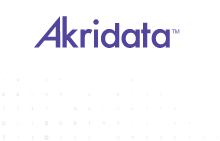
Akridata, an AI platform for visual data, announced the first availability of its platform that provides data science teams the tools to easily explore, search, analyze, and compare visual data to improve data sets and improve model training.
The volume of visual data has been increasing at unprecedented rates, with more and more cameras continually being deployed into use across the globe. However, the tools and procedures to process this massive amount of data have not kept pace with the growth. For many, ingesting and curating data is still a largely manual and time-intensive project.
Helge Jacobsen, Senior Deep Learning Engineer at Veo, says “Most academic datasets are far from the industry’s needs. In our case we have rather small objects in fairly big images. This is probably a common problem, but in academia it is kind of a niche.”
Akridata Data Explorer is the first platform designed to be uniquely focused on processing visual data in the ML lifecycle. Founded by a team of serial entrepreneurs with deep technical expertise in solving image processing challenges, Akridata quickly realized from working with computer vision data science teams that the bigger challenges lay in searching, clustering and selecting visual data to accelerate model accuracy.
“The demand for data scientists and the tools they need is only going to grow over time”, says Vijay Karamcheti, CEO and Co-Founder of Akridata. “There are 50 billion cameras worldwide, and the data they produce is increasing exponentially, so it’s imperative that data scientists have tools to comb through and analyze this data efficiently.”
The initial launch of Akridata Data Explorer arms data scientists with tools across the MLops lifecycle: Tools to visualize and drill down into large data sets as clusters based on embeddings (e.g. spotting different actions by vehicles, people, etc.), tools to search data (e.g. finding additional instances of objects found within a bounding box specified by the user), and identification of novelty data sets to reduce class imbalances, and reduce data labeling spend.
“Having worked with data scientists building models for computer vision applications, we understand the challenges they face,” said Sanjay Pichaiah, Vice President, Products & GTM of Akridata. “For AI models to be production grade, it is equally if not more important to choose the training datasets than the model parameters. There is a burning need for tools to assist data scientists in making intelligent and informed data selection. ”
Sign up for the free insideAI News newsletter.
Join us on Twitter: https://twitter.com/InsideBigData1
Join us on LinkedIn: https://www.linkedin.com/company/insidebigdata/
Join us on Facebook: https://www.facebook.com/insideAI NewsNOW
Speak Your Mind