In an age in which data governance has become all but synonymous with data privacy and data protection, there are numerous aspects of data management that are regarded much differently than how they traditionally were.
Data modeling, for example, is frequently considered a dimension of data engineering or data science. From this perspective, data models are manipulated to integrate data between sources so organizations can load applications or analytics tools with a bevy of data across their various ecosystems.
However, conceptual data models—alternatively referred to as subject area models or ontologies—have always remained firmly entrenched within the realm of data governance. These models give data its meaning for achieving business objectives. Of all the forms of data modeling, conceptual models are likely the most important and the basis for many others (such as logical data models, entity-relationship models, etc.).
According to Aaron Colcord, Privacera Senior Director, Governance and Security, Center of Excellence, these conceptual models are “about how you, as a company, think about yourself.” Subsequently, these foundational data models include numerous aspects of organizations, from how they’re mapped out according to business units, to specific terminology, definitions, and taxonomies that influence what data mean to different roles.
These concepts are essential for successfully governing data so organizations can profit from long term data reusability while circumscribing risk.
Mapping The Business
Although there’s a broad spectrum of ontologies or conceptual data models (spanning from basic ones to highly intricate ones), at the very least they solidify the way an organization or business unit is structured. That information is integral for assigning ownership of data and forming the rudiments of what data means according to organizational definitions. When implementing the business concepts that are ascribed to this type of data model, data modelers must incorporate their companies’ multiple departments, roles, and responsibilities. “The thing is, you’ll always find that every single organization, the executives, they know how their business works, and that’s how their data is organized,” Colcord remarked.
Inputting that information in a subject area model clarifies these facts and becomes the means by which organizations define data for numerous downstream applications, including metadata management, data cataloging, and data quality. Moreover, for securing data to preserve data privacy and adhere to regulations, companies can rely on conceptual data models so that they can “now know where data is, go call it, and figure out what data is,” Colcord commented. That information becomes the basis for masking PII, for example, to conform to regulations for doing so.
Schema
The next progression in the utility ontologies provide for data governance pertains to the issue of schema—which is why data modeling has somewhat been annexed into the realm of data engineering. However, it’s important to realize that even in terms of schema, ontologies reflect domain information about business concepts and their meaning. The most exhaustive and utilitarian conceptual data models involve “an ontology or schema of all the important objects in a particular domain,” observed Franz CEO Jans Aasman. The amount of detail that such ontologies include is vast. These not only involve different concepts like product types and hierarchies of such products, but similar information for users, their roles, and even the relationships between these business objects and users.
The specificity for such ontologies inherently makes them unique. “For a bank, of course, it’s completely different than for a hospital or an airline inspector like the FAA,” Aasman noted. The data governance value of these highly detailed ontologies is multifold. They standardize the various constructs required for defining data so governance rules can be consistent and uniformly followed. They also provides concrete definitions for data in relation to those business objects, which helps solidify data’s meaning across use cases, business units, and sources. “Before you can share it, you have to know what data means,” Aasman said. With ontologies supplying that meaning, organizations can aggregate data between departments for customer 360 views, for example, to mine them for business value while conforming to governance mandates.
Terminology
The uniformity of meaning Aasman alluded to is characteristic of the most advanced ontologies, which typically include taxonomies. The relationship between the hierarchies of definitions that taxonomies deliver and the underlying conceptual data model isn’t always clear. It’s possible to utilize taxonomies without ontologies (and vice versa), although the most sophisticated ontologies invariably have some component for defining the words that describe business concepts. This glossary component that readily lends itself to conceptual data models is “where you have the vocabulary, the terminology,” expert.ai CTO Marco Varone revealed.
The importance of this element of conceptual data models is inestimable for governance purposes. By stipulating exactly what terms related to data mean to the business, all ambiguity is removed for implementing data quality and certain facets of metadata management. Data’s meaning in relation to business goals is further clarified by the support of synonyms that this linguistic aspect of conceptual data models provides. Varone characterized this utility as “more of a thesaurus… the language specific part.” Clearly defining the words and definitions that support business concepts reflected in data is integral for well-governed data sharing across domains and applications. It also assists with certain forms of Artificial Intelligence, including inference techniques, symbolic reasoning, and “structuring knowledge in the right way,” Varone indicated.
Data Governance 101
Despite current perceptions that suggest otherwise, data modeling is still a weighty part of data governance. Conceptual data models elucidate how organizations are structured, critical business concepts for which data are used, and what data specifically means in relation to those concepts.
That information impacts almost every dimension of data governance, from access control methods to lifecycle management and data cataloging. Creating these subject area models, perfecting them, and fortifying data governance with them is foundational to “trying to know what exactly your data is,” Colcord summarized—which is integral for forming the appropriate rules upon which data governance is predicated, and implementing them.
About the Author
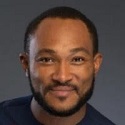
Jelani Harper is an editorial consultant servicing the information technology market. He specializes in data-driven applications focused on semantic technologies, data governance and analytics.
Sign up for the free insideAI News newsletter.
Join us on Twitter: @InsideBigData1 – https://twitter.com/InsideBigData1
Speak Your Mind