrease data’s risk while boosting its enterprise value over the long term.
MDM sits between each of these constructs as a viable means of balancing the usefulness of one with the other. “You shouldn’t do governance in MDM,” Melcher cautioned. “You should do governance and MDM. You should be governing across more than just your Master Data Management platform.”
About the Author
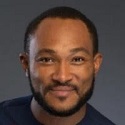
Jelani Harper is an editorial consultant servicing the information technology market. He specializes in data-driven applications focused on semantic technologies, data governance and analytics.
Sign up for the free insideAI News newsletter.
Join us on Twitter: @InsideBigData1 – https://twitter.com/InsideBigData1
Speak Your Mind